

How travel demand modeling gets a boost from on-demand traffic data analytics
What is travel demand modeling.
Travel demand models use current travel behavior to predict future travel patterns from a sample of travel behavior data. As you might expect, models are critical tools for planners and engineers who use them to forecast the transportation needs of the communities they serve. They also help transportation professionals assess the pros and cons of different options for meeting those needs.
Is Travel Demand Modeling the same thing as transportation modeling?
Travel demand models are a subset of transportation models that specifically focus on — you guessed it — travel demand. They are commonly developed within a region or, more broadly, across an entire state. The regional “demand” for trip-making, informed by land use and economic conditions, and the “supply” of the transportation network determine when, where, and how trips are made within a region. These existing travel conditions can be extrapolated to various future scenarios to predict the impacts of transportation policy and infrastructure improvements.
But other types of transportation models also use mobility data to predict future travel patterns based on current inputs. The scale and complexity of these models can vary significantly, depending on the data inputs available and the level of detail required from the model forecasts. Outputs from these models can inform decisions ranging from traffic signal timing at an intersection to congestion management policies on a corridor to multi-billion dollar highway or transit infrastructure investments.
Transportation models are a critical tool utilized by planners, engineers, and decision-makers to understand the performance of a transportation network today so that the impacts of future changes in demographics, land use, policy decisions, and infrastructure improvements can be forecasted for different future scenarios. For example, a microsimulation model may use existing travel behaviors to predict how localized roadway changes (such as work zones or detours) will impact weaving, congestion, speeding, and other traffic patterns.
Other transportation modeling use cases include:
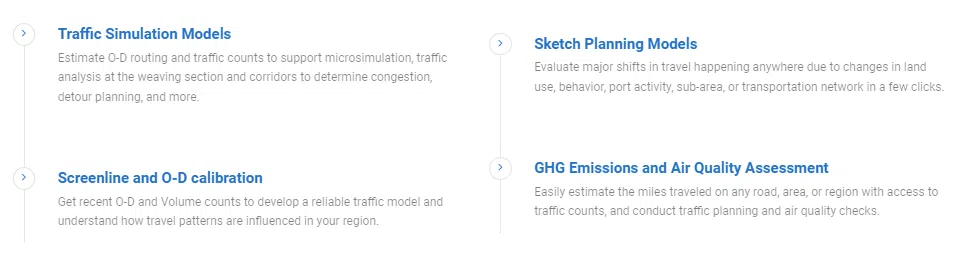
Getting the right data for travel demand models
Travel demand models — and transportation models in general— are only as accurate as their input data. Without an accurate understanding of real-world conditions today, planners and engineers cannot predict future demand.
Traditional data collection methods like physical sensors and surveys help gather information about existing conditions, but they’re also expensive and time-consuming to implement . This means these methods must be targeted at high-priority locations and may cover only a brief time period, resulting in low sample sizes that limit the precision of travel demand models.
On-demand transportation analytics — or “big data” analytics — address these limitations by using data collected from Connected Devices and the Internet of Things (IoT) to generate larger and more granular datasets that cover any road or time period. When used alongside traditional data collection methods, they help fill data gaps and enhance the efficacy of travel demand models .
In this guide, we’ll explore how travel demand models are built and calibrated and how transportation analytics help modelers get more actionable insights from these models.
WEBINAR: Build effective travel demand models with data that reflects changing mobility
Creating travel demand models
There are many ways to build a model, but no matter what, building accurate travel demand models requires detailed information about:
- Where groups of people go
- The ways their travel behavior changes during specific conditions
Traditionally, travel demand models use a four-step process to analyze regional transportation planning:
- Trip generation (the number of trips to be made)
- Trip distribution (where those trips go)
- Mode choice (how the trips will be divided among the available modes of travel)
- Trip assignment (predicting the route trips will take)
The results obtained through this four-step process vary widely, since they depend on the quality of the assumptions and data used, as well as the particular model’s sophistication. Micro models , or “microsimulations,” for small areas usually give users an estimate of highway volumes for individual roadways or intersections, where as “macro” and “meso” models look at entire counties, regions, and even state and multi-state geographies.
In the following example, traffic engineers with WSP USA used Big Data to build and calibrate a microsimulation to understand post-COVID travel demand on I-75 in Phoenix, looking specifically at weaving during peak hours and traffic interchange with a nearby highway.
No matter their size, the most sophisticated models incorporate and analyze highly granular data, such as commercial truck activity, HOV lane usage, tolling behavior, and more. Route choice between the same two locations, for example, can vary dramatically depending on time of day and other factors that influence drivers.
To illustrate, let’s say that our friend Sarah needs to drive from her home in Pittsburgh’s east end to the downtown business district. Most of the time, she finds Penn Avenue to be the fastest and most enjoyable route, but she also knows it is foolish to take that route during rush hour, a sports game, a major concert, or a visit from the President.
Ultimately, accurate travel demand models require quality input data. Outdated or incomplete data results in imprecise models, and your predictions could fail to account for the decisions that drivers like Sarah make almost subconsciously.
Using Big Data for origin-destination matrices
One of the most important data inputs for any model is an origin-destination (“O-D”) matrix—data that tells you where people are coming from (origin) and where they are going (destination) after visiting or passing through a specific location. In the past, planners utilized surveys, a set of “gravity” assumptions, and/or license plate studies to create O-D matrices, which are all costly and typically have small sample sizes.
Transportation experts can now use on-demand traffic data to quickly create precise, accurate and comprehensive O-D matrices , using algorithmic techniques that analyze trillions of data points and organize them by location and time stamp.
When transportation experts have analytics that incorporate this many data points, they can create traditional O-D matrices that represent a far greater percentage of the population and a longer timespan than could be captured through surveys or license plate studies. Planners can also capture short trips more accurately, like a quick run into the grocery store while on the commute home. Those types of short trips tend to get undercounted or completely overlooked in a typical travel survey.
In a nutshell, trips and series of activities are created by:
- Identifying the pings that occur when Connected Vehicles and IoT devices begin moving (the origins);
- Following the series of pings that occur as these vehicles or devices move (the route); and
- Identifying the final pings when vehicles or devices come to rest (the destinations).
It’s important, however, to recognize that these data points are messy at the outset. No single person could manage trillions of data points using Microsoft Excel! That means sophisticated processing techniques are critical to making these data sets manageable and effective for planning transportation projects.
Using on-demand analytics for these matrices helps deliver the fine resolution needed for accurate travel demand modeling. But that’s only the beginning. Trip data (such as the time stamps that identify home and work locations) can be combined with contextual data sets, such as parcel boundaries and aggregate demographic information from the Census. Now, your traditional O-D matrices can be analyzed in terms of trip purpose.
Inferring trip purpose
Trip purpose is also a key input for transportation modelers to consider. With Big Data, you can infer why groups of people travel from one location to another by analyzing aggregated behavior over a longer period. Big Data can help you estimate the portion of trips in a study that are:
- Home-Based Work: Travel between home and work in either direction
- Home-Based Other: Travel to and from the home, to anywhere other than work
- Non-Home Based: All travel not to or from home
For example, StreetLight InSight ® — that’s StreetLight Data’s interactive, online platform that aggregates and simplifies those trillions of data points — can provide the trip purposes for the purposes listed above for any O-D matrix in just a few minutes.
Planners can benefit from our Home-Work Trip Purpose Metrics because they no longer need to get this information from models or surveys, which are expensive to conduct and typically have low response rates. With comprehensive analytics derived from Big Data, planners can now gather spatially precise trip purpose information using real-world data. For example, when creating a traditional, four-step, Travel Demand Model, the Home-Work Trip Purpose Metric will be a huge asset.
Data requirements for dynamic traffic assignment
Another great modeling technique where one can use Big Data is Dynamic Traffic Assignment (DTA). DTA is better at modeling user response to issues such as peak spreading, freight analysis, and congestion at fine resolutions. But DTA is a meticulous modeling technique, so it requires detailed, rigorous data to be done right. The old approach requires collection from at least 6 different sources, and many of them are very cumbersome and expensive. It also requires a huge effort to integrate, calibrate, and check that data integration. The result is not only expensive and time consuming, but also has a lot of assumptions. It’s simply clunky and messy.
Working with Big Data, you can attack DTA with a direct, data-driven approach. Using fine-tuned origin-destination studies based on Big Data, modelers can uncover precise analytics such as how left-hand turns are affected by time of day and type of trip . Route choice can vary dramatically by time of day, so understanding the ways that behavior changes during specific conditions is critical to building accurate models.
This detailed modeling technique is particularly effective for modeling user response to issues such as peak spreading, freight analysis and congestion, in fine resolution. To be effective, however, DTA modeling requires detailed, rigorous data. When you use location data from mobile devices, you can get the information you need to create a DTA quickly and easily.
Stay ahead of fast-changing mobility trends with effective travel demand models
Big Data integration with other modeling tools
Beyond Big Data being a great source of information on its own, it can also be easily integrated into other modeling and simulation tools . CSV files of our Metrics can be downloaded directly from StreetLight InSight®, and then users can input that information into their preferred modeling tools . We have also developed easy-to-use transportation APIs , including the StreetLight InSight® API that can be used to integrate our analytics into many mapping and modeling tools.
Real-world case studies
Hundreds of transportation agencies are benefiting from using Big Data in travel demand models. It’s helping them understand travel behavior more comprehensively, precisely, and accurately. If your goals are to collect high-quality data cost-effectively, there is no better way to understand group travel patterns.
For example, when the City of Edmonton, Canada was unable to run its typically active annual traffic count program due to COVID, access to on-demand traffic data helped fill the gap and zero in on pandemic-driven shifts in travel behaviors, calibrating their model to these recent changes and allowing for more actionable insights to guide infrastructure projects and temporary traffic measures.

Similarly, in the video below, you’ll see how an AEC firm used on-demand analytics to update the Butte County Association of Governments (BCAG) travel demand model, using O-D analyses and Zone Activity analyses to understand interregional travel, paying special attention to key tourist and special event destinations.
Beyond travel demand modeling
As we’ve discussed, Big Data is an essential tool for understanding the ways behavior changes during specific conditions, and building accurate travel demand models. But there are a number of other applications that are transforming transportation.
Here are seven other ways to harness the power of Big Data in your planning and projects:
- Build climate-forward infrastructure and decarbonize transportation
- Diagnose and mitigate traffic congestion
- Improve mobility on high-priority corridors
- Win federal grant funding for infrastructure projects
- Eliminate traffic fatalities and make streets safer
- Build equity-first transportation systems
Claim Your Free Consultation
Schedule your no-strings consultation to explore how digital analytics can help future-proof mobility in your region.
General Inquiries:
Contact streetlight, partnership inquiries:, [email protected], join the conversation, subscribe to insights & research.
Please provide your contact information so we can send the latest insights and research from StreetLight to your inbox; you can unsubscribe at any time.
- About StreetLight
- Website Privacy Notice
GETTING STARTED
- Why StreetLight
- Read Customer Stories
- Schedule a Demo
- StreetLight InSight® LOGIN
- StreetLight Training Portal
- Help Center
- Community Forum
- Customer Care
Copyright © 2011 - 2023, StreetLight Data, Inc. 4 Embarcadero Center Suite 3800 San Francisco, CA 94105. All rights reserved. Website Privacy Notice | Open Source
- How It Works
- Cost Savings
- 3rd Party Validations
- Testimonials
- AADT Traffic Counts
- Origin-Destination
- Turning Movement Counts
- Advanced Traffic Counts
- More Metrics…
- Personal Vehicles
- Bicycle & Pedestrian
- Bus & Rail
- Commercial Truck
- Delegate the work to us
- Get Data Driven Insights
- Developer Resources
- Transportation Planning
- Traffic Engineering & Operations
- Electric Vehicles (EVs)
- New Mobility Deployment
- Ride Hailing & Delivery
- AVs & UAM
- Financial Services
- Retail & Real Estate
- Climate Solutions
Congestion Management
Corridor studies, transportation modeling, roadway volumes, intersection studies.
- Grant Solutions
Safety Studies
- Social Equity
- Data Privacy
- Partnerships
- University Research
- Case Studies
- Research Reports
- White Papers
- Support & Training
Save time without relying on outdated equipment and get instant granular counts for any intersection at any time on any day.
Modes included:
Metrics included:
Total, Inbound, and Outbound Intersection Traffic Volumes, AM/PM Peak Hours, Peak Hour Factor
All plans include:
24/7 access to StreetLight InSight® & 100% coverage of Traffic Analysis Zones
Business Consulting & Services
Enhance value to clients with location intelligence and mobility insights informed by granular, validated transportation data..
Real Estate Site Selection, Portfolio Analysis, Due Diligence
Key metrics:
Vehicle, Truck, Pedestrian, Bicycle, and Transit Travel Patterns
Private Transportation
Model demand for your mobility service – airline, evtol, train, micro-mobility, and more – to plan routes and hubs that capture your target customers..
Travel Demand Modeling, Hub Site Selection, Route Planning
Origin-Destination, Travel Patterns for many modes, Demographics and Trip Purpose
Electric Vehicles, Energy & Utilities
Future-proof your electric vehicle infrastructure and investments with data-driven insights..
Electric Vehicle Infrastructure Site Selection, Grid Planning
Traffic Volumes, Trip Length, Travel Time, Dwell Time, EV Travel Patterns, Demographics
Technology, Data & Analytics
Uplevel your location intelligence solutions by partnering with streetlight to incorporate validated mobility metrics into your platform, models, or services., hospitality &tourism, analyze seasonal visitation to your region or property to forecast future tourism and manage traffic impacts..
Visitation, Cut-Through Analysis, Congestion Management
Traffic Counts, Origin-Destination, Top Routes for Travelers, Vehicle Speed
Position your projects for success with turnkey access to defensible and validated traffic counts for all roadways.
Average Annual Daily Traffic (AADT) and more
Active Transportation
Get the reliable data you need to justify your active transportation decisions and build infrastructure that reflects the reality of today..
Volume/Traffic Counts, Origin-Destination, Select Link, Speed, Demographics, Travel Time, and more
Develop, calibrate, and validate your travel demand models’ granular analytics to ensure that your models properly forecast travel patterns.
Volume/Traffic Counts, Origin-Destination, Select Link, Demographics, Trip Purpose, and more
Truck Studies
Measure up-to-date truck activity across all road types to identify congestion bottlenecks and evaluate the economic impact..
Volume, Travel Time, Trip Length, Origin-Destination, Routing, Vehicle Classification, and more
Diagnose traffic patterns for all hours of the day to develop data-informed strategies that mitigate traffic congestion.
Volume, Vehicle Miles Traveled (VMT), Vehicle Hours of Delay (VHD), Planning Index, Buffer Time Index, Travel Time, Speed, and more
Traffic Calming & Management
Easily fill data gaps, address residents’ top concerns, and justify infrastructure initiatives with insights into local and residential roads..
Volume, VMT/VKT, Speed, VHD, Congestion, Travel Time, Trip Length, Speed and Travel Time Percentiles, Demographics, and more
Visitation Studies
Effectively manage operations by accessing on-demand visitation metrics that show when, how, and from where people travel to key sites..
Volume, Trip Origins, Travel Time, Trip Length, Trip Speed, Traveler Demographics, Home and Work locations
Passenger Rail Studies
Evaluate network ridership for any time of day or day of week to help prioritize projects and advocate for bus and rail improvements..
Ridership, Travel Time, Origin-Destination Pairs, Rider Demographics
Retail & Restaurant
Choose the best locations for your business with mobility analytics that pinpoint customer demand to drive sales.
Key use cases:
Site Selection, Trade Area Analysis, Electric Vehicle Charger Site Selection
Traffic Counts, Trip Purpose, Demographics, Top Routes
Automotive Aftermarket & Fueling
Understand demand for your automotive product or service with validated nationwide vehicle travel patterns..
Supply Chain and Inventory Management, Market Research & Demand Forecasting
VMT / VKT, Personal and Commercial Vehicle Roadway Volumes, Fleet Characteristics
Leverage powerful multimodal insights to zero in on opportunities to keep your community safe and equitable.
Volume, VMT/VKT, Speed, VHD, Congestion, Travel Time, Demographics, Trip Purpose, Speed and Travel Time Percentiles, and more
Run multiple analyses for the same corridor in a few clicks to ensure that you are solving problems with a macro- and micro-level lens.
Volume, VMT/VKT, Speed, VHD, Congestion, Travel Time, and more
Analyze traffic patterns on popular routes near toll roads to evaluate the impact of toll changes on congestion, accessibility, and safety.
Origin-Destination, Top Routes between locations, Trip Speed, Travel Time, Trip Length, Traveler Demographics
Table removed due to copyright \ restrictions.
Table removed due to copyright restrictions.
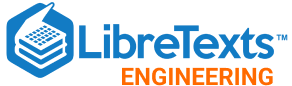
- school Campus Bookshelves
- menu_book Bookshelves
- perm_media Learning Objects
- login Login
- how_to_reg Request Instructor Account
- hub Instructor Commons
- Download Page (PDF)
- Download Full Book (PDF)
- Periodic Table
- Physics Constants
- Scientific Calculator
- Reference & Cite
- Tools expand_more
- Readability
selected template will load here
This action is not available.
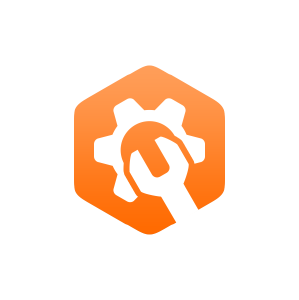
3.4: Trip Generation
- Last updated
- Save as PDF
- Page ID 47326
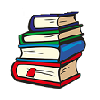
- David Levinson et al.
- Associate Professor (Engineering) via Wikipedia
Trip Generation is the first step in the conventional four-step transportation forecasting process (followed by Destination Choice, Mode Choice, and Route Choice), widely used for forecasting travel demands. It predicts the number of trips originating in or destined for a particular traffic analysis zone.
Every trip has two ends, and we need to know where both of them are. The first part is determining how many trips originate in a zone and the second part is how many trips are destined for a zone. Because land use can be divided into two broad category (residential and non-residential) we have models that are household based and non-household based (e.g. a function of number of jobs or retail activity).
For the residential side of things, trip generation is thought of as a function of the social and economic attributes of households (households and housing units are very similar measures, but sometimes housing units have no households, and sometimes they contain multiple households, clearly housing units are easier to measure, and those are often used instead for models, it is important to be clear which assumption you are using).
At the level of the traffic analysis zone, the language is that of land uses "producing" or attracting trips, where by assumption trips are "produced" by households and "attracted" to non-households. Production and attractions differ from origins and destinations. Trips are produced by households even when they are returning home (that is, when the household is a destination). Again it is important to be clear what assumptions you are using.
People engage in activities, these activities are the "purpose" of the trip. Major activities are home, work, shop, school, eating out, socializing, recreating, and serving passengers (picking up and dropping off). There are numerous other activities that people engage on a less than daily or even weekly basis, such as going to the doctor, banking, etc. Often less frequent categories are dropped and lumped into the catchall "Other".
Every trip has two ends, an origin and a destination. Trips are categorized by purposes , the activity undertaken at a destination location.
Observed trip making from the Twin Cities (2000-2001) Travel Behavior Inventory by Gender
Some observations:
- Men and women behave differently on average, splitting responsibilities within households, and engaging in different activities,
- Most trips are not work trips, though work trips are important because of their peaked nature (and because they tend to be longer in both distance and travel time),
- The vast majority of trips are not people going to (or from) work.
People engage in activities in sequence, and may chain their trips. In the Figure below, the trip-maker is traveling from home to work to shop to eating out and then returning home.

Specifying Models
How do we predict how many trips will be generated by a zone? The number of trips originating from or destined to a purpose in a zone are described by trip rates (a cross-classification by age or demographics is often used) or equations. First, we need to identify what we think the relevant variables are.
The total number of trips leaving or returning to homes in a zone may be described as a function of:
\[T_h = f(housing \text{ }units, household \text{ }size, age, income, accessibility, vehicle \text{ }ownership)\]
Home-End Trips are sometimes functions of:
- Housing Units
- Household Size
- Accessibility
- Vehicle Ownership
- Other Home-Based Elements
At the work-end of work trips, the number of trips generated might be a function as below:
\[T_w=f(jobs(area \text{ }of \text{ } space \text{ } by \text{ } type, occupancy \text{ } rate\]
Work-End Trips are sometimes functions of:
- Area of Workspace
- Occupancy Rate
- Other Job-Related Elements
Similarly shopping trips depend on a number of factors:
\[T_s = f(number \text{ }of \text{ }retail \text{ }workers, type \text{ }of \text{ }retail, area, location, competition)\]
Shop-End Trips are sometimes functions of:
- Number of Retail Workers
- Type of Retail Available
- Area of Retail Available
- Competition
- Other Retail-Related Elements
A forecasting activity conducted by planners or economists, such as one based on the concept of economic base analysis, provides aggregate measures of population and activity growth. Land use forecasting distributes forecast changes in activities across traffic zones.
Estimating Models
Which is more accurate: the data or the average? The problem with averages (or aggregates) is that every individual’s trip-making pattern is different.
To estimate trip generation at the home end, a cross-classification model can be used. This is basically constructing a table where the rows and columns have different attributes, and each cell in the table shows a predicted number of trips, this is generally derived directly from data.
In the example cross-classification model: The dependent variable is trips per person. The independent variables are dwelling type (single or multiple family), household size (1, 2, 3, 4, or 5+ persons per household), and person age.
The figure below shows a typical example of how trips vary by age in both single-family and multi-family residence types.

The figure below shows a moving average.

Non-home-end
The trip generation rates for both “work” and “other” trip ends can be developed using Ordinary Least Squares (OLS) regression (a statistical technique for fitting curves to minimize the sum of squared errors (the difference between predicted and actual value) relating trips to employment by type and population characteristics.
The variables used in estimating trip rates for the work-end are Employment in Offices (\(E_{off}\)), Retail (\(E_{ret}\)), and Other (\(E_{oth}\))
A typical form of the equation can be expressed as:
\[T_{D,k}=a_1E_{off,k}+a_2E_{oth,k}+a_3E_{ret,k}\]
- \(T_{D,k}\) - Person trips attracted per worker in Zone k
- \(E_{off,i}\) - office employment in the ith zone
- \(E_{oth,i}\) - other employment in the ith zone
- \(E_{ret,i}\)- retail employment in the ith zone
- \(a_1,a_2,a_3\) - model coefficients
Normalization
For each trip purpose (e.g. home to work trips), the number of trips originating at home must equal the number of trips destined for work. Two distinct models may give two results. There are several techniques for dealing with this problem. One can either assume one model is correct and adjust the other, or split the difference.
It is necessary to ensure that the total number of trip origins equals the total number of trip destinations, since each trip interchange by definition must have two trip ends.
The rates developed for the home end are assumed to be most accurate,
The basic equation for normalization:
\[T'_{D,j}=T_{D,j} \dfrac{ \displaystyle \sum{i=1}^I T_{O,i}}{\displaystyle \sum{j=1}^J T_{TD,j}}\]
Sample Problems
Planners have estimated the following models for the AM Peak Hour
\(T_{O,i}=1.5*H_i\)
\(T_{D,j}=(1.5*E_{off,j})+(1*E_{oth,j})+(0.5*E_{ret,j})\)
\(T_{O,i}\) = Person Trips Originating in Zone \(i\)
\(T_{D,j}\) = Person Trips Destined for Zone \(j\)
\(H_i\) = Number of Households in Zone \(i\)
You are also given the following data
A. What are the number of person trips originating in and destined for each city?
B. Normalize the number of person trips so that the number of person trip origins = the number of person trip destinations. Assume the model for person trip origins is more accurate.
Solution to Trip Generation Problem Part A
\[T'_{D,j}=T_{D,j} \dfrac{ \displaystyle \sum{i=1}^I T_{O,i}}{\displaystyle \sum{j=1}^J T_{TD,j}}=>T_{D,j} \dfrac{37500}{36750}=T_{D,j}*1.0204\]
Solution to Trip Generation Problem Part B
Modelers have estimated that the number of trips leaving Rivertown (\(T_O\)) is a function of the number of households (H) and the number of jobs (J), and the number of trips arriving in Marcytown (\(T_D\)) is also a function of the number of households and number of jobs.
\(T_O=1H+0.1J;R^2=0.9\)
\(T_D=0.1H+1J;R^2=0.5\)
Assuming all trips originate in Rivertown and are destined for Marcytown and:
Rivertown: 30000 H, 5000 J
Marcytown: 6000 H, 29000 J
Determine the number of trips originating in Rivertown and the number destined for Marcytown according to the model.
Which number of origins or destinations is more accurate? Why?
T_Rivertown =T_O ; T_O= 1(30000) + 0.1(5000) = 30500 trips
T_(MarcyTown)=T_D ; T_D= 0.1(6000) + 1(29000) = 29600 trips
Origins(T_{Rivertown}) because of the goodness of fit measure of the Statistical model (R^2=0.9).
Modelers have estimated that in the AM peak hour, the number of trip origins (T_O) is a function of the number of households (H) and the number of jobs (J), and the number of trip destinations (T_D) is also a function of the number of households and number of jobs.
\(T_O=1.0H+0.1J;R^2=0.9\)
Suburbia: 30000 H, 5000 J
Urbia: 6000 H, 29000 J
1) Determine the number of trips originating in and destined for Suburbia and for Urbia according to the model.
2) Does this result make sense? Normalize the result to improve its accuracy and sensibility?
- \(T_{O,i}\) - Person trips originating in Zone i
- \(T_{D,j}\) - Person Trips destined for Zone j
- \(T_{O,i'}\) - Normalized Person trips originating in Zone i
- \(T_{D,j'}\) - Normalized Person Trips destined for Zone j
- \(T_h\) - Person trips generated at home end (typically morning origins, afternoon destinations)
- \(T_w\) - Person trips generated at work end (typically afternoon origins, morning destinations)
- \(T_s\) - Person trips generated at shop end
- \(H_i\) - Number of Households in Zone i
- \(E_{off,k}\) - office employment in Zone k
- \(E_{ret,k}\) - retail employment in Zone k
- \(E_{oth,k}\) - other employment in Zone k
- \(B_n\) - model coefficients
Abbreviations
- H2W - Home to work
- W2H - Work to home
- W2O - Work to other
- O2W - Other to work
- H2O - Home to other
- O2H - Other to home
- O2O - Other to other
- HBO - Home based other (includes H2O, O2H)
- HBW - Home based work (H2W, W2H)
- NHB - Non-home based (O2W, W2O, O2O)
External Exercises
Use the ADAM software at the STREET website and try Assignment #1 to learn how changes in analysis zone characteristics generate additional trips on the network.
Additional Problems
- the start and end time (to the nearest minute)
- start and end location of each trip,
- primary mode you took (drive alone, car driver with passenger, car passenger, bus, LRT, walk, bike, motorcycle, taxi, Zipcar, other). (use the codes provided)
- purpose (to work, return home, work related business, shopping, family/personal business, school, church, medical/dental, vacation, visit friends or relatives, other social recreational, other) (use the codes provided)
- if you traveled with anyone else, and if so whether they lived in your household or not.
Bonus: Email your professor at the end of everyday with a detailed log of your travel diary. (+5 points on the first exam)
- Are number of destinations always less than origins?
- Pose 5 hypotheses about factors that affect work, non-work trips? How do these factors affect accuracy, and thus normalization?
- What is the acceptable level of error?
- Describe one variable used in trip generation and how it affects the model.
- What is the basic equation for normalization?
- Which of these models (home-end, work-end) are assumed to be more accurate? Why is it important to normalize trip generation models
- What are the different trip purposes/types trip generation?
- Why is it difficult to know who is traveling when?
- What share of trips during peak afternoon peak periods are work to home (>50%, <50%?), why?
- What does ORIO abbreviate?
- What types of employees (ORIO) are more likely to travel from work to home in the evening peak
- What does the trip rate tell us about various parts of the population?
- What does the “T-statistic” value tell us about the trip rate estimation?
- Why might afternoon work to home trips be more or less than morning home to work trips? Why might the percent of trips be different?
- Define frequency.
- Why do individuals > 65 years of age make fewer work to home trips?
- Solve the following problem. You have the following trip generation model:
\[Trips=B_1Off+B_2Ind+B_3Ret\]
And you are given the following coefficients derived from a regression model.
If there are 600 office employees, 300 industrial employees, and 200 retail employees, how many trips are going from work to home?
Open Access is an initiative that aims to make scientific research freely available to all. To date our community has made over 100 million downloads. It’s based on principles of collaboration, unobstructed discovery, and, most importantly, scientific progression. As PhD students, we found it difficult to access the research we needed, so we decided to create a new Open Access publisher that levels the playing field for scientists across the world. How? By making research easy to access, and puts the academic needs of the researchers before the business interests of publishers.
We are a community of more than 103,000 authors and editors from 3,291 institutions spanning 160 countries, including Nobel Prize winners and some of the world’s most-cited researchers. Publishing on IntechOpen allows authors to earn citations and find new collaborators, meaning more people see your work not only from your own field of study, but from other related fields too.
Brief introduction to this section that descibes Open Access especially from an IntechOpen perspective
Want to get in touch? Contact our London head office or media team here
Our team is growing all the time, so we’re always on the lookout for smart people who want to help us reshape the world of scientific publishing.
Home > Books > Models and Technologies for Smart, Sustainable and Safe Transportation Systems
Recent Progress in Activity-Based Travel Demand Modeling: Rising Data and Applicability
Submitted: 18 May 2020 Reviewed: 31 August 2020 Published: 17 September 2020
DOI: 10.5772/intechopen.93827
Cite this chapter
There are two ways to cite this chapter:
From the Edited Volume
Models and Technologies for Smart, Sustainable and Safe Transportation Systems
Edited by Stefano de Luca, Roberta Di Pace and Chiara Fiori
To purchase hard copies of this book, please contact the representative in India: CBS Publishers & Distributors Pvt. Ltd. www.cbspd.com | [email protected]
Chapter metrics overview
1,073 Chapter Downloads
Impact of this chapter
Total Chapter Downloads on intechopen.com

Total Chapter Views on intechopen.com
Over 30 years have passed since activity-based travel demand models (ABMs) emerged to overcome the limitations of the preceding models which have dominated the field for over 50 years. Activity-based models are valuable tools for transportation planning and analysis, detailing the tour and mode-restricted nature of the household and individual travel choices. Nevertheless, no single approach has emerged as a dominant method, and research continues to improve ABM features to make them more accurate, robust, and practical. This paper describes the state of art and practice, including the ongoing ABM research covering both demand and supply considerations. Despite the substantial developments, ABM’s abilities in reflecting behavioral realism are still limited. Possible solutions to address this issue include increasing the inaccuracy of the primary data, improved integrity of ABMs across days of the week, and tackling the uncertainty via integrating demand and supply. Opportunities exist to test, the feasibility of spatial transferability of ABMs to new geographical contexts along with expanding the applicability of ABMs in transportation policy-making.
- activity-based models
- travel demand forecasting
- transportation planning
- transferability of transport demand models
Author Information
Atousa tajaddini *.
- Institute of Transport Studies, Monash University, Australia
Geoffrey Rose
Kara m. kockelman.
- The University of Texas, The United States of America
Hai L. Vu *
*Address all correspondence to: [email protected] and [email protected]
1. Introduction
In recent years, behaviorally oriented activity-based travel demand models (ABMs) have received much attention, and the significance of these models in the analysis of travel demand is well documented in the literature [ 1 , 2 ]. These models are found to be consistent and realistic in several fundamental aspects. They possess some significant advantages over the simple aggregated trip-based travel demand models [ 3 ]. To achieve this, ABMs consider the linkage among activities and travel for an individual as well as different people within the same household and place more attention to the constraints of time and space. In other words, these models are capable of integrating both the activity, time, and spatial dimensions. The comprehensive advantages of activity-based models in comparison to the trip-based models have been discussed in previous papers [ 4 , 5 , 6 , 7 , 8 ]. Activity-based models are suitable for a wider variety of transportation policies involving individual decisions such as congestion pricing and ridesharing. More especially, enabling the relationship between activity and behavioral pattern of trip making is one of the main reasons for the shift from the aggregate-level in trip based models to disaggregate-level provided by ABMs [ 9 ].
Activity-based travel demand models (ABMs) can be classified into two main groups: Utility maximization-based econometric models and rule-based computational process models (CPM). Utility maximization-based econometric models apply different econometric structures such as logit, probit, hazard-based, and ordered response models. While the logit models rely on different assumptions about the distribution of the error terms in the utility functions, hazard-based models use the duration of activity based on end-of-duration occurrence to generate activity schedules [ 10 ]. Rule-based computational process models apply different sets of condition-action rules and focus on the implementation of daily travel and ordering activities to mimic individuals’ behavior when constructing schedules. In addition to the aforementioned models, other approaches can be employed either in combination with these models or separately to develop activity-based models. Examples include agent-based and time-space prism approaches. While an agent-based approach allows agents to learn, modify, and improve their interactions with other agents as well as their dynamic environment, time-space prisms are utilized to capture spatial and temporal constraints under which individuals construct the patterns of their activities and trips. Figure 1 exhibits critical elements of ABM such as activity generation, activity scheduling, and mobility choices. It also provides a comparison among the notable existing travel demand models regarding their different elements. The development of activity-based travel demand models has been reviewed comprehensively in previous studies [ 10 , 11 ]. Table 1 provides a summary of the literature on the evolution of these models over time by introducing the notable existing developed models and highlighting their limitations.

Components of activity-based travel demand models.
ABM evolution over time.
Despite the existence of many models as listed in Table 1 , ABM’s abilities in reflecting behavioral realism are still limited [ 40 ]. The capability of ABM models in predicting individual travel movements can be evaluated from two perspectives of input (data) and output (applicability). Activity schedules are an essential input into the ABM model. From an input point of view, the necessity of deriving activity schedules from dynamic resources together with their challenges will be reviewed. From the applicability perspective, the application of ABM output in integration with dynamic traffic assignment (DTA) models, transferring to a new geographical context, and why and how it is applied in transport planning management will also be discussed. To this end, the first part of this paper will review the new real-time data resources revealing the pattern and traces of traveler’s mobility at a large scale and over an extended period of time. The big data enables new ABM models to reflect mobility behavior on an unprecedented level of detail while collecting data over a longer period (e.g., more than one typical day) would improve the behavioral realism in trip making [ 41 ]. The second part of this paper looks into the applicability of ABM models. This part includes (i) gap investigation in enriching ABMs by integrating time-dependent OD matrices produced by ABMs with dynamic traffic assignment; (ii) investigation of ABMs’ applicability in transferring from one region to another; and (iii) enriching the capability of ABMs by moving beyond the transportation domain to other such as environment and management strategies.
The remainder of the paper is organized as follows. Section 2 introduces new data sources such as mobile phone call data records, transit smart cards, and GPS data where the influence of new data sources on the planning of activities, formation, and analysis of the travel behavior of individuals will be investigated. This section also introduces activity-based travel demand models, which generates activity-travel schedules longer than a typical day. Section 3 describes the existing experiences in transferring utility-based and CPM activity-based travel demand models from one geographical area to another. This section also reviews the integration of ABM models with dynamic traffic assignment and other models such as air quality models. The possibility of using activity-based models in travel demand management strategies with a focus on car-sharing and telecommuting are considered as examples. The last section concludes the paper and identifies remaining challenges in the area of activity-based travel demand modeling.
2. ABMs and the emerging of big data
This section provides an overview of the role of big data in replacing the traditional data sources, and the changes in activity-based travel demand models given these newly available data.
2.1 Improvements in activity-based travel demand modeling
It is more than half a century that transportation planners try to understand how individuals schedule their activities and travel to improve urban mobility and accessibility. The evolution of travel demand modeling from trip-based to activity-based highlighted the need for high-resolution databases including sociodemographic and economic attributes of individuals and travel characteristics. Today, with the rapid advancements in computation, technology, and applications, the intelligent transportation systems (ITS) have revolutionized the analysis of travel behavior by having more accurate data, removing human errors, and making use of the vast amount of available data [ 42 ]. Tools such as GPS devices, smartphones, smart card data, and social networking sites all have the potential to track the movements and activities of individuals by recording and retaining the relevant data continuously over time. Most of the traditional travel survey data are rich in detail. However, it can result in biased travel demand models because of incomplete self-reports and inaccurate scheduling patterns. Therefore, in this section, the common tools used in collecting big data are introduced and the progress made in the area of extracting big data sources is discussed.
2.1.1 Cell phone data
A call detail record (CDR) is a data record produced by a telephone exchange and consists of spatiotemporal information on the recent system usage [ 40 ], which can track people’s movements. This CDR data can be processed and applied in activity-based travel demand modelings to better understand human mobility and obtain more accurate origin-destination (OD) tables [ 43 ]. The first attempt using CDR data was a study of Caceres et al. [ 44 ], who applied mobile phone data to generate OD matrices. Their concept was then formalized by Wang et al. [ 45 ] to obtain transient OD matrices by counting trips for each pair of the following calls from two different telephone (cell) towers at the same hour. Afterward, using the shortest path algorithm, OD trips are assigned to the road network. In the area of urban activity recognition, Farrahi et al. [ 46 ] applied two probabilistic methods (i.e., Latent Dirichlet Allocation (LDA) and Author Topic Models, ATM) to cluster CDR trajectories according to their temporal aspects to discover the home and work activities. Considering the spatial aspect of CDR data, Phithakkitnukoon et al. [ 47 ] applied auxiliary land use data and geographical information database to find possible activities around a certain cell tower. And considering both the temporal-spatial aspect of CDR, Widhalm et al. [ 48 ] used an undirected relational Markov network to infer urban activities. They extracted activity patterns for Boston and Vienna by analyzing cell phone data (activity time, duration, and land use). Their results show that trip sequence patterns and activity scheduling observed from datasets were compatible with city surveys as well as the stability of generated activity clusters across time. In a more recent study, [ 49 ] an unsupervised generative state-space model is applied to extract user activity patterns from CDR data. Furthermore, it has been shown that the method of CDR sampling is as significant as survey sampling. For example, in one study [ 50 ], CDR and survey data is used during a period of six months to investigate the daily mobility for Paris and Chicago. The result shows that 90% of travel patterns observed in both surveys are compatible with phone data. In another similar study [ 51 ], a probabilistic induction was proposed using motifs (daily mobility network), time of day activity sequence, and land use classification to produce activity types. CDR data of Singapore was used by Jiang et al. [ 52 ] to produce activity-based human mobility patterns.
In the context of activity-based transport modeling, Zilske et al. [ 53 ] replaced travel diaries with CDRs as input data for agent-based traffic simulation. They first generated the synthetic CDR data, then the MATSim simulation software was used to identify every observed person as an agent to convert call information into activity. They fused the CDR data set with traffic counts in their next paper [ 54 ], to reduce the Spatio-temporal uncertainty.
In summary, the findings reported from different studies indicated the major implications of mobile phone records on the estimation of travel demand variables including travel time, mode and route choice as well as OD demand and traffic flow estimation; however; in practice, the information generated from CDR data are yet to be used widely in simulation models. This is mainly because of the conflict between either level of resolution or format and completeness of model and data [ 55 ].
2.1.2 Smart card data
Smart card systems with on- and off-boarding information gained much popularity in large public transport systems all over the world, and have become a new source of data to understand and identify the Spatio-temporal travel patterns of the individual passengers. The smart card data are investigated in various studies such as activity identification, scheduling, agent-based transport models, and simulation [ 56 ]. Besides, in other studies [ 57 , 58 , 59 ] smart card data was used as an analysis tool in investigating the passenger movements, city structure, and city area functions. Similarly, in the recent study [ 60 ], a visual analysis system called PeopleVis was introduced to examine the smart card data (SCD) and predict the travel behavior of each passenger. They used one-week SCD in the city of Beijing and found a group of “familiar strangers” who did not know each other but had lots of similarities in their trip choices. Zhao et al. [ 61 ] also investigated the group behavior of metro passengers in Zhechen by applying the data mining procedure. After extracting patterns from smart card transaction data, statistical-based and clustering-based methods were applied to detect the passengers’ travel patterns. The results show that a temporally regular passenger is very probable to be a spatially regular passenger. The disaggregated nature of smart card data represents suitable input to multi-agent simulation frameworks. For example, the smart card data is used to generate activity plans and implement an agent-based microsimulation of public transport in two cities of Amsterdam and Rotterdam [ 62 ]. An agent-based transport simulation is developed for Singapore’s public transport using MATSim environment [ 63 ]. Unlike Bouman’s study, they considered the interaction of public transport with private vehicles. The study of Fourie et al. [ 64 ] was another research work to present the possibility of integrating big data algorithms with agent-based transport models. Zhu [ 65 ] compared one-week transaction data of smart cards in Shanghai and Singapore. They found feasibility in generating continuous transit use profiles for different types of cardholders. However, to have a better understanding of the patterns and activity behaviors, in addition to collecting the data from smart cards, one should integrate them with other data set.
2.1.3 GPS data
In travel demand modeling, it is important to have accurate and complete travel survey data including trip purpose, length, and companions, travel demand, origin and destination, and time of the day. Since the 1990s, the global positioning system (GPS) became popular for civil engineering applications, especially in the field of transportation as it provides a means of tracking some of the above variables. In the literature, methods of processing the GPS data and identifying activities can be classified according to different approaches such as rule-based and Bayesian model [ 66 ]; fuzzy logic [ 67 ]; multilayer perceptron [ 68 ]; and support vector machine learning [ 69 ]. Nevertheless, the disadvantages of using GPS data include the cost, sample size limitation, and the need to retrieve and distribute GPS devices to participate. Since smartphones are becoming one of the human accessories while equipped with a GPS module, they can be considered as a replacement of the GPS device to gather travel data. In this regard, CDR from smartphones is used [ 70 ] to estimate origin-destination matrices, or a smartphone-based application is used [ 71 ] to map the semiformal minibus services in Kampala (Uganda) and to count passenger boarding and alighting [ 72 ]. In the Netherlands, the Mobidot application is developed for analyzing the mobility patterns of individuals. To deduce travel directions and modes, this application uses the real-time data gathered by sensors of smartphones including GPS, accelerometer, and gyroscope sensors to compare them with existing databases [ 73 ].
Applying smartphones as a replacement of GPS however, holds several restrictions including the draining of smartphone battery and it is not possible to record travel mode and purpose.
2.1.4 Social media data
Today transport modelers, planners, and managers have started to benefit from the popularity of social networking data. There are different kinds of social media data such as Twitter, Instagram, and LinkedIn data, which consist of normal text, hash-tag (#), and check-in data. As hash-tag and check-in data are related to an activity, location or event, they can be used as meaningful resources in analysis of destination/origin of the activity [ 74 ]. According to the literature, social media has a great influence on different aspects of travel demand modeling [ 75 ]. Using social media instead of traditional data collection methods was investigated in different studies [ 76 ]. The way of processing these data to extract useful information is challenging as investigated in different studies [ 77 , 78 ]. Various studies [ 79 , 80 , 81 , 82 ] also examined social media data to understand the mobility behavior of a large group of people. Testing the possibility of evaluating the origin-destination matrix based on location-based social data was researched [ 83 ] or in another similar studies [ 84 , 85 ] where Twitter data was used to estimate OD matrices. The comparison between this new OD with the traditional values produced by the 4-step model proved the great potential of using social media data in modeling aggregate travel behavior. Social media data can be used in other areas such as destination choice modeling [ 86 ], recognizing activity [ 87 ], understanding the patterns of choosing activity [ 80 , 88 , 89 ], and interpreting life-style behaviors via studying activity-location choice patterns [ 90 ].
2.2 Dynamic ABM using a multi-day travel data set
Most existing travel demand modelers have applied the household survey data during the period of one day to construct activity schedules. However, longer periods such as one week or one month gained substantial importance during recent years. For simulating everyday travel behavior and generating schedules, a one-week period provides more comprehensive coverage because it includes weekdays and weekends and represents the weekly routines of individuals in making trips. Periods longer than one week can further provide detail on personal behavior as well as various usage of modes in different ways. So far only a few travel demand models covered a typical week as a studied period. For example rhythm in activity-travel behavior based on the capacity of one week was presented by applying a Kuhn-Tucker method [ 41 ]. Few works have been concentrating on the generation of multiple-day travel dataset. For example, by using large data and surveys, Medina developed two discrete choice models for generating multi-day travel activity types based on the likeliness of the activity [ 91 ]. a sampling method based on activity-travel pattern type clustering [ 92 ] was proposed to extract multi-day activity-travel data according to single-day household travel data. The results show similarities in distributions of intrapersonal variability in multi-day and single-day. MATSim is a popular agent-based simulation for ABM research [ 93 , 94 ], however, it is not appropriate for modeling the multi-day scenarios because MATSim uses the co-evolutionary algorithm to reach the user equilibrium which is a time consuming particularly for multi-day plans. To solve these problems, Ordonez [ 95 ] proposed a differentiation between fixed and flexible activities. Based on different time scales, Lee examined three levels of travel behavior dynamics, namely micro-dynamics (24 hours), macro-dynamics (lifelong travel behavior), meso-dynamics (weekly/monthly/yearly basis) by applying different statistical models [ 96 ]. A learning day-by-day module in another agent-based simulation software SimMobility is proposed [ 97 ]. Furthermore, ADAPTS is one of the few activity-based travel demand models which depends on activity planning horizon data for a longer period than one day, for example, one week or one month [ 33 ].
As highlighted by the above literature review, applying one-day observation data in travel demand modeling provides an inadequate basis of understanding of complex travel behavior to predict the impact of travel demand management strategies. So multi-day data are needed to refine this process. Previously, it was not easy to collect multi-day data, however, today thanks to advantages to technology it is possible to extract data from GPS, smartphones, smart cards, etc. with no burden for the respondent. Models built based on GPS data have been found to be more accurate and precise due to having fewer measurement errors. Collecting call detail records from mobile phones provide modelers with large trip samples and origin-destination matrices, while smart card data are more useful in terms of validation.
3. ABM transferability
We now turn to the recent advances and ongoing research in ABM focused on testing and enhancing geographical transferability and capacity to predict a broader range of impacts than flows and performance of the transport network.
3.1 ABM transferability from one geographical context to another
The spatial transferability of a travel demand model happens when the information or theory of a developed model of one region is applied to a new context [ 98 ]. Transferability can be used not only as a beneficial validation test for the models but also to save the cost and time required to develop a new model. Validation of a model by testing spatial transferability beside other various methods such as base-year and future-year data set is a test of validity which represents the capability of activity-based models in predicting travel behavior in a different context [ 99 ]. The exact theoretical basis and behavioral realism of activity-based travel demand model make them more appropriate for geographic transferability in comparison to traditional trip-based models [ 100 ]. Testing the transferability of ABM was first investigated by Arentze et al. [ 101 ]. They examined the possibility of transferring the ALBATROSS model at both individual and aggregate levels for two municipalities (Voorhout and Apeldoorn) in the Netherlands by simulating activity patterns. The results were satisfactory except for the transportation mode choice. In the United States, the CT-RAMP activity-based model which was developed for the MORPC region then transferred to Lake Tahoe [ 102 ]. In another study, one component of the ADAPTS model showed the potential for having good transferability properties [ 31 ]. The transferability of the DaySim model system developed for Sacramento to four regions in California and two other regions in Florida was investigated in [ 103 ]. The results show that the activity generation and scheduling models can be transferred better than mode and location choice models. The CEMDAP model developed for Dallas Fort Worth (DFW) region was transferred to the southern California region [ 104 ]. Outside the U.S., the TASHA model system developed for Toronto was transferred to London [ 105 ], and also in another study [ 106 ] the transferability of TASHA to the context of the Island of Montreal was assessed. Activity generation, activity location choice, and activity scheduling were three components of TASHA that transferred from Toronto to Montreal. In general, TASHA provided acceptable results at (macro and meso-level) for work and school activities even in some cases better results for Montreal in comparison to Toronto area. The possibility of developing a local area activity-based transport demand model for Berlin by transferring an activity generation model from another geographical area (Los Angeles) and applying the traffic counts of Berlin was investigated [ 107 ]. In their research, the CEMDAP model was applied to achieve a set of possible activity-travel plans, and the MATSim simulation was then used to generate a representative travel demand for the new region. The results were quite encouraging, however, the study indicated a need for further evaluation. In one recent study [ 108 ], an empirical method was used to check the transferability of ABMs between regions. According to their investigations, the most difficult problems with transferability caused by parameters of travel time, travel cost, land use, and logsum accessibilities. They suggested that in the transferability of the ABM from another region, agencies should be aware of finding a region within the same state or with similar urban density, or preferably both in order to improve the results. The possibility of transferring the FEATHERS model to Ho Chi Minh in Vietnam is investigated [ 109 ]. FEATHERS initially is developed for Flanders in Belgium. After calibration of FEATHERs sub-models, testing results using different indicators confirmed the success of transferring the FEATHER’s structure to the new context.
At the theoretical level, a perfect transferable model contributes to the transferability of its underlying behavioral theory, model structure, variable specification and coefficient to the new context. However, perfect transferability is not easy to achieve due to different policy and planning needs as well as the size of the regions, and the availability of data and other resources. Although the results of several transferred ABM model systems seem to have worked reasonably, it is equally important to assess how much accuracy is important in transferring models and how best and where to transfer models from.
3.2 ABM transferability to other non-transport domain
One of the advantages of the activity-based travel demand models over trip-based models is its capability to generate various performance indicators such as emission, health-related indicators, social exclusion, well-being, and quality of life indicators. Application of disaggregate models for the area of emission and air quality analysis was introduced by Shiftan [ 110 ] who investigated the Portland activity-based model in comparison to trip-based models. In another study [ 111 ], the same author integrated the Portland activity-based model with MOBILE5 emission model to study the effects of travel demand techniques on air quality. Regarding the integration of ABM with the emission model, the Albatross ABM model was coupled with MIMOSA (macroscopic emission model) [ 112 ] considering the usage of fuel and the amount of produced emission as a function of travel speed. A study in [ 113 ] added one dispersion model (AUROTA) to the previous integration of Albatross and MIMOSA to predict the hourly ambient pollutant. Albatross linked with a probabilistic air quality system was employed [ 114 ] in air quality assessment study. TASHA was another activity-based model, which has been extensively employed in air quality studies. For example, this model was integrated [ 29 , 115 ] with MOBILE6.2 to quantify vehicle emissions in Toronto. In their study, EMME/2 was used in the traffic assignment part. The previous research was improved [ 116 ] by replacing EMME/2 with MATSim as an agent-based DTA model. This TASHA-MATSim chain was used in the research [ 117 ] with the integration of MOBILE6.2C (emission model) and CALPUFF (dispersion model). OpenAMOS linked with MOVES emission model [ 118 ], and ADAPTS linked with MOVES [ 119 ] together with Sacramento ABM model [ 120 ] are among recent studies which represented the application of activity-based models in analyzing the impacts of vehicular emissions.
Human well-being and personal satisfaction play an important role in social progression [ 121 ]. To understand the theory behind human happiness, transport policies concentrated on the concept of utility as a tool to increase activity, goods, and services [ 122 , 123 ]. The issue of well-being as a policy objective is addressed in the literature and measured through various indicators, which show personal satisfaction and growth. For example, in the study by Hensher and Metz [ 124 , 125 ], saving time which leads to engagement in more activities was introduced as one of the benefits of measuring transport performance. Spatial accessibility was another benefit of travel that provides a range of activities that can be reasonably reached by individuals [ 126 ]. A dynamic ordinal logit model was developed [ 127 ] based on the collected data on happiness for a single activity in Melbourne. The authors found different activity types, which have different influences on the happiness that each individual experienced. Well-being can be integrated into activity-based models based on random utility theory. In terms of modeling, a framework was introduced [ 122 ] considering well-being data to improve activity-based travel demand models. According to their hypothesis, well-being is the final aim of activity patterns. They applied a random utility framework and considered well-being measures as indicators of the utility of activity patterns, and planned to test their framework empirically by adding well-being measurement equations to the DRCOG’s activity-based model.
The above literature review showed the importance of applying traffic models to generate input data to other models such as the air quality model. The accuracy of emission models is highly dependent on the level of detail in transport demand model inputs. Activity-based and agent-based models are supposed to describe reality more accurately by providing more detailed traffic data. Beyond measurement of air quality, well-being and health have drawn increasing attention. The health impact of changes in travel behavior, health inequalities, and social justice can be assessed within the activity-based platform [ 128 ]. With the help of geospatial data acquisition technologies like GPS, behavioral information with health data can be integrated into the development of an activity-based model to provide policies that affect the balance of transport and well-being.
3.3 ABM integration with dynamic traffic assignment
In parallel with the travel demand modeling, on the supply side, the conventional supply models used to be static, which import constant origin-destination flows as an input and produce static congestion patterns as an output. Consequently, these models were unable to represent the flow dynamics in a clear and detailed manner. Dynamic traffic assignment (DTA) models have emerged to address this issue and are capable of capturing the variability of traffic conditions throughout the day. It is evident that the shift of analysis from trips to activities in the demand modeling, as well as, the substitution of the static traffic assignment with dynamic traffic assignment in the supply side, can provide more realistic results in the planning process. Furthermore, the combination of ABM and DTA can better represent the interactions between human activity, their scheduling decision, and the underlying congested networks. Nevertheless, according to the study of [ 11 ], the integration of ABM with DTA received little attention and still requires further theoretical development. There are different approaches to the integration of ABM and DTA, which started with a sequential integration. In this type of integration, exchanging data between two major model components (ABM and DTA) happens at the end of the full iteration, to generate daily activity patterns for all synthetic population in an area of study, the activity-based model is run for the whole period of a complete day. The outputs of the ABM model which are lists of activities and plans are then fed into the DTA model. The DTA model generates a new set of time-dependent skim matrices as inputs to ABM for the next iteration. This process is continued until the convergence will be reached in the OD matrices output. Model systems applying the sequential integration paradigm can be found in most of the studies in the literature. For example, Castiglione [ 129 ] integrated DaySim which is an activity-based travel demand model developed for Sacramento with a disaggregate dynamic network traffic assignment tool TRANSIMS router. Bekhor [ 130 ] investigated the possibility of coupling the Tel Aviv activity-based model with MATSim as an agent-based dynamic assignment framework. Hao [ 116 ] integrated the TASHA model with MATSim. Ziemke [ 107 ] integrated CEMDAP, which is an activity-based model with MATSim to check the possibility of transferring an activity-based model from one geographic region to another. Lin [ 131 ] introduced the fixed-point formulation of integrated CEMDAP as an activity-based model with an Interactive System for Transport Algorithms (VISTA). Based on the mathematical algorithm of household activity pattern problem (HAPP), ABM and DTA were integrated [ 132 ] by presenting the dynamic activity-travel assignment model (DATA) which is an integrated formulation in the multi-state super network framework.
In the sequential integration, the ABM and DTA models run separately until they reach convergence. At the end of an iteration, these models perform data exchange before iterate again. Therefore, this kind of integrated framework cannot react quickly and positively to network dynamics and is unable to adapt to real-time information available to each traveler. In addressing this limitation, integrated models that adopt a much tighter integration framework have been developed recently. This approach is quite similar to the sequential approach, however; the resolution of time for ABM simulation is one minute rather than 24 hours (complete day). Relating to this level of dynamic integration, Pendyala [ 133 ] investigated the possibility of integrating OpenAMOS which is an activity-travel demand model with DTA tool name MALTA (Multiresolution Assignment and loading of traffic activities) with appropriate feedback to the land-use model system. For increasing the level of dynamic integration of ABM and DTA models, dynamic integration having pre-trip enroute information with full activity-travel choice adjustments has been introduced. In this level of ABM & DTA integration, it is assumed that pre-trip information is available for travelers about the condition of the network. It means that travelers are capable of adjusting activity-travel choices since they have access to pre-trip and Enroute travel information. Another tightly integrated modeling framework was proposed in [ 134 ] to integrate ABM (openAMOS) and DTA (DTALite) to capture activity-travel demand and traffic dynamics in an on-line environment. This model is capable of providing an estimation of traffic management strategies and real-time traveler information provision. Zockaie et al. [ 135 ] presented a simulation framework to integrate the relevant elements of an activity-based model with a dynamic traffic assignment to predict the operational impacts related to congestion pricing policies. Auld et al. [ 38 ] developed an agent-based modeling framework (POLARIS) which integrates dynamic simulation of travel demand, network supply, and network operations to solve the difficulty of integrating dynamic traffic assignment, and disaggregate demand models. A summary of the current literature on ABM and DTA integration is presented in Table 2 .
A summary of the empirical literature on ABM and DTA integration.
The above discussion illustrates that most of the model integration platforms between ABM + DTA work based on sequential integration. This loose coupling platform is the most straightforward and popular approach albeit is not responsive to network short-term dynamics and real-time information. Efforts to develop a comprehensive simulation model that can account for all components of dynamic mobility and management strategies continue. Further developments will have to deal with the implementation of an integrated ABM + DTA platform on a large network to support decision-makers, focus on the integration between activity-based demand models and multimodal assignment [ 143 ] as well as reducing computational efforts via better data exchange procedure and improving model communication efficiency. Defining practical convergence criteria is another issue which needs further investigations. Fully realistic convergence is normally never happened in sequential integration due to applying a pre-defined number of feedback loops in order to save model runtime.
3.4 ABM and travel demand management applications
Travel demand management (TDM) strategies are implemented to increase the efficiency of the transportation system and reduce traffic-related emissions. Some examples include mode shift strategies (encouraging people to use public transport) [ 144 ], time shift (to ride in off-peak hours, congestion pricing), and travel demand reduction [ 145 ] (using shared mobility service or teleworking). Shared transport services including car sharing, bike sharing, and ridesharing have been implemented in most of the transport planning systems across the world. Applying activity-based travel demand models to study the optimal fleet size can be found in different studies in the literature [ 146 , 147 ]. Parking price policies and their impacts on car sharing were investigated using MATSim in [ 148 ]. Results show shared vehicles use more efficient parking spaces in comparison to private vehicles. In the first attempt to model car sharing on more than one typical day [ 149 ] the agent-based simulation (mobitopp) was extended with a car-sharing option to study the travel behavior of the population in the city of Stuttgart in one week. In the recent study of [ 150 ], car sharing was integrated into an activity-based dynamic user equilibrium model to show the interaction between the demand and supply of car sharing. Among all the TDM strategies, telecommuting can be implemented in a shorter time [ 151 , 152 , 153 ]. The results of these studies present a reduction in vehicle-kilometers-traveled (VKT) during peak hours mainly because telecommuters change their trip timetable during these times. This plan rescheduling is also investigated and addressed in different studies [ 154 ] based on the statistical analysis of worker’s decisions about choice and frequency of telecommuting. While the plan rescheduling leads to reducing commute travel, the overall impacts of telecommuting on the formation of worker’s daily activity-travel behavior is challenging. For example, this policy reduced total distance traveled by 75% on telecommuting days while telecommuting could reduce the total commute distance up to 0.8% and 0.7% respectively [ 151 , 155 ]. Based on the adoption and frequency of telecommuting, a joint discrete choice model of home-based commuting was developed for New York city using the revealed preference (RP) survey [ 156 ]. Their results show a powerful relationship among individuals’ attributes, households’ demographics, and work-related factors, and telecommuting adoption and frequency decisions. A similar study [ 157 ] estimated the telecommuting choice and frequency by using a binary choice model and ordered-response model respectively. In terms of using activity-based modeling, [ 158 ] POLARIS activity-based framework was applied to research telecommuting adoption behavior and apply MOVES emission simulator model to assess the consequences of implementing this policy on air quality. Their results show that considering 50% of workers in Chicago with flexible working time hours in comparison to the base case with 12% flexible time hour workers, telecommuting can reduce Vehicle Mile Traveled (VMT) and Vehicle Hour Traveled (VHT) by 0.69% and 2.09% respectively. This policy reduces greenhouse gas by up to 0.71% as well. Pirdavani et al. [ 159 ] investigated the impact of two TDM scenarios (increasing fuel price and considering teleworking) on traffic safety. In this work, FEATHERS model, which is an activity-based model, was applied to produce exposure matrices to have a more reliable assessment. The results show the positive impacts of two scenarios on safety ( Figure 2 ).

Travel demand management policies within the activity-based platform.
The above section explores the relationship between transport demand management policies and travel behavior in the ABM context. The use of an activity-based travel demand model provides flexibility to employ a range of policy scenarios, and at the same time, the results are as detailed as possible to obtain the impact of policies on a disaggregated level. The finding highlights the importance of implementing different transportation policies management together to reach the most appropriate effect in terms of improving sustainability and the environment. The discussion emphasizes the need for considering more comprehensive transportation and environmental policies concerning sustainability to tackle travel planning in light of the increasingly diverse and complex travel patterns.
4. Summary and research directions
The use of activity-based models to capture complex underlying human’s travel behavior is growing. In this paper, we began by introducing the components of activity-based models and the evolution of the existing developed ABM models. In the first part of this paper, the new resources of data for travel demand analysis were introduced. In the new era of travel demand modeling, we need to deal with a dynamic, large sample, time-series data provided from new devices, and as a result manage observation covering days, weeks, and even months. The outcome of the recent works revealed that since activity-based models originated from the concept of individual travel patterns rather than aggregate flows, they highly suited to these new big data sources. These big datasets, which document human movements, include the information about mobility traces and activities carried out. Based on the in-depth and critical review of the literature, it is clear that while these big datasets provide detailed insight into travel behavior, challenges remain in extracting the right information and appropriately integrating them into the travel demand models. In particular, extracting personal characteristics and trip information like trip purpose and mode of transport are still open problems as these big data resources which provide space-time traces of trip-maker behaviors. Research works along these lines have been started as it was reviewed in the first part; however, further researches should be conducted to handle the uncertainty of big data mobility traces in the modeling process. Also, new methods should be investigated to validate the results for each step of the data analysis and mining. The possibility of fusing data from different available datasets needs further investigation. For instance, to understand the mode inference both data from the smart card and CDRs can be analyzed simultaneously. Another challenging issue regarding the application of this rich new data in transport modeling is that the need for methodologies to extract useful information needed regarding the traveler’s in-home and out-of-home activity patterns, which highlights the combination of data science, soft computing-based approaches, and transport research methods. It requires new Different algorithms such as statistical, genetic, evolutionary, and fuzzy as well as different techniques including advanced text and data mining, natural language processing, and machine learning.
The spatial transferability of activity-based travel demand models remains an important issue. Generally, it is found that the transferability of these models is more feasible than trip-based models, especially between two different regions with similar density or even between two areas in the same state. To date, most of the transferability research in activity-based travel demand modeling is motivated by a desire to save time, and very few studies that applied spatial transferability of activity-based models have undertaken rigorous validation of the results. While literature showed successful model transferability in terms of transferring activity/tour generation, time-of-day choice components, more studies are required on the model transferability regarding mode and location choice models as well as the validation test of activity-based models in different levels, i.e., micro, meso, and macro models.
As part of the second section of this study, this paper reviewed the progress made in the integration of activity-based models with dynamic traffic assignment.
Based on the literature, although evolution has occurred in DTA models, the loose coupling (sequential method) between ABM and DATA models still dominate the field. Two main challenges remain, namely poor convergence quality and excessively long run time. Replacing MATSim as a dynamic traffic assignment tool with other route assignment algorithms in recent years was a technical solution to loose coupling, which considered route choice as another facet of a multi-dimensional choice problem. MATSim provides not only an integration between the demand and supply side, but it can also act as a stand-alone agent-based modeling framework. However; MATSim potential drawbacks include being based on unrealistic assumptions of utility maximization and perfect information. To remove these unrealistic rational behavioral assumptions, applying other approaches such as a new innovative method of behavioral user equilibrium (BUE) is needed. This method helps trip-makers to reach certain utility-level rather than maximize the utility of their trip making [ 160 ]. Work along this approach has started (e.g., [ 161 ]).
The capability of activity-based models in generating other kinds of performance indicators in addition to OD matrices was also reviewed. Literature proved activity-based models generate more detailed results as inputs to air quality models, however; error rises from the accuracy of the information has a relevant impact on the process of integration. So it is necessary to do a comprehensive analysis of the uncertainties in traffic data. Literature proved that despite of the improvements in such disaggregate frameworks and the capability of these models in replicating policy sensitive simulation environment; there is yet to develop the best and perfect traffic-emission-air quality model. While the issue of health has drawn extensive attention from many fields, activity-based travel demand models have proved to have the potential to be used in estimating health-related indicators such as well-being. However, very few studies have been found to investigate the theories required to extend the random utility model based on happiness. While it is proved that mobility and environment have direct impacts on transport-related health [ 162 ], investigations on how travel mode preferences and air pollution exposure are related in this context are needed. Another area of research within ABM platform which is yet to be studied is the relationship between individual exposure to air pollution and mobility, especially in space, and time.
In the last part of this paper, the capability of activity-based models in the analysis of traffic demand management was investigated. Generally, the influence of telecommuting on both travel demand and network operation is still incomplete. Very few studies were found in which activity-based framework is used to simulate the potential impacts of telecommuting on traffic congestion and network operation where the real power of activity-based models lie.
In conclusion, while there are still open problems in activity-based travel demand models, there has been a lot of progress being made which is evidenced by the various recent and on-going researches reviewed in this paper. The review showed that by applying different methodologies in the modeling of different aspects of activity-based models, these models are becoming more developed, robust, and practical and become an inevitable tool for transport practitioners, city planners, and policy decision-makers alike.
Acknowledgments
The research work presented in this paper was supported by the Australian Government-Department of Education under Research Training Program (RTP Stipend) award.
- 1. Vovsha, P., M. Bradley, and J.L. Bowman. Activity-based travel forecasting models in the United States: progress since 1995 and prospects for the future . in at EIRASS Conference on Progress in Activity-Based Analysis . 2005. Vaeshartelt Castle, Maastricht.
- 2. Joe Castiglione, M.B., and John Gliebe, Activity-Based Travel Demand Models A Primer , in SHRP 2 Report S2-C46-RR-1 . 2015, TRANSPORTATION RESEARCH BOARD: Washington, D.C.
- 3. McNally, M.G. and C.R. Rindt, The activity-based approach , in Handbook of Transport Modelling: 2nd Edition . 2007, Emerald Group Publishing Limited. p. 55-73.
- 4. Henson, K., K. Goulias, and R. Golledge, An assessment of activity-based modeling and simulation for applications in operational studies, disaster preparedness, and homeland security. Transportation Letters, 2009. 1 (1): p. 19-39.
- 5. Davidson, B., P. Vovsha, and J. Freedman. New advancements in activity-based models . in Australasian Transport Research Forum . 2011.
- 6. Chu, Z., L. Cheng, and H. Chen, A Review of Activity-Based Travel Demand Modeling , in CICTP 2012: Multimodal Transportation Systems—Convenient, Safe, Cost-Effective, Efficient . 2012. p. 48-59.
- 7. Zhong, M., et al., A comparative analysis of traditional four-step and activity-based travel demand modeling: a case study of Tampa, Florida. Transportation Planning and Technology, 2015. 38 (5): p. 517-533.
- 8. Subbarao, S.S. and K. Krishnarao, Activity based approach to travel demand modelling: An overview. EUROPEAN TRANSPORT-TRASPORTI EUROPEI, 2016 (61).
- 9. Daisy, N.S.M., Hugh; Liu, Lei, Individuals’ Activity-Travel Behavior in Travel Demand Models: A Review of Recent Progress , in 18th COTA International Conference of Transportation Professionals . 2018: Beijing, China.
- 10. Pinjari, A.R. and C.R. Bhat, Activity-based travel demand analysis. A Handbook of Transport Economics, 2011. 10 : p. 213-248.
- 11. Rasouli, S., and Harry Timmermans, Activity-Based Models of Travel Demand: Promises, Progress and Prospects. International Journal of Urban Sciences 2014. 18(1) : p. 31-60
- 12. Lenntorp, B., Paths in space-time environment: A time geographic study of possibilities of individuals. The Royal University of Lund, Department of Geography. Lund Studies in Geography, Series B. Human Geography, 1976. 44 .
- 13. Jones, P., et al., Understanding Travel Behavior Gower Publishing Co. Ltd. Aldershot, UK Google Scholar, 1983.
- 14. Huigen, P.P., Binnen of buiten bereik?: Een sociaal-geografisch onderzoek in Zuidwest-Friesland . 1986: na.
- 15. Dijst, M. and V. Vidakovic, INDIVIDUAL ACTION SPACE IN THE CITY. Activity-based approaches to travel analysis, 1997.
- 16. Kwan, M.-P., GISICAS: AN ACTIVITY-BASED TRAVEL DECISION SUPPORT SYSTEM USING A GIS-INTERFACED COMPUTATIONAL-PROCESS MODEL. Activity-based approaches to travel analysis, 1997.
- 17. Bradley, M.A., Portland Metro, J. L. Bowman, A system of activitybased models for Portland, Oregon , in USDOT report number DOT-T-99-02 . 1998: Washington, D.C.
- 18. Bradley, M., et al. Estimation of an activity-based micro-simulation model for San Francisco . in 80th Annual Meeting of the Transportation Research Board, Washington DC . 2001.
- 19. Vovsha, P., E. Petersen, and R. Donnelly, Microsimulation in travel demand modeling: Lessons learned from the New York best practice model. Transportation Research Record: Journal of the Transportation Research Board, 2002(1805): p. 68-77.
- 20. Consult, P.B., The MORPC travel demand model: Validation and final report. Prepared for the Mid-Ohio Region Planning Commission, 2005.
- 21. Bowman, J. and M. Bradley, Activity-based travel forecasting model for SACOG: Technical memos numbers 1-11. Available at* HYPERLINK" http://jbowman.net "* http://jbowman.net , 2005.
- 22. Bradley, M. and J. Bowman. A summary of design features of activity-based microsimulation models for US MPOs . in White Paper for the Conference on Innovations in Travel Demand Modeling, Austin, TX . 2006.
- 23. Bhat, C., et al., Comprehensive econometric microsimulator for daily activity-travel patterns. Transportation Research Record: Journal of the Transportation Research Board, 2004(1894): p. 57-66.
- 24. Pinjari, A.R., et al., Activity-based travel-demand analysis for metropolitan areas in Texas: CEMDAP Models, Framework, Software Architecture and Application Results . 2006.
- 25. Pendyala, R., et al., Florida activity mobility simulator: overview and preliminary validation results. Transportation Research Record: Journal of the Transportation Research Board, 2005(1921): p. 123-130.
- 26. Davidson, W., et al. CT-RAMP family of activity-based models . in Proceedings of the 33rd Australasian Transport Research Forum (ATRF) . 2010.
- 27. Arentze, T. and H. Timmermans, Albatross: a learning based transportation oriented simulation system . 2000: Citeseer.
- 28. Arentze, T.A. and H.J. Timmermans, A learning-based transportation oriented simulation system. Transportation Research Part B: Methodological, 2004. 38 (7): p. 613-633.
- 29. Miller, E. and M. Roorda, Prototype model of household activity-travel scheduling. Transportation Research Record: Journal of the Transportation Research Board, 2003(1831): p. 114-121.
- 30. Roorda, M.J. and E.J. Miller, Strategies for resolving activity scheduling conflicts: an empirical analysis. 2005.
- 31. Auld, J. and A. Mohammadian, Framework for the development of the agent-based dynamic activity planning and travel scheduling (ADAPTS) model. Transportation Letters, 2009. 1 (3): p. 245-255.
- 32. Auld, J.A. and A. Mohammadian. Planning Constrained Destination Choice Modeling in the Adapts Activity-Based Model . in Proceedings of the Transportation Research Board 90th Annual Meeting, Washington, DC, USA . 2011.
- 33. Auld, J. and A.K. Mohammadian, Activity planning processes in the Agent-based Dynamic Activity Planning and Travel Scheduling (ADAPTS) model. Transportation Research Part A: Policy and Practice, 2012. 46 (8): p. 1386-1403.
- 34. Bellemans, T., et al., Implementation framework and development trajectory of FEATHERS activity-based simulation platform. Transportation Research Record: Journal of the Transportation Research Board, 2010(2175): p. 111-119.
- 35. Balmer, M., K. Axhausen, and K. Nagel, Agent-based demand-modeling framework for large-scale microsimulations. Transportation Research Record: Journal of the Transportation Research Board, 2006(1985): p. 125-134.
- 36. Nagel, K., R.L. Beckman, and C.L. Barrett. TRANSIMS for transportation planning . in In 6th Int. Conf. on Computers in Urban Planning and Urban Management . 1999. Citeseer.
- 37. Adnan, M., et al. Simmobility: A multi-scale integrated agent-based simulation platform . in 95th Annual Meeting of the Transportation Research Board Forthcoming in Transportation Research Record . 2016.
- 38. Auld, J., et al., POLARIS: Agent-based modeling framework development and implementation for integrated travel demand and network and operations simulations. Transportation Research Part C: Emerging Technologies, 2016. 64 : p. 101-116.
- 39. Kagho, G.O., M. Balac, and K.W. Axhausen, Agent-based models in transport planning: Current state, issues, and expectations. Procedia Computer Science, 2020. 170 : p. 726-732.
- 40. Miller, E.J., Travel demand models, the next generation: Boldly going where no-one has gone before , in Mapping the Travel Behavior Genome . 2020, Elsevier. p. 29-46.
- 41. Nurul Habib, K., E. Miller, and K. Axhausen, Weekly rhythm in joint time expenditure for all at-home and out-of-home activities: application of Kuhn-Tucker demand system model using multiweek travel diary data. Transportation Research Record: Journal of the Transportation Research Board, 2008(2054): p. 64-73.
- 42. Anda, C., A. Erath, and P.J. Fourie, Transport modelling in the age of big data. International Journal of Urban Sciences, 2017. 21 (sup1): p. 19-42.
- 43. Zhao, Z., J. Zhao, and H.N. Koutsopoulos, Individual-Level Trip Detection using Sparse Call Detail Record Data based on Supervised Statistical Learning . 2016.
- 44. Caceres, N., J. Wideberg, and F. Benitez, Deriving origin–destination data from a mobile phone network. IET Intelligent Transport Systems, 2007. 1 (1): p. 15-26.
- 45. Wang, P., et al., Understanding road usage patterns in urban areas. Scientific reports, 2012. 2 : p. 1001.
- 46. Farrahi, K. and D. Gatica-Perez, Discovering routines from large-scale human locations using probabilistic topic models. ACM Transactions on Intelligent Systems and Technology (TIST), 2011. 2 (1): p. 3.
- 47. Phithakkitnukoon, S., et al. Activity-aware map: Identifying human daily activity pattern using mobile phone data . in International Workshop on Human Behavior Understanding . 2010. Springer.
- 48. Widhalm, P., et al., Discovering urban activity patterns in cell phone data. Transportation, 2015. 42 (4): p. 597-623.
- 49. Yin, M., et al., A generative model of urban activities from cellular data. IEEE Transactions on Intelligent Transportation Systems, 2017.
- 50. Schneider, C.M., et al., Unravelling daily human mobility motifs. Journal of The Royal Society Interface, 2013. 10 (84): p. 20130246.
- 51. Jiang, S., et al. A review of urban computing for mobile phone traces: current methods, challenges and opportunities . in Proceedings of the 2nd ACM SIGKDD international workshop on Urban Computing . 2013. ACM.
- 52. Jiang, S., J. Ferreira, and M.C. Gonzalez, Activity-based human mobility patterns inferred from mobile phone data: A case study of Singapore. IEEE Transactions on Big Data, 2017. 3 (2): p. 208-219.
- 53. Zilske, M. and K. Nagel, Studying the accuracy of demand generation from mobile phone trajectories with synthetic data. Procedia Computer Science, 2014. 32 : p. 802-807.
- 54. Zilske, M. and K. Nagel, A simulation-based approach for constructing all-day travel chains from mobile phone data. Procedia Computer Science, 2015. 52 : p. 468-475.
- 55. Bassolas, A., et al., Mobile phone records to feed activity-based travel demand models: MATSim for studying a cordon toll policy in Barcelona. Transportation Research Part A: Policy and Practice, 2019. 121 : p. 56-74.
- 56. Devillaine, F., M. Munizaga, and M. Trépanier, Detection of activities of public transport users by analyzing smart card data. Transportation Research Record: Journal of the Transportation Research Board, 2012(2276): p. 48-55.
- 57. Ceapa, I., C. Smith, and L. Capra. Avoiding the crowds: understanding tube station congestion patterns from trip data . in Proceedings of the ACM SIGKDD international workshop on urban computing . 2012. ACM.
- 58. da Silva, T.L.C., J.A. de Macêdo, and M.A. Casanova. Discovering frequent mobility patterns on moving object data . in Proceedings of the Third ACM SIGSPATIAL International Workshop on Mobile Geographic Information Systems . 2014. ACM.
- 59. Liu, Y., et al., Intelligent bus routing with heterogeneous human mobility patterns. Knowledge and Information Systems, 2017. 50 (2): p. 383-415.
- 60. Zhang, F., et al., Spatiotemporal segmentation of metro trips using smart card data. IEEE Transactions on Vehicular Technology, 2016. 65 (3): p. 1137-1149.
- 61. Zhao, J., et al., Estimation of passenger route choice pattern using smart card data for complex metro systems. IEEE Transactions on Intelligent Transportation Systems, 2017. 18 (4): p. 790-801.
- 62. Bouman, P., et al., Recognizing demand patterns from smart card data for agent-based micro-simulation of public transport. 2012.
- 63. Chakirov, A. and A. Erath, Activity identification and primary location modelling based on smart card payment data for public transport. [Working paper/Transport and Spatial Planning], 2012. 786 .
- 64. Fourie, P.J., et al., Using smartcard data for agent-based transport simulation , in Public Transport Planning with Smart Card Data . 2016, CRC Press. p. 133-160.
- 65. Zhu, Y., Extract the Spatiotemporal Distribution of Transit Trips from Smart Card Transaction Data: A Comparison Between Shanghai and Singapore , in Big Data Support of Urban Planning and Management . 2018, Springer. p. 297-315.
- 66. Patterson, D.J., et al. Inferring high-level behavior from low-level sensors . in International Conference on Ubiquitous Computing . 2003. Springer.
- 67. Schuessler, N. and K. Axhausen, Processing raw data from global positioning systems without additional information. Transportation Research Record: Journal of the Transportation Research Board, 2009(2105): p. 28-36.
- 68. Rudloff, C. and M. Ray, Detecting travel modes and profiling commuter habits solely based on GPS data . 2010.
- 69. McGowen, P. and M. McNally. Evaluating the potential to predict activity types from GPS and GIS data . in Transportation Research Board 86th Annual Meeting, Washington . 2007. Citeseer.
- 70. Demissie, M.G., et al., Inferring passenger travel demand to improve urban mobility in developing countries using cell phone data: a case study of Senegal. IEEE Transactions on Intelligent Transportation Systems, 2016. 17 (9): p. 2466-2478.
- 71. Ndibatya, I., J. Coetzee, and T. Booysen, Mapping the informal public transport network in Kampala with smartphones: international. Civil Engineering = Siviele Ingenieurswese, 2017. 2017 (v25i1): p. 35-40.
- 72. Saddier, S., et al., Mapping the Jitney network with smartphones in Accra, Ghana: the AccraMobile experiment. Transportation Research Record: Journal of the Transportation Research Board, 2016(2581): p. 113-122.
- 73. Bisseling, R., et al., Inferring transportation modes from smartphone sensors. Proceedings 106th European Study Group Mathematics with Industry, 2016: p. 21-34.
- 74. Cheng, Z., et al., Exploring millions of footprints in location sharing services. ICWSM, 2011. 2011 : p. 81-88.
- 75. Rashidi, T.H., et al., Exploring the capacity of social media data for modelling travel behaviour: Opportunities and challenges. Transportation Research Part C: Emerging Technologies, 2017. 75 : p. 197-211.
- 76. Golder, S.A. and M.W. Macy, Digital footprints: Opportunities and challenges for online social research. Annual Review of Sociology, 2014. 40 .
- 77. Cramer, H., M. Rost, and L.E. Holmquist. Performing a check-in: emerging practices, norms and'conflicts' in location-sharing using foursquare . in Proceedings of the 13th international conference on human computer interaction with mobile devices and services . 2011. ACM.
- 78. Maghrebi, M., et al. Complementing travel diary surveys with Twitter data: application of text mining techniques on activity location, type and time . in Intelligent Transportation Systems (ITSC), 2015 IEEE 18th International Conference on . 2015. IEEE.
- 79. Zhu, Z., U. Blanke, and G. Tröster. Inferring travel purpose from crowd-augmented human mobility data . in Proceedings of the First International Conference on IoT in Urban Space . 2014. ICST (Institute for Computer Sciences, Social-Informatics and Telecommunications Engineering).
- 80. Hasan, S. and S.V. Ukkusuri, Social contagion process in informal warning networks to understand evacuation timing behavior. Journal of Public Health Management and Practice, 2013. 19 : p. S68-S69.
- 81. Noulas, A., et al., A tale of many cities: universal patterns in human urban mobility. PloS one, 2012. 7 (5): p. e37027.
- 82. Jurdak, R., et al., Understanding human mobility from Twitter. PloS one, 2015. 10 (7): p. e0131469.
- 83. Cebelak, M.K., Location-based social networking data: doubly-constrained gravity model origin-destination estimation of the urban travel demand for Austin, TX. 2013.
- 84. Lee, J.H., et al., Activity space estimation with longitudinal observations of social media data. Transportation, 2016. 43 (6): p. 955-977.
- 85. Lee, J.H., S. Gao, and K.G. Goulias. Can Twitter data be used to validate travel demand models . in 14th International Conference on Travel Behaviour Research . 2015.
- 86. Hasnat, M.M., et al., Destination choice modeling using location-based social media data. Journal of choice modelling, 2019. 31 : p. 22-34.
- 87. Lian, D. and X. Xie. Collaborative activity recognition via check-in history . in Proceedings of the 3rd ACM SIGSPATIAL International Workshop on Location-Based Social Networks . 2011. ACM.
- 88. Pianese, F., et al. Discovering and predicting user routines by differential analysis of social network traces . in World of Wireless, Mobile and Multimedia Networks (WoWMoM), 2013 IEEE 14th International Symposium and Workshops on a . 2013. IEEE.
- 89. Coffey, C. and A. Pozdnoukhov. Temporal decomposition and semantic enrichment of mobility flows . in Proceedings of the 6th ACM SIGSPATIAL International Workshop on Location-Based Social Networks . 2013. ACM.
- 90. Hasan, S. and S.V. Ukkusuri, Location contexts of user check-ins to model urban geo life-style patterns. PloS one, 2015. 10 (5): p. e0124819.
- 91. Medina, S.A.O., Inferring weekly primary activity patterns using public transport smart card data and a household travel survey. Travel Behaviour and Society, 2016.
- 92. Zhang, A., et al. Multi-day activity-travel pattern sampling based on single-day data . in 97th Annual Meeting of the Transportation Research Board (TRB 2018) . 2018. TRB Annual Meeting.
- 93. Horni, A. and K.W. Axhausen, MATSim Agent Heterogeneity and a One-Week Scenario. ETH, Eidgenössische Technische Hochschule Zürich, IVT, Institut für Verkehrsplanung und Transportsysteme, 2012.
- 94. Ordóñez Medina, S.A., A. Erath, and K.W. Axhausen. Simulating Urban Transport for a Week Time Horizon Using MATSim . in 3rd Workshop on Time Use Observatory (TUO 3) . 2012. Complex Engineering Systems Institute (ISCI).
- 95. Ordóñez Medina, S.A. Recognizing personalized flexible activity patterns . in 14th International Conference on Travel Behavior Research (IATBR 2015) . 2015. IVT, ETH Zurich.
- 96. Lee, J.H., Travel Behavior Dynamics in Space and Time . 2016, University of California, Santa Barbara.
- 97. Balac, M., M. Janzen, and K.W. Axhausen. Alternative Approach to Scoring in MATSim and how it affects Activity Rescheduling . in 97th Annual Meeting of the Transportation Research Board (TRB 2018) . 2018. TRB Annual Meeting.
- 98. Koppelman, F.S. and C.G. Wilmot, Transferability analysis of disaggregate choice models. Transportation Research Record, 1982. 895 : p. 18-24.
- 99. Yasmin, F., C. Morency, and M.J. Roorda, Assessment of spatial transferability of an activity-based model, TASHA. Transportation Research Part A: Policy and Practice, 2015. 78 : p. 200-213.
- 100. Sikder, S., et al., Spatial transferability of travel forecasting models: a review and synthesis. International Journal of Advances in Engineering Sciences and Applied Mathematics, 2013. 5 (2-3): p. 104-128.
- 101. Arentze, T., et al., Spatial transferability of the Albatross model system: Empirical evidence from two case studies. Transportation Research Record: Journal of the Transportation Research Board, 2002(1805): p. 1-7.
- 102. Inc, P.C., Lake Tahoe Resident and Visitor Model: Model Description and Final Results. 2007.
- 103. Bowman, J.L., et al. Making advanced travel forecasting models affordable through model transferability . in 93rd Transportation Research Board Annual Meeting, Washington DC, USA . 2014.
- 104. Goulias, K.G., et al. Simulator of activities, greenhouse emissions, networks, and travel (SimAGENT) in Southern California . in 91st annual meeting of the Transportation Research Board, Washington, DC . 2012.
- 105. Sivakumar, A., S. Le Vine, and J. Polak. An activity-based travel demand model for London . in European Transport Conference, 2010Association for European Transport . 2010.
- 106. Yasmin, F., C. Morency, and M.J. Roorda, Macro-, meso-, and micro-level validation of an activity-based travel demand model. Transportmetrica A: Transport Science, 2017. 13 (3): p. 222-249.
- 107. Ziemke, D., K. Nagel, and C. Bhat, Integrating CEMDAP and MATSim to increase the transferability of transport demand models. Transportation Research Record: Journal of the Transportation Research Board, 2015(2493): p. 117-125.
- 108. Bowman, J.L. and M. Bradley, Testing Spatial Transferability of Activity-Based Travel Forecasting Models. Transportation Research Record: Journal of the Transportation Research Board, 2017(2669): p. 62-71.
- 109. Linha, H.T., et al., Exploring the Transferability of FEATHERS–An Activity Based Travel Demand Model–For Ho Chi Minh City, Vietnam. Journal of Traffic and Transportation Management, 2019. 1 (2): p. 01-09.
- 110. Shiftan, Y., The advantage of activity-based modelling for air-quality purposes: theory vs practice and future needs. Innovation: The European Journal of Social Science Research, 2000. 13 (1): p. 95-110.
- 111. Shiftan, Y. and J. Suhrbier, The analysis of travel and emission impacts of travel demand management strategies using activity-based models. Transportation, 2002. 29 (2): p. 145-168.
- 112. Beckx, C., et al., An integrated activity-based modelling framework to assess vehicle emissions: approach and application. Environment and Planning B: Planning and Design, 2009. 36 (6): p. 1086-1102.
- 113. Dons, E., et al., Using an activity-based framework to determine effects of a policy measure on population exposure to nitrogen dioxide. Transportation Research Record: Journal of the Transportation Research Board, 2011(2233): p. 72-79.
- 114. Pebesma, E., et al. Uncertainty in exposure to air pollution . in EGU General Assembly Conference Abstracts . 2013.
- 115. Hatzopoulou, M., E. Miller, and B. Santos, Integrating vehicle emission modeling with activity-based travel demand modeling: case study of the Greater Toronto, Canada, Area. Transportation Research Record: Journal of the Transportation Research Board, 2007(2011): p. 29-39.
- 116. Hao, J., M. Hatzopoulou, and E. Miller, Integrating an activity-based travel demand model with dynamic traffic assignment and emission models: Implementation in the Greater Toronto, Canada, area. Transportation Research Record: Journal of the Transportation Research Board, 2010(2176): p. 1-13.
- 117. Hatzopoulou, M., J.Y. Hao, and E.J. Miller, Simulating the impacts of household travel on greenhouse gas emissions, urban air quality, and population exposure. Transportation, 2011. 38 (6): p. 871.
- 118. Vallamsundar, S., et al., Maternal Exposure to Traffic-Related Air Pollution Across Different Microenvironments. Journal of Transport & Health, 2016. 3 (2): p. S72.
- 119. Shabanpour, R., et al., Investigating the applicability of ADAPTS activity-based model in air quality analysis. Travel Behaviour and Society, 2017.
- 120. Wu, Y. and G. Song, The Impact of Activity-Based Mobility Pattern on Assessing Fine-Grained Traffic-Induced Air Pollution Exposure. International journal of environmental research and public health, 2019. 16 (18): p. 3291.
- 121. Shliselberg, R. and M. Givoni, Motility as a policy objective. Transport reviews, 2018. 38 (3): p. 279-297.
- 122. Abou-Zeid, M. and M. Ben-Akiva, Well-being and activity-based models. Transportation, 2012. 39 (6): p. 1189-1207.
- 123. Stanley, J.K., et al., Mobility, social exclusion and well-being: Exploring the links. Transportation research part A: policy and practice, 2011. 45 (8): p. 789-801.
- 124. Hensher, D.A., Measurement of the valuation of travel time savings. Journal of Transport Economics and Policy (JTEP), 2001. 35 (1): p. 71-98.
- 125. Metz, D., The myth of travel time saving. Transport reviews, 2008. 28 (3): p. 321-336.
- 126. El-Geneidy, A.M. and D.M. Levinson, Access to destinations: Development of accessibility measures. 2006.
- 127. de Lima, I.V., et al., Dynamic Modeling of Activity Happiness: An Investigation of the Intra-activity Hedonic Treadmill , in Quality of Life and Daily Travel . 2018, Springer. p. 95-118.
- 128. Guo, W., Y. Chai, and M.-P. Kwan, Travel-related exposure to air pollution and its socio-environmental inequalities: Evidence from a week-long GPS-based travel diary dataset , in Spatiotemporal Analysis of Air Pollution and Its Application in Public Health . 2020, Elsevier. p. 293-309.
- 129. Castiglione, J., et al. Building an Integrated Activity-Based and Dynamic Network Assignment Model . in 3rd Transportation Research Board Conference on Innovations in Travel Modeling, Tempe, Ariz . 2010.
- 130. Bekhor, S., C. Dobler, and K. Axhausen, Integration of activity-based and agent-based models: case of Tel Aviv, Israel. Transportation Research Record: Journal of the Transportation Research Board, 2011(2255): p. 38-47.
- 131. Lin, D.-Y., et al., Evacuation planning using the integrated system of activity-based modeling and dynamic traffic assignment. Transportation Research Record: Journal of the Transportation Research Board, 2009(2132): p. 69-77.
- 132. Liu, P., et al., Dynamic activity-travel assignment in multi-state supernetworks. Transportation Research Part B: Methodological, 2015. 81 : p. 656-671.
- 133. Pendyala, R.M., et al., Integrated Land Use–Transport Model System with Dynamic Time-Dependent Activity–Travel Microsimulation. Transportation Research Record, 2012. 2303 (1): p. 19-27.
- 134. Pendyala, R.M., et al., Paradigms for integrated modeling of activity-travel demand and network dynamics in an era of dynamic mobility management . 2017.
- 135. Zockaie, A., et al., Activity-Based Model with Dynamic Traffic Assignment and Consideration of Heterogeneous User Preferences and Reliability Valuation: Application to Toll Revenue Forecasting in Chicago, Illinois. Transportation Research Record: Journal of the Transportation Research Board, 2015(2493): p. 78-87.
- 136. Rieser, M., et al., Agent-oriented coupling of activity-based demand generation with multiagent traffic simulation. Transportation Research Record, 2007. 2021 (1): p. 10-17.
- 137. Hao, J.Y., M. Hatzopoulou, and E.J. Miller, Integrating an activity-based travel demand model with dynamic traffic assignment and emission models: Implementation in the Greater Toronto, Canada, area. Transportation Research Record, 2010. 2176 (1): p. 1-13.
- 138. Lin, D.-Y., et al., Integration of activity-based modeling and dynamic traffic assignment. Transportation Research Record, 2008. 2076 (1): p. 52-61.
- 139. Hadi, M., et al., Dynamic, Integrated Model System: Jacksonville-Area Application . 2014.
- 140. Javanmardi, M., J. Auld, and K. Mohammadian. Integration of TRANSIMS with the ADAPTS Activity-based Model . in Fourth TRB Conference on Innovations in Travel Modeling (ITM), Tampa, FL . 2011.
- 141. Zockaie, A., et al., Activity-based model with dynamic traffic assignment and consideration of heterogeneous user preferences and reliability valuation: application to toll revenue forecasting in Chicago, Illinois. Transportation Research Record, 2015. 2493 (1): p. 78-87.
- 142. Vovsha, P., et al. Integrated model of travel demand and network simulation . in Proceedings of the 6th Conference on Innovations in Travel Modeling (ITM), TRB, Denver, CO . 2016.
- 143. Cipriani, E., et al., Integration between activity-based demand models and multimodal assignment: some empirical evidences. Case Studies on Transport Policy, 2020.
- 144. Adnan, M., et al., Integrated agent-based microsimulation framework for examining impacts of mobility-oriented policies. Personal and Ubiquitous Computing, 2020: p. 1-13.
- 145. Baghestani, A., et al., Evaluating the Traffic and Emissions Impacts of Congestion Pricing in New York City. Sustainability, 2020. 12 (9): p. 3655.
- 146. Cepolina, E.M. and A. Farina, A new shared vehicle system for urban areas. Transportation Research Part C: Emerging Technologies, 2012. 21 (1): p. 230-243.
- 147. Ciari, F., M. Balac, and K.W. Axhausen, Modeling Carsharing with the Agent-Based Simulation MATSim: State of the Art, Applications, and Future Developments. Transportation Research Record: Journal of the Transportation Research Board, 2016(2564): p. 14-20.
- 148. Balac, M., F. Ciari, and K.W. Axhausen, Modeling the impact of parking price policy on free-floating carsharing: Case study for Zurich, Switzerland. Transportation Research Part C: Emerging Technologies, 2017. 77 : p. 207-225.
- 149. Heilig, M., et al., Implementation of free-floating and station-based carsharing in an agent-based travel demand model. Travel Behaviour and Society, 2018. 12 : p. 151-158.
- 150. Li, Q., et al., Incorporating free-floating car-sharing into an activity-based dynamic user equilibrium model: A demand-side model. Transportation Research Part B: Methodological, 2018. 107 : p. 102-123.
- 151. Choo, S., P.L. Mokhtarian, and I. Salomon, Does telecommuting reduce vehicle-miles traveled? An aggregate time series analysis for the US. Transportation, 2005. 32 (1): p. 37-64.
- 152. Kim, S.-N., Is telecommuting sustainable? An alternative approach to estimating the impact of home-based telecommuting on household travel. International Journal of Sustainable Transportation, 2017. 11 (2): p. 72-85.
- 153. Zhu, P. and S.G. Mason, The impact of telecommuting on personal vehicle usage and environmental sustainability. International Journal of Environmental Science and Technology, 2014. 11 (8): p. 2185-2200.
- 154. Paleti, R. and I. Vukovic, Telecommuting and Its Impact on Activity–Time Use Patterns of Dual-Earner Households. Transportation Research Record: Journal of the Transportation Research Board, 2017(2658): p. 17-25.
- 155. Helminen, V. and M. Ristimäki, Relationships between commuting distance, frequency and telework in Finland. Journal of Transport Geography, 2007. 15 (5): p. 331-342.
- 156. Pouri, Y. and C. Bhat, On Modeling Choice and Frequency of Home-Based Telecommunting. Transportation Research Record: Journal of the Transportation Research Board, 2003(1858): p. 55-60.
- 157. Sener, I.N. and C.R. Bhat, A copula-based sample selection model of telecommuting choice and frequency. Environment and Planning A, 2011. 43 (1): p. 126-145.
- 158. Shabanpour, R., et al., Analysis of telecommuting behavior and impacts on travel demand and the environment. Transportation Research Part D: Transport and Environment, 2018. 62 : p. 563-576.
- 159. Pirdavani, A., et al., Traffic Safety Implications of Travel Demand Management Policies: The Cases of Teleworking and Fuel Cost Increase , in Transportation Systems and Engineering: Concepts, Methodologies, Tools, and Applications . 2015, IGI Global. p. 1082-1107.
- 160. Zhang, L., et al., Integrating an agent-based travel behavior model with large-scale microscopic traffic simulation for corridor-level and subarea transportation operations and planning applications. Journal of Urban Planning and Development, 2013. 139 (2): p. 94-103.
- 161. Yang, D., INTEGRATING ACTIVITY-BASED TRAVEL DEMAND AND DYNAMIC TRAFFIC ASSIGNMENT MODEL: A BEHAVIORAL USER EQUILIBRIUM APPROACH . 2018.
- 162. Frank, L.D., et al., Many pathways from land use to health: associations between neighborhood walkability and active transportation, body mass index, and air quality. Journal of the American planning Association, 2006. 72 (1): p. 75-87.
© 2020 The Author(s). Licensee IntechOpen. This chapter is distributed under the terms of the Creative Commons Attribution 3.0 License , which permits unrestricted use, distribution, and reproduction in any medium, provided the original work is properly cited.
Continue reading from the same book
Edited by Stefano de Luca
Published: 28 July 2021
By Joan Harvey
452 downloads
By Carlos Hugo Criado del Valle and Parichehr Scharif...
398 downloads
By Francesco Viti, Marco Rinaldi and Georgios Laskari...
559 downloads
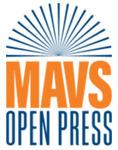
Want to create or adapt books like this? Learn more about how Pressbooks supports open publishing practices.
Part III: Travel Demand Modeling
13 Last Step of Four Step Modeling (Trip Assignment Models)
Chapter overview.
Chapter 13 presents trip assignment, the last step of the Four-Step travel demand Model (FSM). This step determines which paths travelers choose for moving between each pair of zones. Additionally, this step can yield numerous results, such as traffic volumes in different transportation corridors, the patterns of vehicular movements, total vehicle miles traveled (VMT) and vehicle travel time (VTT) in the network, and zone-to-zone travel costs. Identification of the heavily congested links is crucial for transportation planning and engineering practitioners. This chapter begins with some fundamental concepts, such as the link cost functions. Next, it presents some common and useful trip assignment methods with relevant examples. The methods covered in this chapter include all-or-nothing (AON), user equilibrium (UE), system optimum (SO), feedback loop between distribution and assignment (LDA), incremental increase assignment, capacity restrained assignment, and stochastic user equilibrium assignment.
Learning Objectives
- Describe the reasons for performing trip assignment models in FSM and relate these models’ foundation through the cost-function concept.
- Compare static and dynamic trip assignment models and infer the appropriateness of each model for different situations.
- Explain Wardrop principles and relate them to traffic assignment algorithms.
- Complete simple network traffic assignment models using static models such as the all-or-nothing and user equilibrium models.
- Solve modal split analyses manually for small samples using the discrete choice modeling framework and multinominal logit models.
Introduction
In this chapter, we continue the discussion about FSM and elaborate on different methods of traffic assignment, the last step in the FSM model after trip generation, trip distribution, and modal split. The traffic assignment step, which is also called route assignment or route choice , simulates the choice of route selection from a set of alternatives between the origin and the destination zones (Levinson et al., 2014). The first three FSM steps determine the number of trips produced between each zone and the proportion completed by different transportation modes. The purpose of the final step is to determine the routes or links in the study area that are likely to be used. For example, when updating a Regional Transportation Plan (RTP), traffic assignment is helpful in determining how much shift or diversion in daily traffic happens with the introduction an additional transit line or extension a highway corridor (Levinson et al., 2014). The output from the last step can provide modelers with numerous valuable results. By analyzing the results, the planner can gain insight into the strengths and weaknesses of different transportation plans. The results of trip assignment analysis can be:
- The traffic flows in the transportation system and the pattern of vehicular movements.
- The volume of traffic on network links.
- Travel costs between trip origins and destinations (O-D).
- Aggregated network metrics such as total vehicle flow, vehicle miles traveled (VMT) , and vehicle travel time (VTT).
- Zone-to-zone travel costs (travel time) for a given level of demand.
- Modeled link flows highlighting congested corridors.
- Analysis of turning movements for future intersection design.
- Determining the Origin-Destination (O-D) pairs using a specific link or path.
- Simulation of the individual choice for each pair of origins and destinations (Mathew & Rao, 2006).
Link Performance Function
Building a link performance function is one of the most important and fundamental concepts of the traffic assignment process. This function is usually used for estimating travel time, travel cost, and speed on the network based on the relationship between speed and travel flow. While this function can take different forms, such as linear, polynomial , exponential , and hyperbolic , one of the most common functions is the link performance function which represents generalized travel costs (United States Bureau of Public Roads, 1964). This equation estimates travel time on a free-flow road (travel with speed limit) adding a function that exponentially increases travel time as the road gets more congested. The road volume-to-capacity ratio can represent congestion (Meyer, 2016).
While transportation planners now recognize that intersection delays contribute to link delays, the following sections will focus on the traditional function. Equation (1) is the most common and general formula for the link performance function.
![Rendered by QuickLaTeX.com t=t_o[1+\alpha\left(\frac{x}{k}\right)\beta]](https://uta.pressbooks.pub/app/uploads/quicklatex/quicklatex.com-b0069e9cc96ab2ee3dd2a513568bed91_l3.png)
- t and x are the travel time and vehicle flow;
- t 0 is the link free flow travel time;
- k is the link capacity;
- α and β are parameters for specific type of links and calibrated using the field data. In the absence of any field data, it is usually assumed = 0.15, and β= 4.0.
α and β are the coefficients for this formula and can take different values (model parameters). However, most studies and planning practices use the same value for them. These values can be locally calibrated for the most efficient results.
Figure 13.1 demonstrates capacity as the relationship between flow and travel time. In this plot, the travel time remains constant as vehicle volumes increase until the turning point , which indicates that the link’s volume is approaching its capacity.
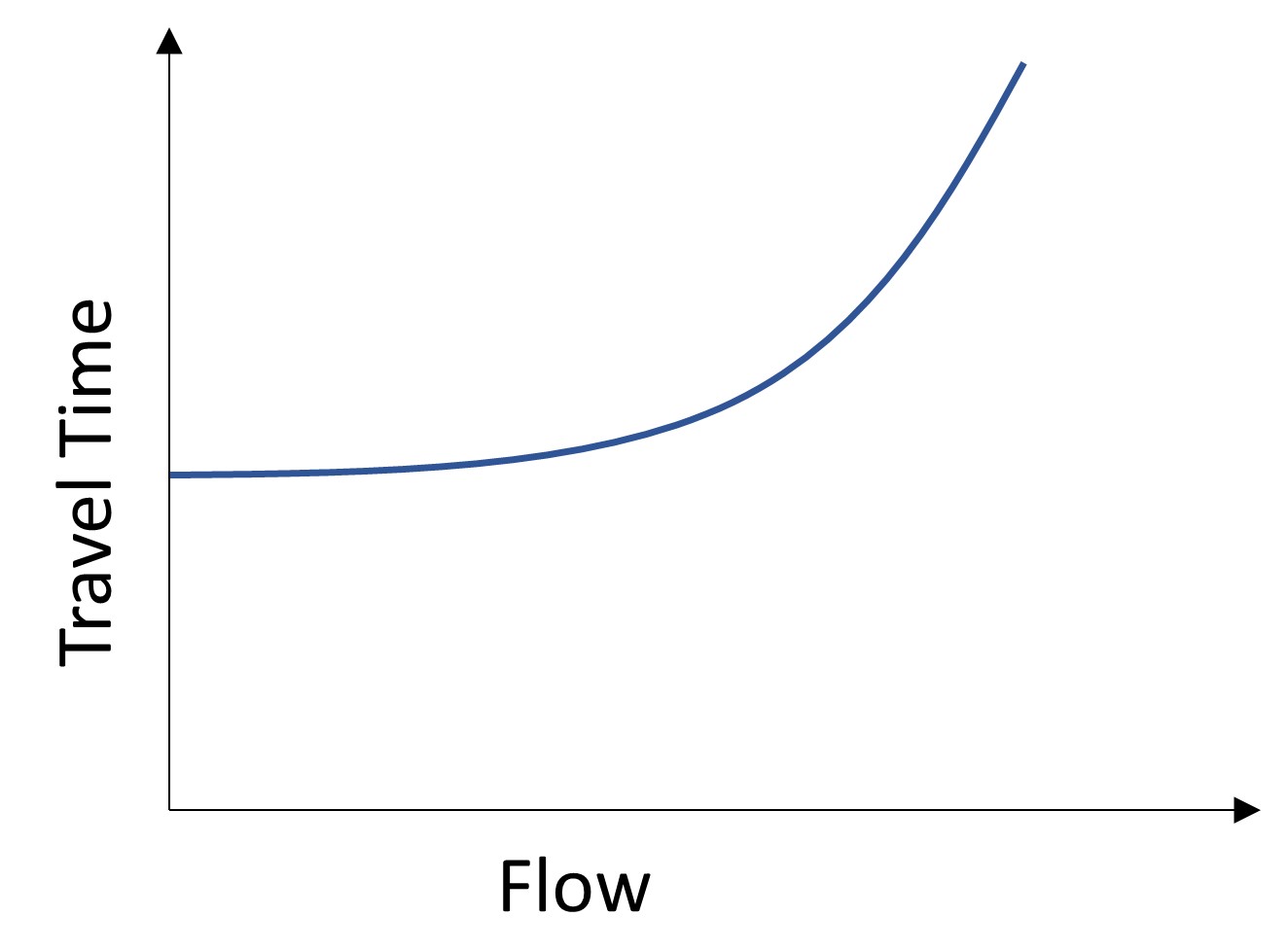
The following example shows how the link performance function helps us to determine the travel time according to flow and capacity.
Performance Function Example
Assume the traffic volume on a path between zone i and j was 525. The travel time recorded on this path is 15 minutes. If the capacity of this path would be 550, then calculate the new travel time for future iteration of the model.
Based on the link performance function, we have:
Now we have to plug in the numbers into the formula to determine the new travel time:
![Rendered by QuickLaTeX.com t=15[1+\0.15\left(\frac{525}{550}\right)\4]=16.86](https://uta.pressbooks.pub/app/uploads/quicklatex/quicklatex.com-4815be46eaf8d0307c939444a157a3ea_l3.png)
Traffic Assignment Models
Typically, traffic assignment is calculated for private cars and transit systems independently. Recall that the impedance function differs for drivers and riders, and thus simulating utility maximization behavior should be approached differently. For public transit assignment, variables such as fare, stop or transfer, waiting time, and trip times define the utility (equilibrium) (Sheffi, 1985). For private car assignment, however, in some cases, the two networks are related when public buses share highways with cars, and congestion can also affect the performance.
Typically, private car traffic assignment models the path choice of trip makers using:
- algorithms like all-or-nothing
- user equilibrium
- system optimum assignment
Of the assignment models listed above, user equilibrium is widely adopted in the U.S. (Meyer, 2016). User equilibrium relies on the premise that travelers aim to minimize their travel costs. This algorithm achieves equilibrium when no user can decrease their travel time or cost by altering their travel path.
- incremental
- capacity-restrained
- iterative feedback loop
- Stochastic user equilibrium assignment
- Dynamic traffic assignment
All-or-nothing Model
Through the all-or-nothing (AON) assignment, it is assumed that the impedance of a road or path between each origin and destination is constant and equal to the free-flow level of service. This means that the traffic time is not affected by the traffic flow on the path. The only logic behind this model is that each traveler uses the shortest path from his or her origin to the destination, and no vehicle is assigned to other paths (Hui, 2014). This method is called the all-or-nothing assignment model and is the simplest one among all assignment models. This method is also called the 0-1 assignment model, and its advantage is its simple procedure and calculation. The assumptions of this method are:
- Congestion does not affect travel time or cost, meaning that no matter how much traffic is loaded on the route, congestion does not take place.
- Since the method assigns one route to any travel between each pair of OD, all travelers traveling from a particular zone to another particular zone choose the same route (Hui, 2014).
To run the AON model, the following process can be followed:
- Step 0: Initialization. Use free flow travel costs Ca=Ca(0) , for each link a on the empty network. Ɐ
- Step 1: Path finding. Find the shortest path P for each zonal pair.
- Step 2: Path flows assigning. Assign both passenger trips (hppod) and freight trips (hfpod) in PCEs from zonal o to d to path P.
- Step 3: Link flows computing. Sum the flows on all paths going through a link as total flows of this link.
Example 2 illustrates the above-mentioned process for the AON model

All-or-nothing Example
Table 13.1 shows a trip distribution matrix with 4 zones. Using the travel costs between each pair of them shown in Figure 13.2, assign the traffic to the network. Load the vehicle trips from the trip distribution table shown below using the AON technique. After assigning the traffic, illustrate the links and the traffic volume on each on them.
Table 13.1 Trip Distribution Results.
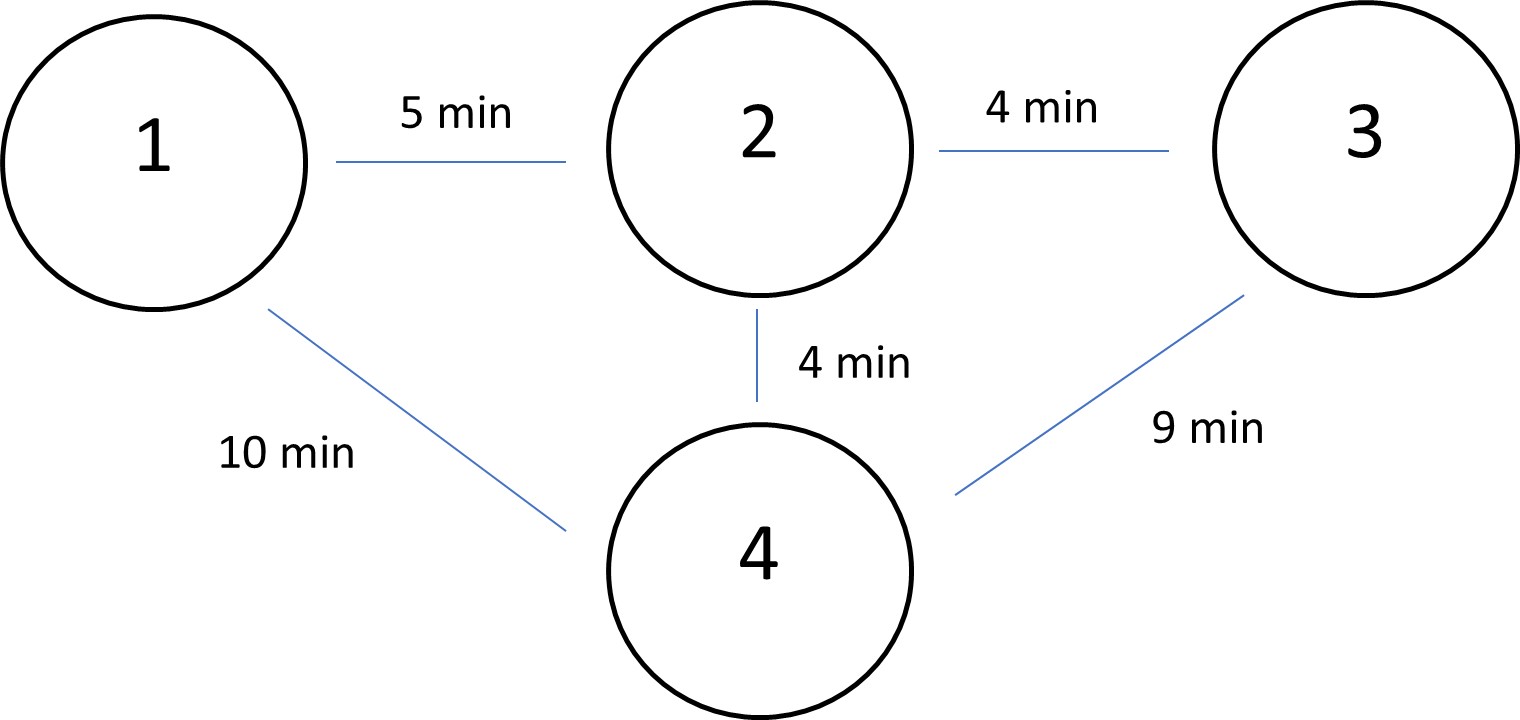
To solve this problem, we need to find the shortest path among all alternatives for each pair of zones. The result of this procedure would be 10 routes in total, each of which bears a specific amount of travels. For instance, the shortest path between zone 1 and 2 is the straight line with 5 min travel time. All other routes like 1 to 4 to 2 or 1 to 4 to 3 to 2 would be empty from travelers going from zone 1 to zone 2. The results are shown in Table 13.2.
As you can see, some of the routes remained unused. This is because in all-or-nothing if a route has longer travel time or higher costs, then it is assumed it would not be used at all.
User Equilibrium
The next method for traffic assignment is called User Equilibrium (UE). The rule or algorithm is adapted from the well-known Wardrop equilibrium (1952) conditions (Correa & Stier-Moses, 2011). In this algorithm, it is assumed that travelers will always choose the shortest path, and equilibrium conditions are realized when no traveler is able to decrease their travel impedance by changing paths (Levinson et al., 2014).
As we discussed, the UE method is based on the first principle of Wardrop : “for each origin- destination (OD) pair, with UE, the travel time on all used paths is equal and less than or equally to the travel time that would be experienced by a single vehicle on any unused path”( Jeihani Koohbanani, 2004, p. 10). The mathematical format of this principle is shown in equation (3):

For a given OD pair, the UE condition can be expressed in equation (3):

This model assumes that all paths have equal travel time. Additionally, the model includes the following general assumptions:
- The users possess all the knowledge needed about different paths.
- The users have perfect knowledge of the path cost.
- Travel time in a route is subject to change only by the cost flow function of that route.
- Travel times increases as we load travel into the network (Mathew & Rao, 2006).
Hence, the UE assignment comes to an optimization problem that can be formulated using equation (4):

k is the path x a equilibrium flow in link a t a travel time on link a f k rs flow on path connecting OD pairs q rs trip rate between and δ a, k rs is constraint function defined as 1 if link a belongs to path k and 0 otherwise
Example 3 shows how the UE method can be applied for the traffic assignment step. This example is a very simple network consisting of two zones with two possible paths between them.
UE Example
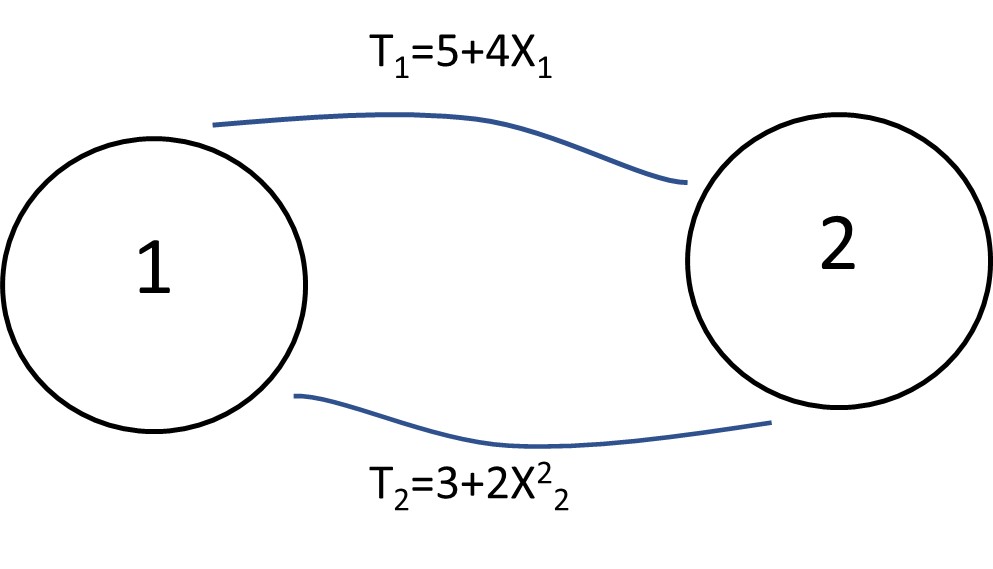
In this example, t 1 and t 2 are travel times measured by min on each route, and x 1 and x 2 are traffic flows on each route measured by (Veh/Hour).
Using the UE method, assign 4,500 Veh/Hour to the network and calculate travel time on each route after assignment, traffic volume, and system total travel time.
According to the information provided, total flow (X 1 +X 2 ) is equal to 4,500 (4.5).
First, we need to check, with all traffic assigned to one route, whether that route is still the shortest path. Thus we have:
T 1 (4.5)=23min
T 2 (0)=3min
if all traffic is assigned to route 2:
T 1 (0)=3min
T 2 (4.5)=43.5 min
Step 2: Wardrope equilibrium rule: t 1 =t 2 5+4x 1 =3+ 2x 2 2 and we have x 1 =4.5-x 2
Now the equilibrium equation can be written as: 6 + 4(4.5 − x2)=4+ x222
x 1 = 4.5 − x 2 = 1.58
Now the updated average travel times are: t 1 =5+4(1.58)=11.3min and T 2 =3+2(2.92)2=20.05min
Now the total system travel time is:
Z(x)=X 1 T 1 (X 1 )+X 2 T 2 (X 2 )=2920 veh/hr(11.32)+1585 veh/hr(20.05)=33054+31779=64833 min
System Optimum Assignment
One traffic assignment model is similar to the previous one and is called system optimum (SO). The second principle of the Wardrop defines the model’s logic. Based on this principle, drivers’ rationale for choosing a path is to minimize total system costs with one another to minimize total system travel time (Mathew & Rao, 2006). Using the SO traffic assignment, one can solve various problems, such as optimizing the departure time for a single commuting route, minimizing the total travel time from multiple origins to a single destination, or minimizing travel time in stochastic time-dependent O-D flows from several origins to a single destination ( Jeihani & Koohbanani, 2004).
One other traffic assignment model similar to the previous one is called system optimum (SO) in which the second principle of the Wardrop defines the logic of the model. Based on this principle, drivers’ rationale for choosing a path is to minimize total system costs with one another in order to minimize total system travel time (Mathew & Rao, 2006). Using the SO traffic assignment, problems like optimizing departure time for a single commuting route, minimizing total travels from multiple origins to one destination, or minimizing travel time in stochastic time-dependent OD flows from several origins to a single destination can be solved (Jeihani Koohbanani, 2004).
The basic mathematical formula for this model that satisfies the principle of the model is shown in equation (5):

In example 4, we will use the same network we described in the UE example in order to compare the results for the two models.
In that simple two-zone network, we had:
T 1 =5+4X 1 T2=3+2X 2 2
Now, based on the principle of the model we have:
Z(x)=x 1 t 1 (x 1 )+x 2 t 2 (x 2 )
Z(x)=x 1 (5+4x 1 )+x 2 (3+2x 2 2 )
Z(x)=5x 1 +4x 1 2 +3x 2 +2x 2 3
From the flow conservation. we have: x 1 +x 2 =4.5 x 1 =4.5-x 2
Z(x)=5(4.5-x 2 )+4(4.5-x 2 )2+4x 2 +x 2 3
Z(x)=x 3 2 +4x 2 2 -27x 2 +103.5
In order to minimize the above equation, we have to take derivatives and equate it to zero. After doing the calculations, we have:
Based on our finding, the system travel time would be:
T 1 =5+4*1.94=12.76min T 2 =3+ 2(2.56)2=10.52 min
And the total travel time of the system would be:
Z(x)=X 1 T 1 (X 1 )+X 2 T 2 (X 2 )=1940 veh/hr(12.76)+2560 veh/hr(10.52)=24754+26931=51685 min
Incremental Increase model
Incremental increase is based on the logic of the AON model and models a process designed with multiple steps. In each step or level, a fraction of the total traffic volume is assigned, and travel time is calculated based on the allocated traffic volume. Through this incremental addition of traffic, the travel time of each route in step (n) is the updated travel time from the previous step (n-1) (Rojo, 2020).
The steps for the incremental increase traffic assignment model are:
- Finding the shortest path between each pair of O-Ds (Origin Destination).
- Assigning a portion of the trips according to the matrix (usually 40, 30, 20 and 10 percent to the shortest path).
- Updating the travel time after each iteration (each incremental increase).
- Continuing until all trips are assigned.
- Summing the results.
The example below illustrates the implementation process of this method.
A hypothetical network accommodates two zones with three possible links between them. Perform an incremental increase traffic assignment model for assigning 200 trips between the two zones with increments of: 30%, 30%, 20%, 20%. (The capacity is 50 trips.)
Incremental Increase Example
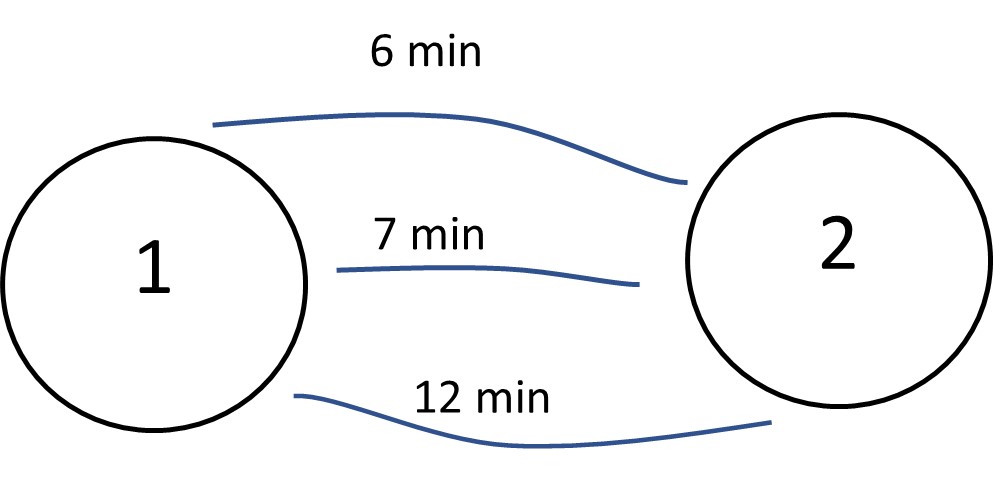
Step 1 (first iteration): Using the method of AON, we now assign the flow to the network using the function below:
![Rendered by QuickLaTeX.com t=to[1+\alpha\left(\frac{x}{k}\right)\beta]](https://uta.pressbooks.pub/app/uploads/quicklatex/quicklatex.com-e6ae32391e4ce2864e22b32f4ce8036d_l3.png)
Since the first route has the shortest travel time, the first 30% of the trips will be assigned to route 1. The updated travel time for this path would be:
![Rendered by QuickLaTeX.com t=6\left[1+0.15\left(\frac{60}{50}\right)4\right]=7.86](https://uta.pressbooks.pub/app/uploads/quicklatex/quicklatex.com-0efdc05f3641753417dd22056d786d27_l3.png)
And the remaining route will be empty, and thus their travel times are unchanged.
Step 2 (second iteration): Now, we can see that the second route has the shortest travel time, with 30% of the trips being assigned to this route, and the new travel time would be:
![Rendered by QuickLaTeX.com t=7\left[1+0.15\left(\frac{60}{50}\right)4\right]=9.17](https://uta.pressbooks.pub/app/uploads/quicklatex/quicklatex.com-ee2cb7dd6e67408c0fd206917565f183_l3.png)
Step 3 (third iteration): In the third step, the 20% of the remaining trips will be assigned to the shortest path, which in this case is the first route again. The updated travel time for this route is:
![Rendered by QuickLaTeX.com t=7.86\left[1+0.15\left(\frac{40}{50}\right)4\right]=8.34](https://uta.pressbooks.pub/app/uploads/quicklatex/quicklatex.com-edaab16adde9967db55782f11d92a0e6_l3.png)
Step 4 (fourth iteration): In the last iteration, the remaining 10% would be assigned to first route, and the time is:
![Rendered by QuickLaTeX.com t=8.34\left[1+0.15\left(\frac{40}{50}\right)4\right]=8.85](https://uta.pressbooks.pub/app/uploads/quicklatex/quicklatex.com-95a27b24b4ec1169c1b611960683dbfe_l3.png)
Finally, we can see that route 1 has a total of 140 trips with a 8.85 travel time, the second route has a total of 60 trips with a 9.17 travel time, and the third route was never used.
Capacity Restraint Assignment
So far, all the presented algorithms or rules have considered the model’s link capacity. The flow is assigned to a link based on travel time as the only factor. In this model, after each iteration, the total number of trips is compared with the capacity to observe how much increase in travel time was realized by the added volume. In this model, the iteration stops if the added volume in step (n) does not change the travel time updated in step (n-1). With the incorporation of such a constraint, the cost or performance function would be different from the cost functions discussed in previous algorithms (Mathew & Rao, 2006). Figure 13.6 visualizes the relationship between flow and travel time with a capacity constraint.
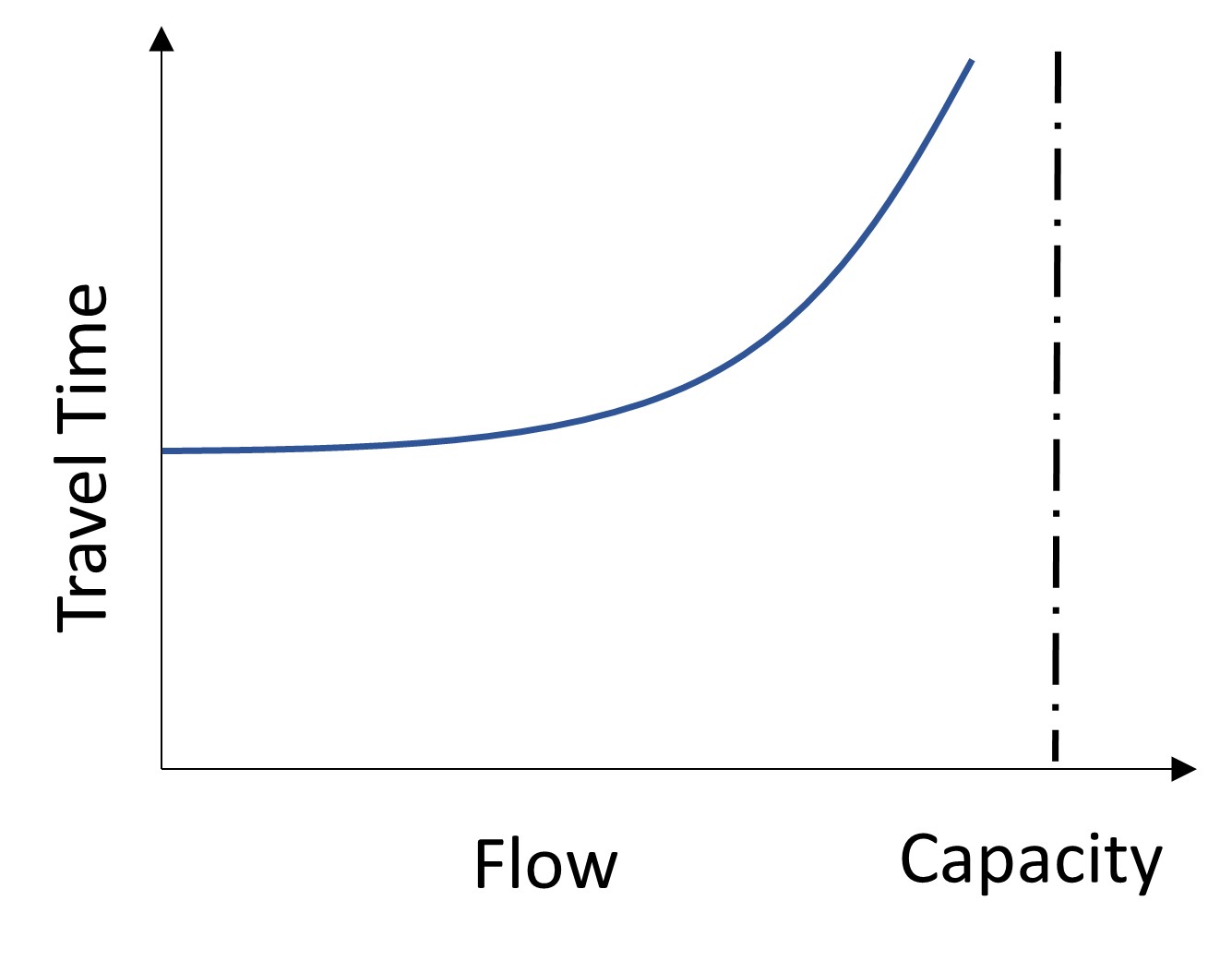
Based on this capacity constraint specific to each link, the α, β can be readjusted for different links such as highways, freeways, and other roads.
Feedback Loop Model (Combined Traffic Assignment and Trip Distribution)
The feedback loop model defines an interaction between the trip distribution route choice step with several iterations. The model allows travelers to change their destination if a route is congested. For example, the feedback loop models that the traveler has a choice of similar destinations, such as shopping malls, in the area. In other words, in a real-world situation, travelers usually simultaneously decide about their travel characteristics (Qasim, 2012).
The chart below shows how the combination of these two modes can take place:
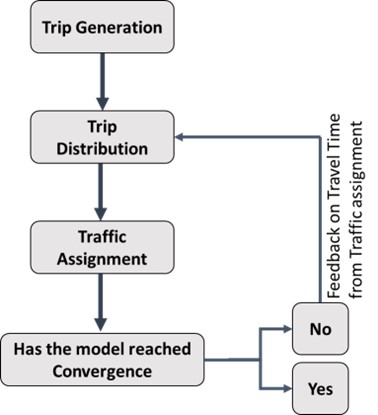
Equation (6), shown below for this model, ensures convergence at the end of the model is:

where C a (t) is the same as previous
P a , is total personal trip flows on link a,
f a ; is total freight trip flows on link a,
T od is the total flow from node o to node d,
p od is personal trip from node o to node d,
F od is freight trip from node o to node d,
ζ is a parameter estimated from empirical data,
K is a parameter depending on the type of gravity model used to calculate T od , Evans (1976) proved that K’ equals to 1 for distribution using doubly constrained gravity model and it equals to 1 plus attractiveness for distribution using singly constrained model. Florian et al. (1975) ignored K for distribution using a doubly constrained gravity model because it is a constant.
Stochastic User Equilibrium Traffic Assignment
Stochastic user equilibrium traffic assignment is a sophisticated and more realistic model in which the level of uncertainty regarding which link should be used based on a measurement of utility function is introduced. This model performs a discrete choice analysis through a logistic model. Based on the first Wardrop principle, this model assumes that all drivers perceive the costs of traveling in each link identically and choose the route with minimum cost. In stochastic UE, however, the model allows different individuals to have different perceptions about the costs, and thus, they may choose non-minimum cost routes (Mathew & Rao, 2006). In this model, flow is assigned to all links from the beginning, unlike previous models, which is closer to reality. The probability of using each path is calculated with the following logit formula shown in equation (7):
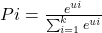
P i is the probability of using path i
U i is the utility function for path i
In the following, an example of a simple network is presented.
Stochastic User Equilibrium Example
There is a flow of 200 trips between two points and their possible path, each of which has a travel time specified in Figure 13.7.
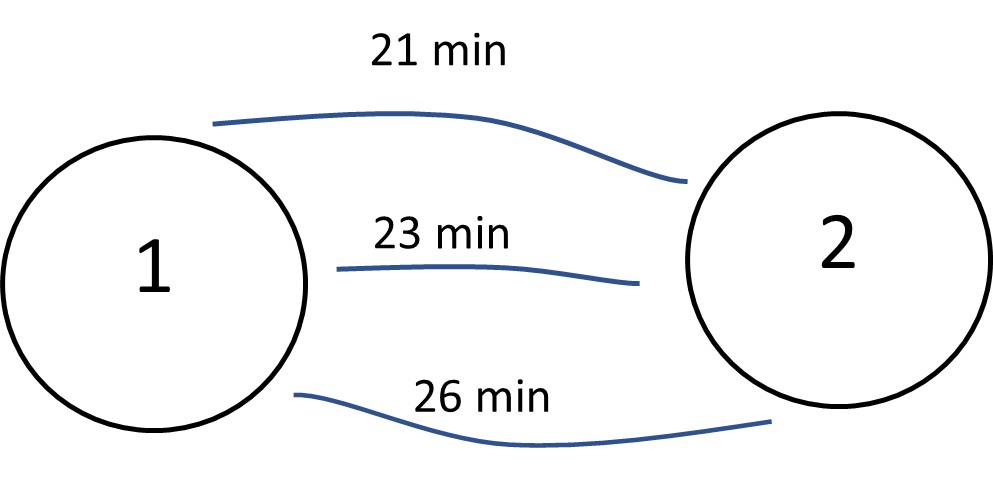
Using the mentioned logit formula for these paths, we have:

Based on the calculated probabilities, the distribution of the traffic flow would be:
Q 1 =175 trips
Q 2 =24 trips
Q 3 =1 trips
Dynamic Traffic Assignment
Recall the first Wardrop principle, in which travelers are believed to choose their routes with the minimum cost. Dynamic traffic assignment is based on the same rule, but the difference is that delays result from congestion. In this way, not only travelers’ route choice affects the network’s level of service, but also the network’s level of service affects travelers’ choice. However, it is not theoretically proven that an equilibrium would result under such conditions (Mathew & Rao, 2006).
Today, various algorithms are developed to solve traffic assignment problems. In any urban transportation system, travelers’ route choice and different links’ level of service have a dynamic feedback loop and affect each other simultaneously. However, a lot of these rules are not present in the models presented here. In real world cases, there can be more than thousands of nodes and links in the network, and therefore more sensitivity to dynamic changes is required for a realistic traffic assignment (Meyer, 2016). Also, the travel demand model applies a linear sequence of the four steps, which is unlike reality. Additionally, travelers may have only a limited knowledge of all possible paths, modes, and opportunities and may not make rational decisions.
In this last chapter of landuse/transportation modeling book, we reviewed the basic concepts and principles of traffic assignment models as the last step in travel demand modeling. Modeling the route choice and other components of travel behavior and demand for transportation proven to be very challenging and can incorporate multiple factors. For instance, going from AON to incremental increase assignment, we factor in the capacity and volume (and resulting delays) relationship in the assignment to make more realistic models. Multiple-time-period assignments for multiple classes, separate specification of facilities like high-occupancy vehicle (HOV) and high-occupancy toll (HOT) lanes; and, independent transit assignment using congested highway travel times to estimate a bus ridership assignment, are some of the new extensions and variation of algorithms that take into account more realities within transportation network. A new prospect in traffic assignment models that adds several capabilities for such efforts is emergence of ITS such as data that can be collected from connected vehicles or autonomous vehicles. Using these data, perceived utility or impedances of different modes or infrastructure from individuals perspective can be modeled accurately, leading to more accurate assignment models, which are crucial planning studies such as growth and land use control efforts, environmental studies, transportation economies, etc.
Route choice is the process of choosing a certain path for a trip from a very large choice sets.
Regional Transportation Plan is long term planning document for a region’s transportation usually updated every five years.
Vehicles (VMT) is the aggregate number of miles deriven from in an area in particular time of day.
- Total vehicle travel time is the aggregate amount of time spent in transportation usually in minutes.
Link performance function is function used for estimating travel time, travel cost, and speed on the network based on the relationship between speed and travel flow.
Hyperbolic function is a function used for linear differential equations like calculating distances and angels in hyperbolic geometry.
Free-flow road is situation where vehicles can travel with the maximum allowed travel speed.
- Algorithms like all-or-nothing an assignment model where we assume that the impedance of a road or path between each origin and destination is constant and is equal to free-flow level of service, meaning that the traffic time is not affected by the traffic flow on the path.
Capacity-restrained is a model which takes into account the capacity of a road compared to volume and updates travel times.
User equilibrium is a traffic assignment model where we assume that travelers will always choose the shortest path and equilibrium condition would be realized when no traveler is able to decrease their travel impedance by changing paths.
System optimum assignment is an assignment model based on the principle that drivers’ rationale for choosing a path is to minimize total system costs with one another in order to minimize total system travel time.
- Static user-equilibrium assignment algorithm is an iterative traffic assignment process which assumes that travelers chooses the travel path with minimum travel time subject to constraints.
- Iterative feedback loop is a model that iterates between trip distribution and route choice step based on the rational that if a path gets too congested, the travel may alter travel destination.
First principle of Wardrop is the assumption that for each origin-destination (OD) pair, with UE, the travel time on all used paths is equal and less than or equally to the travel time that would be experienced by a single vehicle on any unused path.
System optimum (SO) is a condition in trip assignment model where total travel time for the whole area is at a minimum.
- Stochastic time-dependent OD is a modeling framework where generation and distribution of trips are randomly assigned to the area.
Incremental increase is AON-based model with multiple steps in each of which, a fraction of the total traffic volume is assigned, and travel time is calculated based on the allocated traffic volume.
Stochastic user equilibrium traffic assignment employs a probability distribution function that controls for uncertainties when drivers compare alternative routes and make decisions.
Dynamic traffic assignment is a model based on Wardrop first principle in which delays resulted from congestion is incorporated in the algorithm.
Key Takeaways
In this chapter, we covered:
- Traffic assignment is the last step of FSM, and the link cost function is a fundamental concept for traffic assignment.
- Different static and dynamic assignments and how to perform them using a simplistic transportation network.
- Incorporating stochastic decision-making about route choice and how to solve assignment problems with regard to this feature.
Prep/quiz/assessments
- Explain what the link performance function is in trip assignment models and how it is related to link capacity.
- Name a few static and dynamic traffic assignment models and discuss how different their rules or algorithms are.
- How does stochastic decision-making on route choice affect the transportation level of service, and how it is incorporated into traffic assignment problems?
- Name one extension of the all-or-nothing assignment model and explain how this extension improves the model results.
Correa, J.R., & Stier-Moses, N.E.(2010).Wardrope equilibria. In J.J. Cochran( Ed.), Wiley encyclopedia of operations research and management science (pp.1–12). Hoboken, NJ: John Wiley & Sons. http://dii.uchile.cl/~jcorrea/papers/Chapters/CS2010.pdf
Hui, C. (2014). Application study of all-or-nothing assignment method for determination of logistic transport route in urban planning. Computer Modelling & New Technologies , 18 , 932–937. http://www.cmnt.lv/upload-files/ns_25crt_170vr.pdf
Jeihani Koohbanani, M. (2004). Enhancements to transportation analysis and simulation systems (Unpublished Doctoral dissertation, Virginia Tech). https://vtechworks.lib.vt.edu/bitstream/handle/10919/30092/dissertation-final.pdf?sequence=1&isAllowed=y
Levinson, D., Liu, H., Garrison, W., Hickman, M., Danczyk, A., Corbett, M., & Dixon, K. (2014). Fundamentals of transportation . Wikimedia. https://upload.wikimedia.org/wikipedia/commons/7/79/Fundamentals_of_Transportation.pdf
Mathew, T. V., & Rao, K. K. (2006). Introduction to transportation engineering. Civil engineering–Transportation engineering. IIT Bombay, NPTEL ONLINE, Http://Www. Cdeep. Iitb. Ac. in/Nptel/Civil% 20Engineering .
Meyer, M. D. (2016). Transportation planning handbook . John Wiley & Sons.
Qasim, G. (2015). Travel demand modeling: AL-Amarah city as a case study . [Unpublished Doctoral dissertation , the Engineering College University of Baghdad]
Rojo, M. (2020). Evaluation of traffic assignment models through simulation. Sustainability , 12 (14), 5536. https://doi.org/10.3390/su12145536
Sheffi, Y. (1985). Urban transportation networks: Equilibrium analysis with mathematical programming method . Prentice-Hall. http://web.mit.edu/sheffi/www/selectedMedia/sheffi_urban_trans_networks.pdf
US Bureau of Public Roads. (1964). Traffic assignment manual for application with a large, high speed computer . U.S. Department of Commerce, Bureau of Public Roads, Office of Planning, Urban Planning Division.
https://books.google.com/books/about/Traffic_Assignment_Manual_for_Applicatio.html?id=gkNZAAAAMAAJ
Wang, X., & Hofe, R. (2008). Research methods in urban and regional planning . Springer Science & Business Media.
Polynomial is distribution that involves the non-negative integer powers of a variable.
Hyperbolic function is a function that the uses the variable values as the power to the constant of e.
A point on the curve where the derivation of the function becomes either maximum or minimum.
all-or-nothing is an assignment model where we assume that the impedance of a road or path between each origin and destination is constant and is equal to free-flow level
Incremental model is a model that the predictions or estimates or fed into the model for forecasting incrementally to account for changes that may occur during each increment.
Iterative feedback loop is a model that iterates between trip distribution and route choice step based on the rational that if a path gets too congested, the travel may alter travel destination
Wardrop equilibrium is a state in traffic assignment model where are drivers are reluctant to change their path because the average travel time is at a minimum.
second principle of the Wardrop is a principle that assumes drivers’ rationale for choosing a path is to minimize total system costs with one another in order to minimize total system travel time
Stochastic time-dependent OD is a modeling framework where generation and distribution of trips are randomly assigned to the area
feedback loop model is type of dynamic traffic assignment model where an iteration between route choice and traffic assignment step is peformed, based on the assumption that if a particular route gets heavily congested, the travel may change the destination (like another shopping center).
Transportation Land-Use Modeling & Policy Copyright © by Mavs Open Press. All Rights Reserved.
Share This Book

Travel Demand Forecasting: Parameters and Techniques (2012)
Chapter: front matter.
Below is the uncorrected machine-read text of this chapter, intended to provide our own search engines and external engines with highly rich, chapter-representative searchable text of each book. Because it is UNCORRECTED material, please consider the following text as a useful but insufficient proxy for the authoritative book pages.
N A T I O N A L C O O P E R A T I V E H I G H W A Y R E S E A R C H P R O G R A M NCHRP REPORT 716 Travel Demand Forecasting: Parameters and Techniques Cambridge Systematics, Inc. Cambridge, MA Vanasse Hangen Brustlin, Inc. Silver Spring, MD Gallop Corporation Rockville, MD Chandra R. Bhat Austin, TX Shapiro Transportation Consulting, LLC Silver Spring, MD Martin/Alexiou/Bryson, PLLC Raleigh, NC Subscriber Categories Highways ⢠Operations and Traffic Management ⢠Planning and Forecasting ⢠Safety and Human Factors TRANSPORTAT ION RESEARCH BOARD WASHINGTON, D.C. 2012 www.TRB.org Research sponsored by the American Association of State Highway and Transportation Officials in cooperation with the Federal Highway Administration
NATIONAL COOPERATIVE HIGHWAY RESEARCH PROGRAM Systematic, well-designed research provides the most effective approach to the solution of many problems facing highway administrators and engineers. Often, highway problems are of local interest and can best be studied by highway departments individually or in cooperation with their state universities and others. However, the accelerating growth of highway transportation develops increasingly complex problems of wide interest to highway authorities. These problems are best studied through a coordinated program of cooperative research. In recognition of these needs, the highway administrators of the American Association of State Highway and Transportation Officials initiated in 1962 an objective national highway research program employing modern scientific techniques. This program is supported on a continuing basis by funds from participating member states of the Association and it receives the full cooperation and support of the Federal Highway Administration, United States Department of Transportation. The Transportation Research Board of the National Academies was requested by the Association to administer the research program because of the Boardâs recognized objectivity and understanding of modern research practices. The Board is uniquely suited for this purpose as it maintains an extensive committee structure from which authorities on any highway transportation subject may be drawn; it possesses avenues of communications and cooperation with federal, state and local governmental agencies, universities, and industry; its relationship to the National Research Council is an insurance of objectivity; it maintains a full-time research correlation staff of specialists in highway transportation matters to bring the findings of research directly to those who are in a position to use them. The program is developed on the basis of research needs identified by chief administrators of the highway and transportation departments and by committees of AASHTO. Each year, specific areas of research needs to be included in the program are proposed to the National Research Council and the Board by the American Association of State Highway and Transportation Officials. Research projects to fulfill these needs are defined by the Board, and qualified research agencies are selected from those that have submitted proposals. Administration and surveillance of research contracts are the responsibilities of the National Research Council and the Transportation Research Board. The needs for highway research are many, and the National Cooperative Highway Research Program can make significant contributions to the solution of highway transportation problems of mutual concern to many responsible groups. The program, however, is intended to complement rather than to substitute for or duplicate other highway research programs. Published reports of the NATIONAL COOPERATIVE HIGHWAY RESEARCH PROGRAM are available from: Transportation Research Board Business Office 500 Fifth Street, NW Washington, DC 20001 and can be ordered through the Internet at: http://www.national-academies.org/trb/bookstore Printed in the United States of America NCHRP REPORT 716 Project 08-61 ISSN 0077-5614 ISBN 978-0-309-21400-1 Library of Congress Control Number 2012935156 © 2012 National Academy of Sciences. All rights reserved. COPYRIGHT INFORMATION Authors herein are responsible for the authenticity of their materials and for obtaining written permissions from publishers or persons who own the copyright to any previously published or copyrighted material used herein. Cooperative Research Programs (CRP) grants permission to reproduce material in this publication for classroom and not-for-profit purposes. Permission is given with the understanding that none of the material will be used to imply TRB, AASHTO, FAA, FHWA, FMCSA, FTA, or Transit Development Corporation endorsement of a particular product, method, or practice. It is expected that those reproducing the material in this document for educational and not-for-profit uses will give appropriate acknowledgment of the source of any reprinted or reproduced material. For other uses of the material, request permission from CRP. NOTICE The project that is the subject of this report was a part of the National Cooperative Highway Research Program, conducted by the Transportation Research Board with the approval of the Governing Board of the National Research Council. The members of the technical panel selected to monitor this project and to review this report were chosen for their special competencies and with regard for appropriate balance. The report was reviewed by the technical panel and accepted for publication according to procedures established and overseen by the Transportation Research Board and approved by the Governing Board of the National Research Council. The opinions and conclusions expressed or implied in this report are those of the researchers who performed the research and are not necessarily those of the Transportation Research Board, the National Research Council, or the program sponsors. The Transportation Research Board of the National Academies, the National Research Council, and the sponsors of the National Cooperative Highway Research Program do not endorse products or manufacturers. Trade or manufacturersâ names appear herein solely because they are considered essential to the object of the report.
The National Academy of Sciences is a private, nonprofit, self-perpetuating society of distinguished scholars engaged in scientific and engineering research, dedicated to the furtherance of science and technology and to their use for the general welfare. On the authority of the charter granted to it by the Congress in 1863, the Academy has a mandate that requires it to advise the federal government on scientific and technical matters. Dr. Ralph J. Cicerone is president of the National Academy of Sciences. The National Academy of Engineering was established in 1964, under the charter of the National Academy of Sciences, as a parallel organization of outstanding engineers. It is autonomous in its administration and in the selection of its members, sharing with the National Academy of Sciences the responsibility for advising the federal government. The National Academy of Engineering also sponsors engineering programs aimed at meeting national needs, encourages education and research, and recognizes the superior achievements of engineers. Dr. Charles M. Vest is president of the National Academy of Engineering. The Institute of Medicine was established in 1970 by the National Academy of Sciences to secure the services of eminent members of appropriate professions in the examination of policy matters pertaining to the health of the public. The Institute acts under the responsibility given to the National Academy of Sciences by its congressional charter to be an adviser to the federal government and, on its own initiative, to identify issues of medical care, research, and education. Dr. Harvey V. Fineberg is president of the Institute of Medicine. The National Research Council was organized by the National Academy of Sciences in 1916 to associate the broad community of science and technology with the Academyâs purposes of furthering knowledge and advising the federal government. Functioning in accordance with general policies determined by the Academy, the Council has become the principal operating agency of both the National Academy of Sciences and the National Academy of Engineering in providing services to the government, the public, and the scientific and engineering communities. The Council is administered jointly by both Academies and the Institute of Medicine. Dr. Ralph J. Cicerone and Dr. Charles M. Vest are chair and vice chair, respectively, of the National Research Council. The Transportation Research Board is one of six major divisions of the National Research Council. The mission of the Transporta- tion Research Board is to provide leadership in transportation innovation and progress through research and information exchange, conducted within a setting that is objective, interdisciplinary, and multimodal. The Boardâs varied activities annually engage about 7,000 engineers, scientists, and other transportation researchers and practitioners from the public and private sectors and academia, all of whom contribute their expertise in the public interest. The program is supported by state transportation departments, federal agencies including the component administrations of the U.S. Department of Transportation, and other organizations and individu- als interested in the development of transportation. www.TRB.org www.national-academies.org
C O O P E R A T I V E R E S E A R C H P R O G R A M S AUTHOR ACKNOWLEDGMENTS The research project reported herein was performed under NCHRP Project 8-61 by Cambridge Systematics, Inc. in association with Dr. Chandra R. Bhat, Vanasse Hangen Brustlin, Inc., Martin/ Alexiou/Bryson, PLLC, Gallop Corporation, and Shapiro Transportation Consulting, LLC. Cambridge Systematics served as prime contractor. Thomas Rossi of Cambridge Systematics served as project director and principal investigator. Major roles in this project were also performed by David Kurth, John (Jay) Evans, Daniel Beagan, Bruce Spear, Robert Schiffer, and Ramesh Thammiraju of Cambridge Systematics; Dr. Chandra Bhat; Richard Roisman, formerly of Vanasse Hangen Brustlin; Philip Shapiro of Shapiro Transportation Consulting (formerly of Vanasse Hangen Brustlin); C.Y. Jeng of Gallop Corporation; William Martin of Martin/Alexiou/Bryson; and Amlan Banerjee and Yasasvi Popuri, formerly of Cambridge Systematics. A peer review panel provided valuable comments on the draft report. The research team wishes to thank the peer review panel members: Charles Baber of the Baltimore Metropolitan Council, Bart Benthul of the Bryan-College Station Metropolitan Planning Organization (MPO), Mike Conger of the Knoxville Regional Transportation Planning Organization, Ken Kaltenbach of the Corradino Group, Phil Matson of the Indian River MPO, Phil Mescher of the Iowa Department of Transportation, Jeremy Raw of the Federal Highway Administration, and David Schmitt of AECOM. The research team wishes to thank the following organizations and individuals for their assistance: Nancy McGuckin, Ron Milone of the Metropolitan Washington Council of Governments, and Jeffrey Agee-Aguayo of the Sheboygan MPO. CRP STAFF FOR NCHRP REPORT 716 Christopher W. Jenks, Director, Cooperative Research Programs Crawford F. Jencks, Deputy Director, Cooperative Research Programs Nanda Srinivasan, Senior Program Officer Charlotte Thomas, Senior Program Assistant Eileen P. Delaney, Director of Publications Natalie Barnes, Senior Editor NCHRP PROJECT 08-61 PANEL Field of Transportation PlanningâArea of Forecasting Thomas J. Kane, Thomas J. Kane Consulting, Urbandale, IA (Chair) Michael S. Bruff, North Carolina DOT, Raleigh, NC Ed J. Christopher, Berwyn, IL Nathan S. Erlbaum, New York State DOT, Albany, NY Jerry D. Everett, University of Tennessee, Knoxville, TN Bruce Griesenbeck, Sacramento Area Council of Governments, Sacramento, CA Herbert S. Levinson, Wallingford, CT Richard H. Pratt, Richard H. Pratt, Consultant, Inc., Garrett Park, MD Bijan Sartipi, California DOT, Oakland, CA Shuming Yan, Washington State DOT, Seattle, WA Ken Cervenka, FTA Liaison Kimberly Fisher, TRB Liaison
F O R E W O R D This report is an update to NCHRP Report 365: Travel Estimation Techniques for Urban Planning and provides guidelines on travel demand forecasting procedures and their application for solving common transportation problems. The report presents a range of approaches that allow users to determine the level of detail and sophistication in select- ing modeling and analysis techniques most appropriate to their situations and addresses straight-forward techniques, optional use of default parameters, and appropriate references to other more sophisticated techniques. In 1978, TRB published NCHRP Report 187: Quick-Response Urban Travel Estimation Techniques and Transferable Parameters. This report described default parameters, factors, and manual techniques for doing simple planning analysis. The report and its default data were used widely by the transportation planning profession for almost 20 years. In 1998, drawing on several newer data sources including the 1990 Census and National Personal Household Travel Survey, an update to NCHRP Report 187 was published as NCHRP Report 365: Travel Estimation Techniques for Urban Planning. Since NCHRP Report 365 was published, significant changes have occurred affecting the complexity, scope, and context of transportation planning. Planning concerns have grown beyond âurbanâ to include rural, statewide, and special-use lands. Transportation planning tools have evolved and proliferated, enabling improved and more flexible analyses to support decisions. The demands on transportation planning have expanded into special populations (e.g., tribal, immigrant, older, and young) and broader issues (e.g., safety, congestion, pricing, air quality, environment, and freight). In addition, the default data and parameters in NCHRP Report 365 needed to be updated to reflect the planning requirements of today and the next 10 years. Thus, the objective of this research was to revise and update NCHRP Report 365 to reflect current travel characteristics and to provide guidance on travel demand forecasting procedures and their application for solving common transportation problems. The research was performed by Cambridge Systematics, Inc. in association with Vanasse Hangen Brustlin, Inc., Gallop Corporation, Dr. Chandra R. Bhat, Shapiro Transportation Consulting, LLC, and Martin/Alexiou/Bryson, PLLC. Information was gathered via liter- ature review, interviews with practitioners, and a database of parameters collected from metropolitan planning organizations as well as from the 2009 National Household Travel Survey. Planners can make use of the information presented in this report in two primary ways: (1) to develop travel model components when local data suitable for model develop- ment are insufficient or unavailable and (2) to check the reasonableness of model outputs. By Nanda Srinivasan Staff Officer Transportation Research Board
C O N T E N T S 1 Chapter 1 Introduction 1 1.1 Background 2 1.2 Travel Demand Forecasting: Trends and Issues 3 1.3 Overview of the Four-Step Travel Modeling Process 5 1.4 Summary of Techniques and Parameters 5 1.5 Model Validation and Reasonableness Checking 5 1.6 Advanced Travel Analysis Procedures 5 1.7 Case Study Applications 5 1.8 Glossary of Terms Used in This Report 7 Chapter 2 Planning Applications Context 7 2.1 Types of Planning Analyses 10 2.2 Urban Area Characteristics Affecting Planning and Modeling 14 Chapter 3 Data Needed for Modeling 14 3.1 Introduction 14 3.2 Socioeconomic Data and Transportation Analysis Zones 18 3.3 Network Data 24 3.4 Validation Data 27 Chapter 4 Model Components 27 4.1 Introduction 31 4.2 The Logit Model 33 4.3 Vehicle Availability 37 4.4 Trip Generation 43 4.5 Trip Distribution 48 4.6 External Travel 53 4.7 Mode Choice 58 4.8 Automobile Occupancy 62 4.9 Time of Day 65 4.10 Freight/Truck Modeling 72 4.11 Highway Assignment 77 4.12 Transit Assignment 80 Chapter 5 Model Validation and Reasonableness Checking 80 5.1 Introduction 80 5.2 Model Validation Overview 81 5.3 Model Validation and Reasonableness Checking Procedures for Existing Models 86 5.4 Model Validation and Reasonableness Checking Procedures for Models or Model Components Developed from Information Contained in Chapter 4 88 5.5 Cautions Regarding Use of This Report for Validation
89 Chapter 6 Emerging Modeling Practices 90 6.1 The Activity-Based Approach 92 6.2 Activity-Based Travel Model Systems in Practice 96 6.3 Integration with Other Model Systems 99 6.4 Summary 100 Chapter 7 Case Studies 100 7.1 Introduction 100 7.2 Model Reasonableness Check 108 7.3 Model Development Case Study for a Smaller Area without Data for Model Estimation 114 References A-1 Appendix A Federal Planning and Modeling Requirements B-1 Appendix B Review of Literature on Transferability Studies C-1 Appendix C Transferable Parameters Note: Many of the photographs, figures, and tables in this report have been converted from color to grayscale for printing. The electronic version of the report (posted on the Web at www.trb.org) retains the color versions.
TRB’s National Cooperative Highway Research Program (NCHRP) Report 716: Travel Demand Forecasting: Parameters and Techniques provides guidelines on travel demand forecasting procedures and their application for helping to solve common transportation problems.
The report presents a range of approaches that are designed to allow users to determine the level of detail and sophistication in selecting modeling and analysis techniques based on their situations. The report addresses techniques, optional use of default parameters, and includes references to other more sophisticated techniques.
Errata: Table C.4, Coefficients for Four U.S. Logit Vehicle Availability Models in the print and electronic versions of the publications of NCHRP Report 716 should be replaced with the revised Table C.4 .
NCHRP Report 716 is an update to NCHRP Report 365 : Travel Estimation Techniques for Urban Planning .
In January 2014 TRB released NCHRP Report 735 : Long-Distance and Rural Travel Transferable Parameters for Statewide Travel Forecasting Models , which supplements NCHRP Report 716.
READ FREE ONLINE
Welcome to OpenBook!
You're looking at OpenBook, NAP.edu's online reading room since 1999. Based on feedback from you, our users, we've made some improvements that make it easier than ever to read thousands of publications on our website.
Do you want to take a quick tour of the OpenBook's features?
Show this book's table of contents , where you can jump to any chapter by name.
...or use these buttons to go back to the previous chapter or skip to the next one.
Jump up to the previous page or down to the next one. Also, you can type in a page number and press Enter to go directly to that page in the book.
To search the entire text of this book, type in your search term here and press Enter .
Share a link to this book page on your preferred social network or via email.
View our suggested citation for this chapter.
Ready to take your reading offline? Click here to buy this book in print or download it as a free PDF, if available.
Get Email Updates
Do you enjoy reading reports from the Academies online for free ? Sign up for email notifications and we'll let you know about new publications in your areas of interest when they're released.
The Travel Cost Model
Cite this chapter.
- George R. Parsons 3
Part of the book series: The Economics of Non-Market Goods and Resources ((ENGO,volume 3))
1969 Accesses
90 Citations
The travel cost model is used to value recreational uses of the environment. For example, it may be used to value the recreation loss associated with a beach closure due to an oil spill or to value the recreation gain associated with improved water quality on a river. The model is commonly applied in benefit-cost analyses and in natural resource damage assessments where recreation values play a role. Since the model is based on observed behavior, it is used to estimate use values only.
This is a preview of subscription content, log in via an institution to check access.
Access this chapter
- Available as PDF
- Read on any device
- Instant download
- Own it forever
- Compact, lightweight edition
- Dispatched in 3 to 5 business days
- Free shipping worldwide - see info
Tax calculation will be finalised at checkout
Purchases are for personal use only
Institutional subscriptions
Unable to display preview. Download preview PDF.
Adamowicz, W. L. 1994. Habit Formation and Variety Seeking in a Discrete Choice Model of Recreation Demand. Journal of Agricultural and Resource Economics 19(1):19–31.
Google Scholar
Adamowicz, W., J. Louviere, and M. Williams. 1994. Combining Revealed and Stated Preference Methods for Valuing Environmental Amenities. Journal of Environmental Economics and Management 26:271–92.
Adamowicz, W. L., J. Swait, P. Boxall, and M. Williams. 1997. Perception Versus Objective Measures of Environmental Quality in Combined Revealed and Stated Preference Models of Environmental Evaluation. Journal of Environmental Economics and Management 32:65–84.
Andrews, T. 1996. A Discrete Choice Model of Recreational Trout Angler Benefits in Pennsylvania. Unpublished manuscript, Department of Economics, West Chester University.
Ben-Akiva, M., and S. Lerman. 1985. Discrete Choice Analysis . Cambridge, MA: MIT Press.
Bockstael, N. E. 1995. Travel Cost Models. Handbook of Environmental Economics . Cambridge, MA: Blackwell Publishers.
Bockstael, N. E., and I. E. Strand. 1987. The Effect of Common Sources of Regression Error on Benefit Estimates. Land Economics 63(1):11–20.
Bockstael, N., W. M. Hanemann, and C. L. Kling. 1987. Estimating the Value of Water Quality Improvements in a Recreational Demand Framework. Water Resources Research 23(5):951–960.
Bockstael, N. E., W. M. Hanemann, and I. E. Strand, Jr. 1984. Measuring the Benefits of Water Quality Improvements Using Recreation Demand Models. Report to the U.S. Environmental Protection Agency. College Park, MD: University of Maryland.
Bockstael, N. E., K. E. McConnell, and I. E. Strand. 1988. Benefits from Improvements in Chesapeake Bay Water Quality. Report to the U.S. Environmental Protection Agency. College Park, MD: University of Maryland.
Bockstael, N. E., K.E. McConnell, and I. E. Strand. 1989. A Random Utility Model for Sportfishing: Some Preliminary Results for Florida. Marine Resource Economics 6:245–260.
Bockstael, N. E., K. E. McConnell, and I. E. Strand, Jr. 1991. Recreation: Measuring the Demand for Environmental Quality. New York: North Holland.
Bockstael, N. E., I. E. Strand, and W. M. Hanemann. 1987. Time and the Recreational Demand Model. American Journal of Agricultural Economics :293–302.
Brown, G. M., R. Congar, and E. A. Wilman. 1983. Recreation: Tourists and Residents. In Assessing the Social Costs of Oil Spills: The Amoco Cadiz Case Study . U.S. National Ocean Service, National Oceanic and Atmospheric Administration Report (NTIS PB83-100536). Washington, DC.
Brown, G., and R. Mendelsohn. 1984. The Hedonic Travel Cost Method. Review of Economics and Statistics 66:427–433.
Burt, O., and D. Brewer. 1971. Estimation of net Social Benefits from Outdoor Recreation. Econometrica 39(5):813–828.
Chen, H. Z., and S. R. Cosslett. 1998. Environmental Quality Preference and Benefit Estimation in Multinomial Probit Models: A Simulation Approach. American Journal of Agricultural Economics 80(3):512–520.
Cicchetti, C. J., A. C. Fisher, and V. K. Smith. 1976. An Econometric Evaluation of a Generalized Consumer Surplus Measure: The Mineral King Controversy. Econometrica 44(6):1259–1276.
Creel, M. D., and J. B. Loomis. 1990. Theoretical and Empirical Advantages of Truncated Count Data Estimators for Analysis of Deer Hunting in California. American Journal of Agricultural Economics 72:434–441.
Dilman, D.A. 2000. Mail and Internet Surveys: The Tailored Design Method (2 nd edition). New York: Wiley.
Feather, P., and W. D. Shaw. 1999. Estimating the Cost of Leisure Time for Recreation Demand Models. Journal of Environmental Economics and Management 38:49–65.
Freeman, A. M. III. 1993. The Measurement of Environmental and Resource Values: Theory and Methods. Washington, DC: Resources for the Future.
Greene, W. H. 1997. Econometric Analysis (3rd Ed.). Upper Saddle River, NJ: Prentice Hall.
Haab, T. C., and K. E. McConnell. 1996. Count Data Models and the Problem of Zeros in Recreation Demand Analysis. American Journal of Agricultural Economics 78:89–102.
Haab, T. C, and K. E. McConnell. 2002. Valuing Environmental and Natural Resources . Cheltenham, UK: Edward Elgar.
Hackett, S. C. 2000. The Recreational Economic Value of the Eastern Trinity Alps Wilderness. School of Business and Economics, Humbolt State University.
Hanemann, W. M. 1999. Welfare Analysis with Discrete Choice Models. In Valuing Recreation and the Environment: Revealed Preference Methods in Theory and Practice . Edited by Herriges, J. A., and C. L. Kling. Cheltenham, UK: Edward Elgar.
Hauber, A. H. IV, and G. R. Parsons. 2000. The Effect of nesting Structure on Welfare Estimation in Random Utility Models: An Application to the Demand for Recreational Fishing. American Journal of Agricultural Economics 82:501–514.
Hausman, J. A., G K. Leonard, and D. McFadden. 1995. A Utility-Consistent, Combined Discrete Choice and Count Data Model: Assessing Recreational Use Losses Due to Natural Resource Damage. Journal of Public Economics 56:1–30.
Hellerstein, D. 1999. Can We Count on Count Models? In Valuing Recreation and the Environment: Revealed Preference Methods in Theory and Practice . Edited by Herriges, J. A., and C. L. Kling. Cheltenham, UK: Edward Elgar.
Hellerstein, D., and R. Mendelsohn. 1993. A Theoretical Foundation for Count Data Models. American Journal of Agricultural Economics 75:604–11.
Herriges, J. A., and C. L. Kling, eds. 1999. Valuing Recreation and the Environment: Revealed Preference Methods in Theory and Practice . Aldershot, UK: Edward Elgar.
Herriges, J. A., C. L. Kling, and D. J. Phaneuf. 1999. Corner Solution Models of Recreation Demand: A Comparison of Competing Frameworks. In Valuing Recreation and the Environment: Revealed Preference Methods in Theory and Practice . Edited by Herriges, J. A., and C. L. Kling. Cheltenham, UK: Edward Elgar.
Hicks, R. L. and I. Strand. 2000. The Extent of Information: Its Relevance for Random Utility Models. Land Economics 76(3):374–385.
Hoehn, J. P., T. Tomasi, F. Lupi, and H. Z. Chen. 1996. An Economic Model for Valuing Recreational Angling Resources in Michigan. Michigan State University, Report to the Michigan Department of Environmental Quality.
Karou, Y. S1995. Measuring Marine Recreation Benefits of Water Quality Improvements by the Nested Random Utility Model. Resource and Energy Economics 17:119–136.
Kolstad, C. D. 2000. Environmental Economics . New York: Oxford University Press.
Laitila, T. 1999. Estimation of Combined Site-Choice and Trip-Frequency Models of Recreational Demand Using Choice-Based and On site Samples. Economic Letters 64:17–23.
Layman, R. C, J. R. Boyce, and K. R. Criddle. 1996. Economic Valuation of the Chinook Salmon Sport Fishery of the Gulkana River, Alaska, under Current and Alternate Management Plans. Land Economics 72(1):113–128.
Loomis, J. B., and R. G. Walsh. 1997. Recreation Economic Decisions: Comparing Benefits and Costs . State College, PA: Venture.
Lupi, F., and P. M. Feather. 1998. Using Partial Aggregation to Reduce Bias in Random Utility Travel Cost Models. Water Resources Research 34(12):3595–3603.
McConnell, K. E. 1986. The Damages to Recreational Activities from PCB’s in New Bedford Harbor. University of Maryland, Department of Agricultural and Resource Economics. Report prepared for the Ocean Assessment Division, National Oceanic and Atmospheric Administration.
McConnell, K. E. 1992. On site Time in the Demand for Recreation. American Journal of Agricultural Economics 74:918–925.
McConnell, K E., I. Strand, and L. Blake-Hedges. 1995. Random Utility Models of Recreational Fishing: Catching Fish Using a Poisson Process. Marine Resource Economics 10:247–261.
McConnell, K. E., and I. E. Strand. 1981. Measuring the Cost of Time in Recreation Demand Analysis. American Journal of Agricultural Economics 63:153–156.
McConnell, K. E. and I. E. Strand. 1994. The Economic Value of Mid and South Atlantic Sportfishing. University of Maryland, Report to the U.S. EPA and NOAA. Department of Agricultural and Resource Economics, University of Maryland.
McFadden, D. 2001. Economic Choices. American Economic Review 91(3):351–378.
Mendelsohn, R., J. Hof, G. Peterson, and R. Johnson. 1992. Measuring Recreation Values with Multiple Destination Trips. American Journal of Agricultural Economics 74:926–933.
Montgomery, M. and M. Needelman. 1997. The Welfare Effects of Toxic Contamination in Freshwater Fish. Land Economics 73:211–223.
Morey, E. 1981. The Demand for Site-Specific Recreational Activities: A Characteristics Approach. Journal of Environmental Economics and Management 8:345–371.
Morey, E. 1999. Two Rums Uncloaked: Nested-Logit Models of Site Choice and Nested-Logit Models of Participation and Site Choice. Chapter 3 in Valuing Recreation and the Environment: Revealed Preference Methods in Theory and Practice . Edited by Herriges, J. A., and C. L. Kling. Cheltenham, UK: Edward Elgar.
Morey, E., R. D. Rowe, and M. Watson. 1993. A Repeated Nested-Logit Model of Atlantic Salmon Fishing. American Journal of Agricultural Economics 75:578–592.
Murray, C, B. Sohngen, and L. Pendelton. 2001. Valuing Water Quality Advisories and Beach Amenities in the Great Lakes. Water Resources Research 37(10):2583–2590.
Ozuna, T., and I. A. Gomez 1994. Estimating a System of Recreation Demand Function Using a Seemingly Unrelated Poisson Regression Approach. Review of Economics and Statistics 76:356–360.
Parsons, G. R. 1991. A Note on Choice of Residential Location in Travel Cost Demand Models. Land Economics 67(3):360–364.
Parsons, G. R., and M J. Kealy. 1992. Randomly Drawn Opportunity Sets in a Random Utility Model of Lake Recreation. Land Economics 68(1):93–106.
Parsons, G. R., and M. Needelman. 1992. Site Aggregation in a Random Utility Model of Recreation. Land Economics 68(4):418–433.
Parsons, G. R., and A. J. Wilson. 1997. Incidental and Joint Consumption in Recreation Demand. Agricultural and Resource Economics Review 26:1–6.
Parsons, G. R., and A. B. Hauber. 1998. Spatial Boundaries and Choice Set Definition in a Random Utility Model of Recreation Demand. Land Economics 74(1):32–48.
Parsons, G R., P. M. Jakus, and T. Tomasi. 1999. A Comparison of Welfare Estimates from Four Models for Linking Seasonal Recreational Trips to Multinomial Logit Models of Site Choice. Journal of Environmental Economics and Management 38:143–157.
Parsons, G. R., D. M. Massey, and T. Tomasi. 1999. Familiar and Favorite Sites in a Random Utility Model of Beach Recreation. Marine Resource Economics 14:299–315.
Parsons, G. R., and D. M. Massey. 2003. A RUM Model of Beach Recreation. In The New Economics of Outdoor Recreation . Edited by Hanely, N., W. D. Shaw, R. E. Wright, and J. C. Coleman. Cheltenham, UK: Edward Elgar.
Parsons, G. R., A. J. Plantinga, and K. J. Boyle. 2000. Narrow Choice Sets in a Random Utility Model of Recreation Demand. Land Economics 76(1):86–99.
Peters, T., W. L. Adamowicz, and P. C. Boxall. 1995. Influence of Choice Set Considerations in Modeling the Benefits from Improved Water Quality. Water Resources Research 31(7):T781–1787.
Phaneuf, D. J., C. L. Kling, and J. Herriges. 2000. Estimation and Welfare Calculation in a Generalized Corner Solution Model With an Application to Recreation Demand. Review of Economics and Statistics 82:83–92.
Phaneuf, D. J. and V. K. Smith. 2002. Recreation Demand Models. Unpublished manuscript, North Carolina State University.
Randall, A. 1994. A Difficulty with the Travel Cost Method. Land Economics 70(1):88–96.
Shaw, D. 1988. ‘On site Samples’ Regression Problems of Nonnegative Integers, Truncation, and Enodgenous Stratification. Journal of Econometrics 37:211–223.
Shaw, W. D., and P. Jakus. 1996. Travel Cost Models of the Demand for Rock Climbing. Agricultural and Resource Economics Review 25(2):133–142.
Shaw, W. D., and M. Ozog. 1999. Modeling Overnight Recreation Trip Choice: Application of a Repeated Multinomial Logit Model. Environmental and Resource Economics 13(4):397–414.
Shonkwiler, J. S. 1999. Recreation Demand Systems for Multiple Site Count Data Travel Cost Models. Chapter 9 in Valuing Recreation and the Environment: Revealed Preference Methods in Theory and Practice . Edited by Herriges, J. A., and C. L. Kling. Cheltenham, UK: Edward Elgar.
Shonkwiler, J. S. and W. D. Shaw. 1996. Hurdle Count-Data Models in recreation Demand Analysis. Journal of Agricultural and Resource Economics 21(2):210–219.
Siderelis, C, G. Brothers, and P. Rea. 1995. A Boating Choice Model for the Valuation of Lake Access. Journal of Leisure Research 27(3):264–282.
Siderelis, C, and R. Moore. 1995. Outdoor Recreation Net Benefits of Rail-Trails. Journal of Leisure Research 27(4):344–359.
Smith, V. K., W. H. Desvousges, and M. P. McGivney. 1983. The Opportunity Cost of Travel Time in Recreation Demand Models. Land Economics 59(3):259–277.
Smith, V. K, and W. H. Desvousges. 1985. The Generalized Travel Cost Model and Water Quality Benefits: A Reconsideration. Southern Economics Journal 52(2):371–381.
Smith, V. K., W. H. Desvousges, and M. P. McGivney. 1983. Estimating Water Quality Benefits: An Econometric Analysis. Southern Economic Journal 50(2):422–437.
Sohngen, B. 2000. The Value of Day Trips to Lake Erie Beaches. Unpublished report, Dept. of Agricultural, Environmental, and Development Economics, Ohio State University.
Train, K. E. 1999. Mixed Logit Models for Recreation Demand. Chapter 4 in Valuing Recreation and the Environment: Revealed Preference Methods in Theory and Practice . Edited by Herriges, J. A., and C. L. Kling. Cheltenham, UK: Edward Elgar.
Ward, F. A., and D. Beal. 2000. Valuing Nature with Travel Cost Models: A Manuel. Cheltenham, UK: Edward Elgar.
Download references
Author information
Authors and affiliations.
University of Delaware, USA
George R. Parsons
You can also search for this author in PubMed Google Scholar
Editor information
Editors and affiliations.
Rocky Mountain Research Station, U.S. Forest Service, Fort Collins, CO, USA
Patricia A. Champ & Thomas C. Brown &
Department of Resource Economics & Policy, University of Maine, USA
Kevin J. Boyle ( Libra Professor of Environmental Economics ) ( Libra Professor of Environmental Economics )
Rights and permissions
Reprints and permissions
Copyright information
© 2003 Springer Science+Business Media New York
About this chapter
Parsons, G.R. (2003). The Travel Cost Model. In: Champ, P.A., Boyle, K.J., Brown, T.C. (eds) A Primer on Nonmarket Valuation. The Economics of Non-Market Goods and Resources, vol 3. Springer, Dordrecht. https://doi.org/10.1007/978-94-007-0826-6_9
Download citation
DOI : https://doi.org/10.1007/978-94-007-0826-6_9
Publisher Name : Springer, Dordrecht
Print ISBN : 978-1-4020-1445-1
Online ISBN : 978-94-007-0826-6
eBook Packages : Springer Book Archive
Share this chapter
Anyone you share the following link with will be able to read this content:
Sorry, a shareable link is not currently available for this article.
Provided by the Springer Nature SharedIt content-sharing initiative
- Publish with us
Policies and ethics
- Find a journal
- Track your research

Activity Based Models
Uses for Activity-Based Models
Current Practice
Modeling Approaches
Constraint-based models
Utility-maximizing models
Computational process models
Early Applications in the US
Latest Developments
Future Prospects
Page categories
Topic Circles
More pages in this category:
(opens new window) is derived from people's daily activity patterns. Activity-based models predict which activities are conducted when, where, for how long, for and with whom, and the travel choices they will make to complete them. Having this type of detailed model at their disposal allows researchers, practitioners, and policy makers to evaluate the effect of alternative policies on individuals travel behavior at a high level of temporal and spatial resolution and select the best policy alternative considering a potential wide range of performance indicators. For a comprehensive introductory overview of this paradigm, consider reading the Activity Based Modeling Primer published under SHRP2 in 2014.
# Background
Activity based models represent an emerging practice in regional transportation planning. Explaining activity-based models to a general audience remains an ongoing challenge. The genesis and continued support for their development is derived from the challenges of modern transportation planning practice itself, especially with regard to distinguishing between the social outcomes of transportation policy and the underlying choices made by travelers.
While academic research and development has been underway since the 1970s, practical implementation in a handful of, mainly large, urban regions in the US began in the early 2000s. As of 2015, state of practice differs largely between countries. In Europe, a limited number of countries such as The Netherlands and Belgium and Switzerland apply activity-based models in practice.
# Uses for Activity-Based Models
Activity-based models are uniquely suited to:
- Permitting a continuous distribution of value-of- travel-time-savings across the population; essential for highway pricing evaluations
- A clearer accounting of the full range of costs and utilities associated with both travel and activity by individual persons; improves transit mode choice estimation
These features also improve the analyst's ability to specify the parameters associated with local travel (e.g. walking and biking) as well as person-specific attributes that can effect travel choices (e.g. age, gender, willingness-to-pay). Because each person is uniquely specified and their personal itinerary is preserved, it is easier to make intuitive connections to spatial (e.g. land use, aerial image) databases that can better explain the continuity of activity patterns throughout the day.
# Current Practice
(opens new window) and tracking of individual travel choices. These features permit the model results to retain the disaggregate character of the original [Travel survey data|travel survey] data; making them appealing to a wide variety of policy analyses.
# Modeling Approaches
Three theoretical approaches are distinguished in the construction of activity based models.
# Constraint-based models
(opens new window) ), CARLA (Jones et al. 1983), BSP (Huigen, 1986), MAGIC (Dijst, 1995; Dijst and Vidakovic, 1997), and GISICAS (Kwan, 1997). There are some limitations in classic constraint based approaches .
# Utility-maximizing models
This stream of models are based on the premise that individuals maximize their utility when organizing their daily schedule. Some representative examples are are the daily activity schedule model (Ben-Akiva, et al., 1996), and PCATS (Kitamura and Fujii, 1998)
# Computational process models
Some scholars advocate the idea of mimicking decision heuristics to avoid the unrealistic assumption uncertainty analysis]] that individuals are maximizing the utility of their daily schedule. ALBATROSS (Arentze and Timmermans, 2000) and TASHA (Roorda and Miller, 2006) are examples of computational process models.
# Early Applications in the US
(opens new window) was a study commissioned by the Ohio Department of Transportation to compare the earliest of tour-based models to a similar trip-based model for Columbus, Ohio.
# Latest Developments
(opens new window) environment. As acceptance of the fundamental features of activity-based model converges some efforts have been made to standardize data definitions and execution code with the goal of offering robust proprietary applications for widespread use.
(opens new window) tools (e.g. dynamic traffic assignment) and the level-of-service information required by the activity based demand model.
# Future Prospects
There are still several issues remaining in the current designs and implementations of activity based models that need further attention and discussion. Future prospect for activity-based models on one hand increase the complexity of these models and increase the necessity to do formal uncertainty analysis. On the other hand, these future prospects for these activity based models may help promote and establish the activity based model as a more integrated, interdependent framework.
← Trip-based models Trip Generation →
This site uses cookies to learn which topics interest our readers.
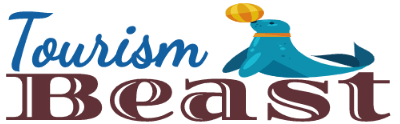
Travel Motivation
There is always a motive behind everything happens in this world. Always there is a motivation in doing any work or anything. There are mainly two factors in behind happening anything it may be pull factors or push factors. In tourism, behind the movement of tourist there are various motives, it may may be leisure, business, pilgrimage or any other.
As we know tourism is a people centric and one of the fastest growing industry. Assessing behavior and motivations of tourist is a critical task as travel decisions of tourist depends on it. Travel has been a nomadic urge in human earlier in quest of food. As humanity grows desire for shelter came into being and with the rises of civilization search of trade bundles with safety and security. The game changing moment for mass tourism comes with the rise of leisure time. Assessing motivations of tourist is a critical task as travel decisions of tourist depends on it.
Motivation is an intrinsic property of a human-being that arises from the inside or of psychological origin. A combination of internal and external forces that motivates to drive the tourism industry. To satisfy all the levels of needs such psychological, physiological, etc. humans travels. It tells of tourist attempt to satisfy their recognized need and strongly influence decisions made by tourist in every steps of decision. Various theories have been developed to reveal the most important question why people travel?
The answer to this question is influenced by the nature of the travel motives.
4. Types of Motivation
Motivation can be classified into categories:
1. Intrinsic Motivation:
this type of motivation comes from the inside through the core of the heart and mind of the tourist to do any specific work. t is defined as the performing of an activity for its intrinsic motivation that emerges from an individual’s inherent satisfactions, enjoyment, challenge entailed, interest or the feeling of inner pleasure that drives from the task rather than for some external pressures, or rewards or any separable consequence.
2. Extrinsic Motivation:
All the external factors which tends and individual to do any specific work. Individuals performs certain activities in order to attain or receive something from the outcomes. It involves executing an action influenced by the outer stimuli, to attain an external reinforcements or rewards from others such as money, praise, status etc.
Travel Motivation Theories
The tourist selecting to travel for business, leisure, pilgrimage, adventure or for other reasons, depends on motivation to visit destination. There are some common factors influencing tourist travel decisions such as
• Availability free time
• Disposable income
• Age and status
• Attractiveness of destinations
A. Gray’s Travel-Motivation Theory .
Gray explains the motivation of individual and given two motives for travel:
Wanderlust:
It describes the motive or the desire to go from a known to an unknown place. It is travelling from or leaving a familiar places to go and see different or unfamiliar places. It is about going to different destinations to experience monumental and socio-cultural heritage.
It is a type of travel to a destination that can provide the tourist with specific facilities or better amenities. It is travelling for particular purpose or facilities that are not available in place of residence of the tourist.
B. McIntosh and Goeldner Categorization of Travel Motivation
McIntosh has stated that basic travel motivators may be grouped into four broad categories:
1. Physical Motivators
These types of motivation are concerned with the individual physical health and well-being involves physical relaxation and rest, sporting activities, medical care or treatment and specific remedial health management. It include physical motivators that are concern with health from recreation to attending yoga camp to medical treatment for upkeep of health.
2.Interpersonal Motivators
Human are social animal and always keen to make new friends, have a desire to visit and meet relatives and friends, or simply want to escape from the daily hassles of everyday life. These type of motivators are termed as interpersonal motivators.
3.Cultural Motivators
Cultural motivators are describe as curiosity that tourists have and want to experience different people’s cultures and lifestyle. These are related with tourists desire to travel to different destination, in order to know about other countries, people, their culture, tradition, life style, art, music etc.
4.Status and Prestige Motivators
Tourists travel to secure respect among their friends or in family and recognition of education and knowledge or for pursuit of hobbies. These types of motivators are identified with the need for fame and status or of personal esteem and personal development. It also include travel for business or professional interests.
C. Anomie and Ego Enhancement Motivation Theory
Dann investigated two push factors and proposes that motivational factors can be grouped into anomie and ego enhancement.
Anomie refers to the need and desire to rise above the feeling of loneliness and quarantine inherent in everyday life and to get away from daily hassles. It is associated with search for life’s meaning and interaction with family and friends and social communication. The anomic tourists are mainly young, married, male mostly repeat visitors. They are from urban and rural areas and are above-average socio-economic status.
2.Ego enhancement
Ego-enhancement tourists are the opposite end of spectrum. It derived from desire and need for recognition. The individual’s desire and need for social recognition is mainly associated with it and is achieved through the status or ego enhance conferred by travel. Ego-enhancement tourists are likely to be female, married or single mostly first-time visitors. They are older than anomic tourists and typically from lower socio-economic group.
Dann argues that push factors are logically, and often an antecedent to pull factors. The question that ‘what makes tourists travel’ can only be recognized through the push factors. As the tourist to take two different difficult decisions at two diverse times i.e. “whether to go” and “where to go”.
D. Push and Pull Theory
Crompton identified two different levels of socio-psychological motivation. The first level of motivation is the desire to travel, that drives the initial decision for a trip or a vacation. It subsequently facilitates individual’s decision for destination. The second level of motivation drives the decision of selection of destinations. After the initial decision for making a trip, various motivation factors comes into being that impact the selection of destination. Crompton proposes two groups of motives among pleasure vacationers, one that push for a trip or tour are socio-psychological motives, while another factors that pull are cultural motives.
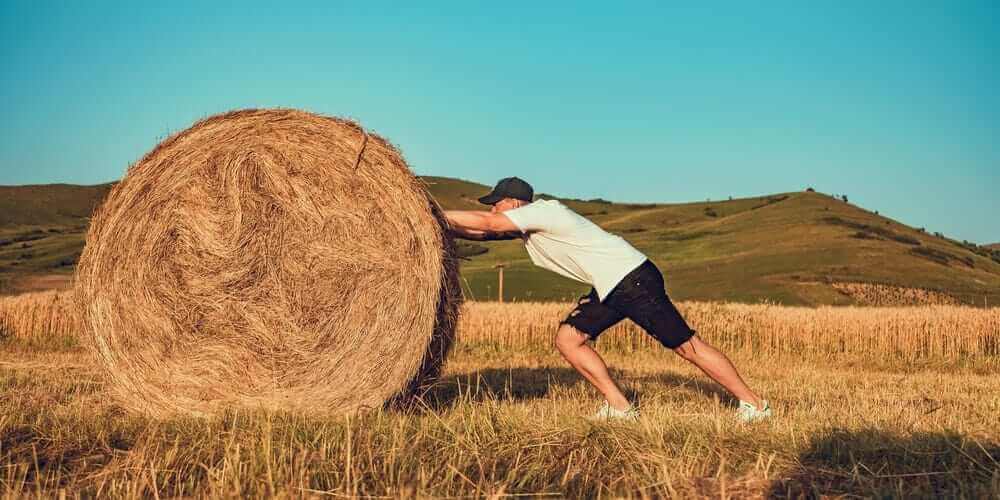
1.Push Factors.
Push factors are those factors which motivate people to travel. Push factors are initiating travel desire of an individual to engage in recreational activities or tourism. .Push factors are the inner motives which tends tourists to seek activities for needs fulfillment. Some push factors of travelling are:
• Exploration and evaluation of self.
• Escape from a perceived mundane environment.
• Relaxation.
• Health and fitness.
• Re-experiencing family.
• Facilitation of social interaction.
• Enhancement of kinship relationships.
• Novelty.
• Cultural experiences.
• Education.
• Prestige.
2.Pull Factors
All the external factors that pulls or attracts and individual towards some specific things or any destination. Pull factors appear due to the attractiveness and the attributes associated with the destination. It is related to the external condition, cognitive aspects or choices available on a destination such as attractions, climate, culture etc. Everyone has unique taste and choices which attract or pull individuals experience the destination. Identifying a set of pull factors that can be applicable to all sites is possible as different destinations have different or unique set of pull factors.
Pull factors were characterized by facilities , core attractions and landscape features .
• The facilities factor encompasses all tourism facilities of a destination including fooding, lodging, roads, hospitality and security.
• The core attractions factor includes all those activities and services available for tourism. It includes sports activities, night life, entertainment and amusement and shopping facilities.
• The landscape features pull factor strongly relates to the geographical and sociocultural features of destination i.e. natural and cultural environment of destination.
E. Escaping and Seeking Motivation of Mannell and Iso-Ahola
Mannell and Iso-Ahola defined the motivation in socio psychological perspective. A two-dimensional model of tourist motivation where both the forces simultaneously influence tourists’ behaviour. The theory consists of both personal and interpersonal escape & seeking motives together. An individual perceive that satisfaction can be feel from leisure related activities, for two major reasons
Escaping – the desire to get out from the monotonous environment of work.
Seeking – the aspiration to get intrinsic psychological rewards from other or self through traveling
Tourist are motivated for leisure or tourism related activities in order to get away from the personal and/or interpersonal problems of day to day hustle and bustle of life and get personal and/or interpersonal rewards from passive and active tourism activities.
Personal rewards are more about individual satisfaction includes exploration and relaxation; learning, challenge, a sense of competence etc.
Interpersonal rewards are arising from social interaction with family and friends or with other people in destination.
Intrinsic rewards arise from the activity that provide certain feelings, such as a feeling of mastery and escape from the monotonous environment.
F. Travel Career Ladder (TCL)
The travel career ladder approach was proposed by Pearce. The approach is based on Maslow’s hierarchy of needs theory of motivation.
Following Maslow theory, the proposed model sees the needs of travelers is organized into a hierarchy or ladder model. Biological needs including relaxation at the foundation level, followed by safety needs and relationships needs, and in line selfesteem and development needs, and fulfillment needs at the highest level.
The model identified five steps of hierarchy affecting tourist behaviour. It suggests that a group of needs in the ladder steps or level can be dominant at a particular time but travel motivation of tourist may derive from the different level of ladder. It emphasizes all the motives for traveling of a tourist, rather than a single motive. TCL proposes that every individual or tourist headway upward through hierarchy levels with accumulated travel experience and psychologically maturity.
This shows that travel motivation changes as tourist acquire experiences in tourism developmental and dynamic motivations process. An individual can descend or ascend on the ladder as the direction of the change in level may vary with the experience. Some individuals may undergo every steps or level on either side of the model or may ascend the ladder on one side of the schema. Each individual every time not seek the similar type of experience or fulfillment from travel. For example: First time visitor may be more cautious of safety and security then repeat visitor due more knowledge and experience about the destination or of trip.
G. Travel Career Pattern (TCP)
The TCP model is modification of the earlier Travel Career Ladder (TCL) approach. The TCP emphasis on motivations patterns of tourist over their life cycle because of different motivation and its impact on their travelling experience. Travel experience plays a driver role for changing travel motivations which has crucial part in
TCP model. Travel experience is the collective changes in an individual’s perspective about self and environment due to tourism activities and events that individual passes through in course of travelling.
Pearce suggested three layers of travel motivation:
Layer 1- It is the core of the TCP and includes common motives such as escape, relaxation, health and fitness, social interaction etc.
Layer 2- It comprises series of moderately important motivators that surround core layer of motivations. It related to self-actualization and interaction of guest with the host society and environment.
Layer 3- The outer layer of model embraces motives with lesser importance such as social status and nostalgia.
You Might Also Like
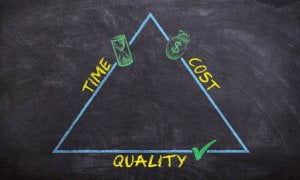
Tourism and Governance
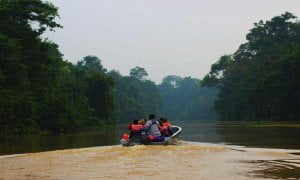
Tour Guiding
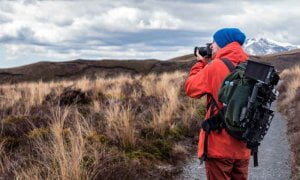
Flash Packing

Numbers, Facts and Trends Shaping Your World
Read our research on:
Full Topic List
Regions & Countries
- Publications
- Our Methods
- Short Reads
- Tools & Resources
Read Our Research On:
Discrimination Experiences Shape Most Asian Americans’ Lives
3. asian americans and the ‘model minority’ stereotype, table of contents.
- Key findings from the survey
- Most Asian Americans have been treated as foreigners in some way, no matter where they were born
- Most Asian Americans have been subjected to ‘model minority’ stereotypes, but many haven’t heard of the term
- Experiences with other daily and race-based discrimination incidents
- In their own words: Key findings from qualitative research on Asian Americans and discrimination experiences
- Discrimination in interpersonal encounters with strangers
- Racial discrimination at security checkpoints
- Encounters with police because of race or ethnicity
- Racial discrimination in the workplace
- Quality of service in restaurants and stores
- Discrimination in neighborhoods
- Experiences with name mispronunciation
- Discrimination experiences of being treated as foreigners
- In their own words: How Asian Americans would react if their friend was told to ‘go back to their home country’
- Awareness of the term ‘model minority’
- Views of the term ‘model minority’
- How knowledge of Asian American history impacts awareness and views of the ‘model minority’ label
- Most Asian Americans have experienced ‘model minority’ stereotypes
- In their own words: Asian Americans’ experiences with the ‘model minority’ stereotype
- Asian adults who personally know an Asian person who has been threatened or attacked since COVID-19
- In their own words: Asian Americans’ experiences with discrimination during the COVID-19 pandemic
- Experiences with talking about racial discrimination while growing up
- Is enough attention being paid to anti-Asian racism in the U.S.?
- Acknowledgments
- Sample design
- Data collection
- Weighting and variance estimation
- Methodology: 2021 focus groups of Asian Americans
- Appendix: Supplemental tables
In the survey, we asked Asian Americans about their views and experiences with another stereotype: Asians in the U.S. being a “model minority.” Asian adults were asked about their awareness of the label “model minority,” their views on whether the term is a good or bad thing, and their experiences with being treated in ways that reflect the stereotype.
What is the ‘model minority’ stereotype?
Amid the Civil Rights Movement in the 1960s, another narrative about Asian Americans became widespread: being characterized as a “model” minority. In 1966, two articles were published in The New York Times Magazine and U.S. News and World Report that portrayed Japanese and Chinese Americans as examples of successful minorities. Additionally, in 1987 Time magazine published a cover story on “those Asian American whiz kids.” The model minority stereotype has characterized the nation’s Asian population as high-achieving economically and educationally, which has been attributed to Asians being hardworking and deferential to parental and authority figures, among other factors. The stereotype generalizes Asians in the U.S. as intelligent, well-off, and able to excel in fields such as math and science. Additionally, the model minority myth positions Asian Americans in comparison with other non-White groups such as Black and Hispanic Americans.
For many Asians living in the United States, these characterizations do not align with their lived experiences or reflect their diverse socioeconomic backgrounds . Among Asian origin groups in the U.S., there are wide differences in economic and social experiences. Additionally, academic research has investigated how the pressures of the model minority stereotype can impact Asian Americans’ mental health and academic performance . Critics of the myth have also pointed to its impact on other racial and ethnic groups, especially Black Americans. Some argue that the myth has been used to minimize racial discrimination and justify policies that overlook the historical circumstances and impacts of colonialism, slavery and segregation on other non-White racial and ethnic groups.
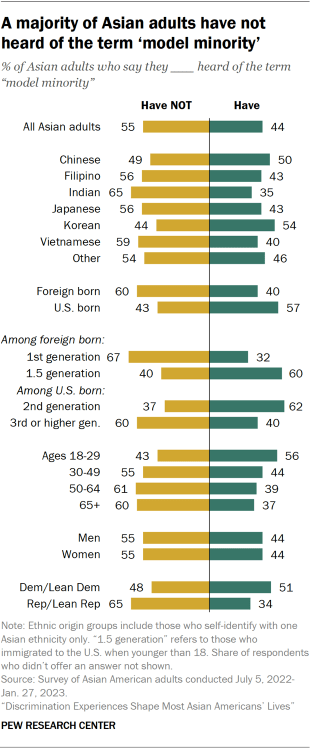
More than half of Asian adults (55%) say they have not heard of the term “model minority.” Just under half (44%) say they have heard of the term.
There are some differences in awareness of the term across demographic groups:
- Ethnic origin: About half of Korean and Chinese adults say they have heard of the term, while only about one-third of Indian adults say the same.
- Nativity: 57% of U.S.-born Asian adults have heard the term “model minority,” compared with 40% of immigrants.
- Immigrant generation: Among immigrants, 60% of those who came to the U.S. as children (“1.5 generation” in this report) say they have heard of the term “model minority,” compared with 32% of those who came to the U.S. as adults (first generation). And among U.S.-born Asian Americans, those who are second generation are more likely than those who are third or higher generation to say the same (62% vs. 40%).
- Age: 56% of Asian adults under 30 say they have heard of the term, compared with fewer than half among older Asian adults.
- Party: 51% of Asian adults who identify with or lean to the Democratic Party say they’ve heard the term, compared with 34% of those who identify with or lean to the Republican Party.
Awareness of the term ‘model minority’ varies across education and income
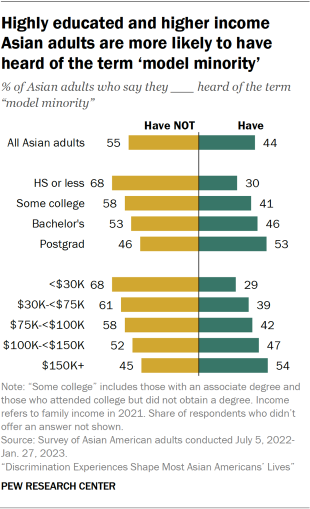
Asian adults with higher levels of formal education and higher family income are more likely to say they have heard of the term “model minority”:
- 53% of Asian adults with a postgraduate degree say they have heard the term, compared with smaller shares of those with a bachelor’s degree or less.
- 54% of Asian adults who make $150,000 or more say they have heard the term, higher than the shares among those with lower incomes. Among Asian Americans who make less than $30,000, only 29% say they have heard of the term “model minority.”
Notably, awareness of the term is higher among those born in the U.S. than immigrants across all levels of education and income.
Among Asian adults who have heard of the term “model minority,” about four-in-ten say using it to describe Asians in the U.S. is a bad thing. Another 28% say using it is neither good nor bad, 17% say using it is a good thing, and 12% say they are not sure.
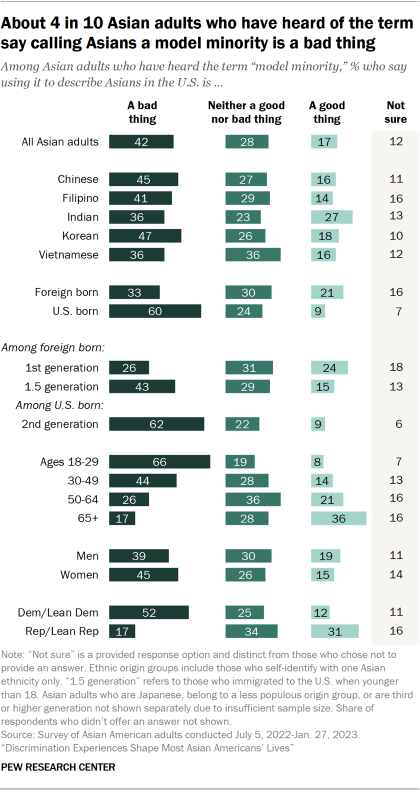
These views vary by ethnic origin, nativity, age and party. Among those who have heard of the term:
- Ethnic origin: Among Indian adults, the gap between those who say the term “model minority” is a bad thing and those who say it is a good thing (36% vs. 27%) is smaller than among other ethnic origin groups.
- Nativity: 60% of U.S.-born Asian adults say describing Asians as a model minority is a bad thing, while 9% say it is a good thing. Meanwhile, immigrants’ views of the model minority stereotype are more split (33% vs. 21%, respectively).
- Immigrant generation: Among immigrants, 43% of 1.5-generation Asian adults say using the term “model minority” is a bad thing, compared with 26% of first-generation Asian adults.
- Age: Asian adults under 30 are far more likely to say the model minority label is a bad thing than a good thing (66% vs. 8%). Meanwhile, Asian adults 65 and older are more likely to say describing Asian Americans as a model minority is a good thing (36%) than a bad thing (17%).
- Party: 52% of Asian Democrats say describing Asians as a model minority is a bad thing, about three times the share of Asian Republicans who say the same (17%).
Among those who know the term “model minority,” views of whether using it to describe Asians in the U.S. is a good or bad thing does not vary significantly across education levels. By income, Asian adults who make less than $30,000 are somewhat less likely to say it is a bad thing than those with higher incomes. 18
Views of the ‘model minority’ label are linked to perceptions of the American dream
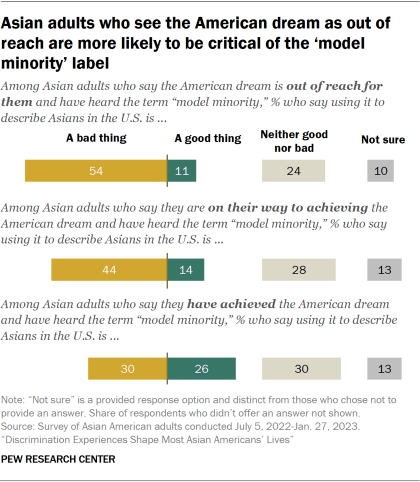
In the survey, we asked Asian Americans if they believe they have achieved the American dream, are on their way to achieving it, or if they believe the American dream is out of their reach. Among those who have heard of the term “model minority”:
- 54% of Asian adults who believe the American dream is out of their reach say describing Asian Americans as a model minority is a bad thing. This is higher than the shares among those who believe they are on their way to achieving (44%) or believe they have already achieved the American dream (30%).
- Meanwhile, 26% of Asian adults who believe they have achieved the American dream say the model minority label is a good thing. In comparison, 14% of those who believe they are on their way to achieving the American dream and 11% of those who believe that the American dream is out of their reach say the same.
In this survey, we asked Asian Americans how informed they are about the history of Asians in the U.S.
Whether Asian adults have heard of the model minority label is linked to their knowledge of Asian American history:
- 62% of Asian adults who are extremely or very informed of U.S. Asian history have heard of the term “model minority.”
- Smaller shares of those who are somewhat informed (44%) or a little or not at all informed (29%) about U.S. Asian history say they are aware of the term.
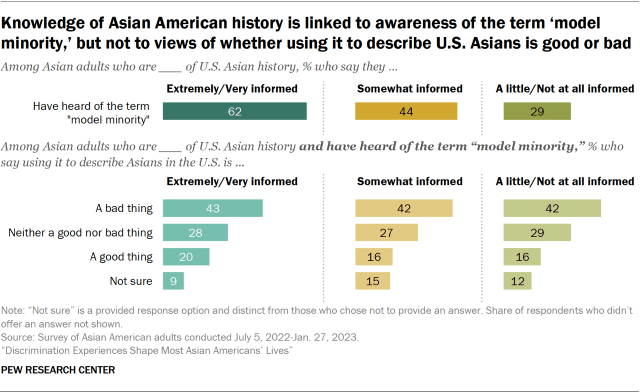
However, among those who have heard of the “model minority” label, views on whether using it to describe Asian Americans is good or bad are similar regardless of how informed they are on Asian American history. About four-in-ten across knowledge levels say describing Asian Americans as a model minority is a bad thing.
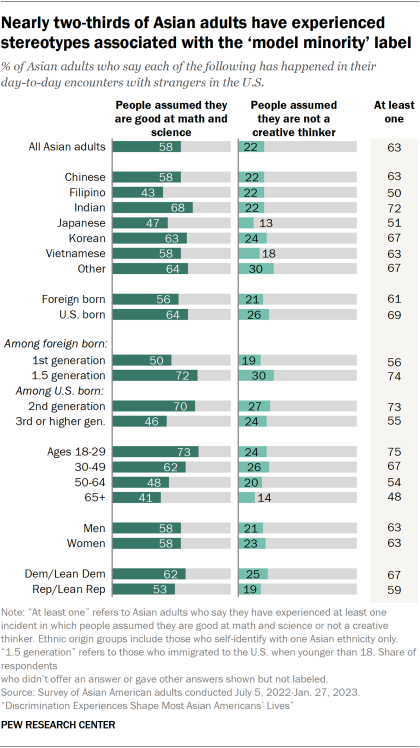
The model minority stereotype often paints Asian Americans as intellectually and financially successful, deferential to authority, and competent but robotic or unemotional , especially in comparison with other racial and ethnic groups. Additionally, some stereotypes associated with the model minority characterize Asian Americans as successful in fields such as math and science, as well as lacking in creativity.
Nearly two-thirds of Asian adults (63%) say that in their day-to-day encounters with strangers, they have at least one experience in which someone assumed they are good at math and science or not a creative thinker.
Broadly, Asian adults are far more likely to say someone has assumed they are good at math and science (58%) than not a creative thinker (22%).
Across these experiences, there are some differences by demographic groups:
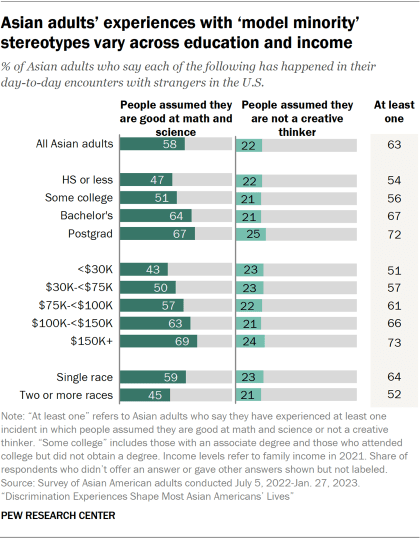
- Ethnic origin: 68% of Indian adults say strangers have assumed they are good at math and science, a higher share than among most other origin groups. Meanwhile, about half or fewer of Japanese (47%) and Filipino (43%) adults say people have made this assumption about them.
- Immigrant generation: About seven-in-ten Asian adults who are 1.5 generation and second generation each say people have assumed they are good at math and science, compared with 50% among the first generation and 46% among third or higher generations.
- Education: About two-thirds of Asian adults with a postgraduate degree or a bachelor’s degree say strangers have assumed they are good at math and science, compared with roughly half of those with some college experience or less. Similar shares regardless of education say people have assumed they are not a creative thinker.
- Income: 69% of those who make $150,000 or more say strangers have assumed they are good at math and science, compared with 43% of those who make less than $30,000.
- Race: 59% of single-race Asian adults (those who identify as Asian and no other race) say someone assumed they are good at math and science, compared with 45% of Asian adults who identify with two or more races (those who identify as Asian and at least one other race).
In our 2021 focus groups of Asian Americans, participants talked about their views of and experiences with the “model minority” stereotype.
Many U.S.-born Asian participants shared how it has been harmful , with some discussing the social pressures associated with it. Others spoke about how the stereotype portrays Asians as monolithic and compares them with other racial and ethnic groups.
“You have to be polished. There’s no room for failure. There’s no room for imperfections. You have to be well-spoken, well-educated, have the right opinions, be good-looking, be tall. [You] have to have a family structure. There’s no room for any sort of freedom in identity except for the mold that you’ve been painted as – as a model citizen.”
–U.S.-born man of Pakistani origin in early 30s
“As an Asian person, I feel like there’s a stereotype that Asian students are high achievers academically. They’re good at math and science. … I was a pretty mediocre student, and math and science were actually my weakest subjects, so I feel like it’s either way you lose. Teachers expect you to fit a certain stereotype and if you’re not, then you’re a disappointment, but at the same time, even if you are good at math and science, that just means that you’re fitting a stereotype. It’s [actually] your own achievement, but your teachers might think ‘Oh, it’s because they’re Asian,’ and that diminishes your achievement.”
–U.S.-born woman of Korean origin in late 20s
“The model minority myth … mak[es] us as Asians [and] South Asians monoliths. … I’ve had people go, ‘Oh, so your dad’s a doctor? Is he a lawyer? Do you have money? Do you have this? Do you have that? Are you [in] an arranged marriage?’ And just the kind of image that portrays and gives us. But the expectations put on us as being high performing and everyone assumes you’re going to be smart. … I am a black sheep in many ways, not only within my family, but within Asian [and] South Asian culture, being [in my profession], someone who’s not a doctor, who hasn’t gone the professional, traditional, educational route. So, it’s very harmful, that too, for those communities within the Asian diaspora who have come to the United States. … [M]any of them come from impoverished and underrepresented communities and the expectations put on them to produce or the types of jobs and menial labor they have to take on as a result is really a very poisonous mythos to have out there.”
–U.S.-born woman of Indian origin in early 40s
“One of the reasons the model minority fallacy works so well as an argument against affirmative action [for Indians is] they are a newer immigrant group that has come here and … [t]here’s a lot of education [in India]. People have opportunity there that then they can come [to America] and continue with those connections. Whereas Blacks and Hispanics have had generations of oppression, so they don’t have anything to build off of. So when you bucket everybody – Black, Hispanics and Asians – into one group, then you can make those arguments of, ‘Oh, [Asians] are the model minority, they can do it.’”
Some participants talked about having mixed feelings about being called the “model minority” and how they felt like it put them in a kind of “middle ground.”
“I feel like Asians are kind of known as the model minority. That kind of puts us in an interesting position where I feel like we’re supposed to excel and succeed in the media, or we’re seen in the media as exceeding in all these things as smart. All of us are not by any means. Yeah, I feel like we’re in this weird middle ground.”
–U.S.-born man of Chinese origin in early 20s
“A lot of people believe that Japanese are the most humble and honest people, even among other Asians. I feel like I need to live up to that. I have to try hard when people say things like that. Of course, it is good, but it’s a lot of work sometimes. As Japanese, and for my family, I try hard.”
–Immigrant man of Japanese origin in mid-40s (translated from Japanese)
Others had more positive impressions of the model minority label, saying it made them proud to be Asian and have others see them that way:
“Whenever I apply for any job, in the drop-down there is an option to choose the ethnicity, and I write Asian American proudly because everyone knows us Asians as hardworking, they recognize us as loyal and hardworking.”
–Immigrant woman of Nepalese origin in mid-40s (translated from Nepali)
“I think any model is a good thing. I mean the cognitive, the word ‘model,’ when you model after somebody it’s a positive meaning to it. So personally for me I have no issues with being called the model minority because it only tells me that I’m doing something right.”
–U.S.-born man of Hmong origin in early 40s
- Some of these groups had relatively small sample sizes. For shares of Asian adults who have heard of the term “model minority” and say using the term to describe the U.S. Asian population is a good or bad thing, by education and income, refer to the Appendix . ↩
Sign up for our weekly newsletter
Fresh data delivery Saturday mornings
Sign up for The Briefing
Weekly updates on the world of news & information
- Asian Americans
- Discrimination & Prejudice
- Immigration Issues
- Race Relations
- Racial Bias & Discrimination
Asian Americans, Charitable Giving and Remittances
Key facts about asian americans living in poverty, methodology: 2023 focus groups of asian americans, 1 in 10: redefining the asian american dream (short film), the hardships and dreams of asian americans living in poverty, most popular, report materials.
1615 L St. NW, Suite 800 Washington, DC 20036 USA (+1) 202-419-4300 | Main (+1) 202-857-8562 | Fax (+1) 202-419-4372 | Media Inquiries
Research Topics
- Age & Generations
- Coronavirus (COVID-19)
- Economy & Work
- Family & Relationships
- Gender & LGBTQ
- Immigration & Migration
- International Affairs
- Internet & Technology
- Methodological Research
- News Habits & Media
- Non-U.S. Governments
- Other Topics
- Politics & Policy
- Race & Ethnicity
- Email Newsletters
ABOUT PEW RESEARCH CENTER Pew Research Center is a nonpartisan fact tank that informs the public about the issues, attitudes and trends shaping the world. It conducts public opinion polling, demographic research, media content analysis and other empirical social science research. Pew Research Center does not take policy positions. It is a subsidiary of The Pew Charitable Trusts .
Copyright 2024 Pew Research Center
Terms & Conditions
Privacy Policy
Cookie Settings
Reprints, Permissions & Use Policy
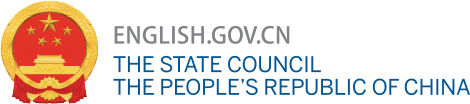
- INSTITUTIONS

BEIJING, April 27 -- Vidu, a text-to-video large AI model capable of creating a 16-second, high-definition video in 1080p resolution with a single click, was unveiled on Saturday at the 2024 Zhongguancun Forum in Beijing.
Developed by Tsinghua University and Chinese AI firm ShengShu Technology, Vidu is China's first video large AI model with "extended duration, exceptional consistency and dynamic capabilities."
As a large AI model developed in China, Vidu is able to understand and generate Chinese content such as the panda and the loong, or the Chinese dragon, according to Zhu Jun, deputy director of the Tsinghua Institute for Artificial Intelligence.
The company said that Vidu's core architecture was proposed as early as in 2022.
Copyright© www.gov.cn | About us | Contact us
Website identification code bm01000001 registration number: 05070218, all rights reserved. the content (including but not limited to text, photo, multimedia information, etc) published in this site belongs to www.gov.cn., without written authorization from www.gov.cn, such content shall not be republished or used in any form., copyright© www.gov.cn | contact us, website identification code bm01000001, registration number: 05070218.

IMAGES
COMMENTS
In the gravity model, this primarily relies on private car travel time and excludes travel times via other modes like public transit. This leads to a broader distribution of trips. Limited consideration of socio-economic and cultural factors: Another drawback of the gravity model is its neglect of certain socio-economic or cultural factors.
The number and definition of trip purposes in a model depend on the types of information the model needs to provide for planning analyses, the char- acteristics of the region being modeled, and the availability of data with which to obtain model parameters and the inputs to the model. ... (Travel Model Improvement Program, 2009). Feedback of ...
The TPB, like virtually all U.S. metropolitan areas, makes use of a trip-based travel demand model, which is often called a "four-step model," due to the four major steps (shown below). An alternate approach to the trip-based model (TBM) is the activity-based model (ABM). Although academics and researchers have been studying ABMs for about ...
Travel demand models are a subset of transportation models that specifically focus on — you guessed it — travel demand. They are commonly developed within a region or, more broadly, across an entire state. The regional "demand" for trip-making, informed by land use and economic conditions, and the "supply" of the transportation ...
Travel demand is derived from demand for activities. Tours are interdependent. People face time and space constraints that limit their activity schedule choice. Activity and travel scheduling decisions are made in the context of a broader framework. Conditioned by outcomes of longer term processes.
3) If the interzonal travel cost (from Suburbia to Urbia or Urbia to Suburbia) is 10 minutes, and the intrazonal travel cost (trips within Suburbia or within Urbia) is 5 minutes, use a (doubly-constrained) gravity model wherein the impedance is \(f(t_{ij})=t_{ij}^{-2}\), calculate the impedance, balance the matrix to match trip generation, and ...
The number and definition of trip purposes in a model depends on the types of informa- tion the model needs to provide for planning analyses, the characteristics of the region being modeled, and the avail- ability of data with which to obtain model parameters and the inputs to the model. ... If an analyst wishes to model the travel of younger ...
Activity-Based Travel Demand Models. A Primer. S2-C46-RR-1. SHRP 2 TRB. Activity-Based Travel Demand Models: A Primer. 029353 SHRP2 Activity-Based Final with No Mailer.indd 1 2/13/15 1:16 PM. TRANSPORTATION RESEARCH BOARD 2015 EXECUTIVE COMMITTEE* OFFICERS. Chair: Daniel Sperling,
The Travel Model Validation and Reasonableness Checking Manual, Second Edition (Cambridge Systematics, Inc., 2010b) includes detailed discussions of other transit network check- ing methods, including comparing modeled paths to observed paths from surveys and assigning a trip table developed from an expanded transit survey to the transit ...
This chapter describes methods and applications of travel demand forecasting techniques. It focuses on urban applications. The chapter discusses the basic principles, including a review of the commonly used four-step modeling paradigm, forecasting Transportation Demand Management (TDM), impacts and how forecasts can be applied to traffic impact analyses (TIAs).
Chapter Overview. The previous chapter introduces the four-step travel demand model (FSM), provides a real-world application, and outlines the data required to carry out each of the model steps. Chapter 10 focuses on the first step of the FSM, which is trip generation. This step involves predicting the total number of trips generated by each ...
Model calibration is the adjustment of constants and other model parameters in estimated or asserted models in an effort to make the models replicate observed data for a base year or otherwise produce more reasonable results. Model validation is the application of the calibrated models and comparison of the results against observed data ...
Over 30 years have passed since activity-based travel demand models (ABMs) emerged to overcome the limitations of the preceding models which have dominated the field for over 50 years. Activity-based models are valuable tools for transportation planning and analysis, detailing the tour and mode-restricted nature of the household and individual travel choices. Nevertheless, no single approach ...
The travel cost model (TCM) is the revealed preference method used in this context. ... Site definition (Step 3) is obviously only for one site, and site characteristic data (Step 6) are typically not gathered. In instances where several single-site models are being "stacked" in estimation, analysts will often gather site characteristic ...
Chapter Overview. Chapter 13 presents trip assignment, the last step of the Four-Step travel demand Model (FSM). This step determines which paths travelers choose for moving between each pair of zones. Additionally, this step can yield numerous results, such as traffic volumes in different transportation corridors, the patterns of vehicular ...
The Version 2.3 Travel Model estimates both motorized person trips and non-motorized person trips. Motorized person trips include both those made in private vehicles (e.g., cars, vans, trucks) and those made in public transit (e.g., buses, subways, commuter rail trains). Non-motorized person trips include walk and bike/bicycle trips.
Quality model validation must test all steps of the travel model and also should test model sensitivity. If standards are set for models by agencies or model reviewers, it is beneficial that they not convey a formal definition of acceptance but, rather to help set boundaries or levels of confidence regarding the use of travel forecasts for studies.
89 Chapter 6 Emerging Modeling Practices 90 6.1 The Activity-Based Approach 92 6.2 Activity-Based Travel Model Systems in Practice 96 6.3 Integration with Other Model Systems 99 6.4 Summary 100 Chapter 7 Case Studies 100 7.1 Introduction 100 7.2 Model Reasonableness Check 108 7.3 Model Development Case Study for a Smaller Area without Data for ...
The travel cost model is used to value recreational uses of the environment. For example, it may be used to value the recreation loss associated with a beach closure due to an oil spill or to value the recreation gain associated with improved water quality on a river. The model is commonly applied in benefit-cost analyses and in natural ...
Activity-based models are uniquely suited to: Permitting a continuous distribution of value-of- travel-time-savings across the population; essential for highway pricing evaluations. A clearer accounting of the full range of costs and utilities associated with both travel and activity by individual persons; improves transit mode choice estimation.
Section 1. Short Title. This Act shall be known as the "Travel Insurance Model Act.". Section 2. Scope and Purposes. A. The purpose of this Act is to promote the public welfare by creating a comprehensive legal framework within which Travel Insurance may be sold in this state. B.
Travel experience plays a driver role for changing travel motivations which has crucial part in. TCP model. Travel experience is the collective changes in an individual's perspective about self and environment due to tourism activities and events that individual passes through in course of travelling.
After discussing the definition of a travel agency, let's examine the general business model. Travel agents often operate in one of two ways. The first is through getting paid by the businesses ...
Immigrant generation: Among immigrants, 43% of 1.5-generation Asian adults say using the term "model minority" is a bad thing, compared with 26% of first-generation Asian adults. Age: Asian adults under 30 are far more likely to say the model minority label is a bad thing than a good thing (66% vs. 8%).
Rapid Access Computing Environment (RACE) is a United States federal government infrastructure-as-a-service ( IaaS ) initiative for developing and testing new Department of Defense (DoD) software applications. RACE is managed by the Defense Information Systems Agency (DISA), a government service provider that supplies and supports battlefield ...
BEIJING, April 27 -- Vidu, a text-to-video large AI model capable of creating a 16-second, high-definition video in 1080p resolution with a single click, was unveiled on Saturday at the 2024 Zhongguancun Forum in Beijing. Developed by Tsinghua University and Chinese AI firm ShengShu Technology, Vidu is China's first video large AI model with ...