

Compare Public Transport Connectivity In Europe
2 minute read
By: evelina bezubec
What is public transport density?
We’ve created a way to identify how public transport friendly a country is. The purple highlights all the areas of a country that can reach some sort of public transport service.
How we built the maps
- We used our own public transport database to identify the lat/long of each public transit stop
- We drew a 15 minute walking catchment area around each stop to identify the other surrounding areas with easy access (using the TravelTime API)
- We then exported the SVG file using QGIS
How to read the map
The more purple the area is, the greater the public transport density of an area. The white areas show where public transport is not reachable.
Where did we get the data from?
Our public transport model consolidates the timetables from thousands of different providers and agencies to produce an overall model of public transport for a country. At last count we had over 3m public transport stops and stations worldwide.
We include all combinations of public transport including train, bus, coach, metro, tram, and ferry. Walking is also included for all public transport journeys.
Our in-house data team updates this data globally at least every two weeks, so it is always right up-to-date with changes to timetables and services.
Explore our transport data in more detail
What can it be used for?
- Public transport network planning and development
- Travel plans - how easily can tourists explore the country using public transport
- Predicting commuting/travel habits in a local area
- Identifying the cause of road network congestion
We have created one of the largest public transport databases not only for the given but also for 100+ countries worldwide. Explore our data further:
- Create your own travel time catchment area : see where's reachable within a travel time limit
- Try our API Playground : are you a developer? Explore the code behind our API
- Explore our products : see what's possible with TravelTime
Calculate thousands of travel times with the TravelTime API
Building a transport system that works: Five insights from our 25-city report
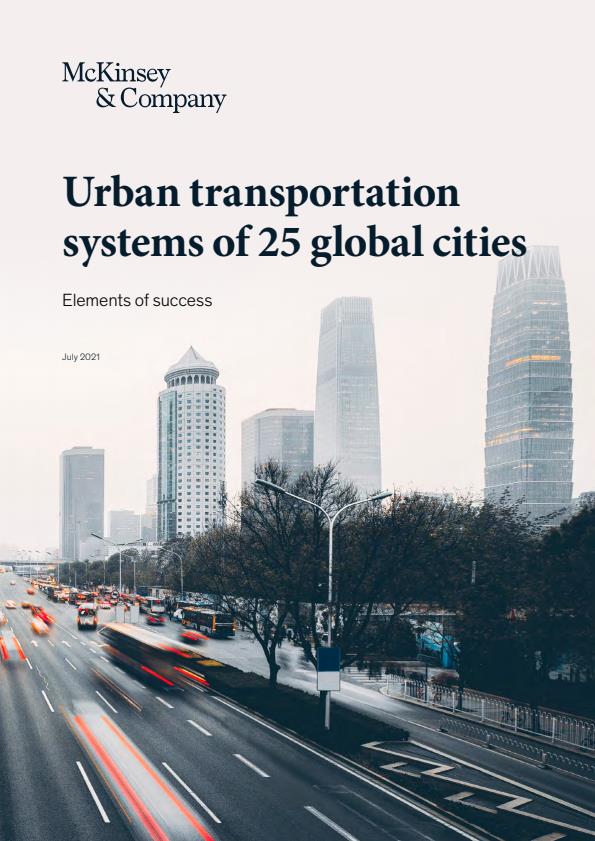
Elements of success: Urban transportation systems of 25 global cities
A city’s transportation network is its cardiovascular system—a multifaceted structure that enables the continuous flow of people and goods through its arteries. Municipal authorities, city councils, urban planners, and transport-infrastructure owners and operators around the world are well aware that its quality and efficiency are crucial for the economy and for the well-being of citizens.
The research methodology
Our analysis of the performance and efficiency of transport systems uses a methodology similar to the one we used for our 2018 report , but we should not draw direct comparisons between the two. We tweaked our metrics and drew from different sources of information to derive this year’s results.
Instead of providing one general ranking for all the cities, we found it more useful to rank the cities according to specific indicators, such as public-transport efficiency and affordability (exhibit). We ranked the cities and grouped them into three categories: leading (first to tenth place), contending (11th through 18th), and emerging (19th through 25th). We assessed the transportation network of each city for availability, affordability, efficiency, convenience, and safety and sustainable development, with separate ratings for public and personal transport use.
Cities at the bottom of the ratings table need to improve the availability of their transport infrastructure and expand electronic services, which have already become part and parcel of living in most of the examined cities (highlighted chart areas marked “a”). These aspects should be a top-priority task for any city that is improving its transport system.
To rise from the middle to the top of the ratings table, cities need to improve their efficiency and safety and sustainable-development performance. These aspects differentiate the leading cities from all others (highlighted chart areas marked “b”).
The ratings table used 50 different metrics for comparison, including road and rail networks, ticketing, airport flight routes, bicycle lanes, public transport, electronic-service availability, and environmental safety. The geospatial data collected are supplemented by opinions gathered from interviews with more than 30 transport-system-development experts, plus survey responses from 10,000 residents across 25 cities to gauge current satisfaction with existing transport systems and any changes that have been implemented. We have presented the findings in a series of easy-to-digest graphics alongside summaries of the individual transportation projects and the impact of the COVID-19 pandemic in all 25 cities. The report covers Buenos Aires, Chicago, Los Angeles, Mexico City, New York, São Paulo, and Toronto in the Americas; Berlin, Istanbul, London, Madrid, Milan, Moscow, Paris, and Saint Petersburg in Europe; Bangkok, Beijing, Hong Kong, Seoul, Shanghai, Shenzhen, Singapore, and Sydney in the Asia –Pacific (APAC) region, and Johannesburg in Africa.
To help stakeholders make informed decisions, we benchmarked the transport systems in 25 cities around the world in our latest report, Key elements of success in urban transportation systems (see sidebar “The research methodology”). We ranked the cities and grouped them into three categories: leading (first through tenth place), contending (11th through 18th), and emerging (19th through 25th).
All 25 cities have expanded projects to enhance their transport systems since 2018 (Exhibit 1). Leading cities invested more in improving the availability of their public-transport infrastructure, while emerging cities invested relatively less in safety and sustainability than in the other categories. As this article explains, such factors could have implications on residents’ willingness to use public transport.
While decision makers should delve into the full report for the complete rankings and details (see sidebar “Rankings at a glance: Top five cities by category”), this article distills the report’s findings into five key insights that stakeholders should pay attention to and highlights best-in-class practices in cities around the world.
Rankings at a glance: Top five cities by category
Here are the top-performing cities in each of the five categories:.
Availability—the variety of travel-mode options for residents
Affordability—the relative weight of costs associated with various transport modes
Efficiency—the speed and predictability of getting around the city
- Johannesburg
Convenience—the ease of transferring from one mode of transport to another
Safe and sustainable development—the level of safety of city travel and the environmental impact of the transport system
1. Keeping service and safety standards high assuages pandemic-related fears of using public transport
COVID-19 lockdown restrictions clearly had an impact on lifestyles and commuting patterns in 2020. Many people stopped traveling to work completely. People who relied on private cars, as well as those who used public transport, actually increased their use of private cars, even as the overall number of trips dipped (Exhibit 2). And in some cities, staff shortages and declining revenue from lower passenger usage led to reductions in service frequencies to avoid fare increases.
If these trends persist postpandemic, they are likely to exacerbate traffic congestion, pollution, and the number of traffic accidents. As such, public-transport operators and authorities will need to find ways to restore confidence in shared modes of getting around and reduce reliance on private cars. Our research found that the safer people feel about using public transport, the more they’ll use it (Exhibit 3), which suggests that the visibility of pandemic-related safety measures has a significant influence on perceived risks.
In Chinese cities, there is a lower perceived risk of infection on public-transport systems, thanks to a mandatory mask mandate, physical-distancing mandate, regular disinfection, and other epidemiological safety measures that citizens visibly adhere to. These measures are stepped up as needed (for instance, when sporadic outbreaks occur), and commuters may have to present a green health code and have their temperatures taken before entering public-transport areas. As a result, Chinese cities also experienced higher-than-average levels of public-transport mobility during the pandemic.
2. Expanding transport networks and infrastructure, as well as smart policies, keep travel options available and affordable
The top-scoring cities in transport availability—London, Madrid, and Paris—share some common characteristics: they are major railway hubs and offer good road networks, bike lanes, and pedestrian infrastructure. Beijing, Madrid, and Moscow jumped up in the transport-availability rankings by expanding their metro and rail lines. These cities also improved their road infrastructure, increased the number of bicycle lanes and pedestrian streets, and invested heavily in shared-transport schemes such as rental-bike and ride-sharing services (Exhibit 4).
Madrid’s bike-share system consists of 3,000 bicycles and 250 rental stations, with 50 rental stations added in 2020 alone. Since our last urban-transport report, in 2018, Moscow added 3,000 two-wheelers to its bike-share program. It also opened new underground lines, resulting in 700,000 more people gaining access to the Moscow Metro, while Beijing opened three new underground lines over the past several years.
Public policies play a critical role in keeping transport affordable, whether it’s by regulating low bus and subway fares or by encouraging competition between legacy transport operators and ride-sharing companies. High rates of private-car ownership tend to constrict revenue flows for the public-transport system because fewer people use public transport. Thus, policies that discourage private-car ownership tend to prevent public-transport operators from either raising fares or reducing service standards.
The Asian cities of Seoul, Shenzhen, and Singapore, for example, top the rankings for public-transport affordability, and to offset the environmental and societal costs of personal car use, these cities actively make car ownership a more expensive choice.
Public-transport systems in Buenos Aires, Mexico City, and Shanghai are also becoming much more affordable because of government policies stimulating economic competition and technology. Cars registered outside Shanghai are barred from certain districts, and technologies for self-driving taxis are being piloted, which may lead to lower costs in the future. Commuters enjoy the benefit of lower fares, the result of competition among multiple ride-share providers. The widespread implementation of paid parking systems in Buenos Aires and Mexico City is making private-car ownership more expensive. With more people turning to public transport or ride-sharing over private cars to avoid incurring parking costs, there are fewer vehicles on the road, which eases traffic congestion.
3. Dedicated public-transport lanes and digitalization can make the commuter experience more efficient and convenient
Efficiency refers to how quickly and predictably one can move around the city, while convenience measures how easily commuters can switch from one mode of transport to another. Increasing the number of dedicated public-transport lanes, optimizing bus routes, completing road construction or modernization projects, and implementing digital upgrades all help improve the commuter experience.
Moscow, Shenzhen, and Singapore all scored high on transport efficiency. The Russian capital’s transport system has low underground waiting times, high speeds during rush hour, and a significantly above-average proportion of dedicated bus lanes. Shenzhen, too, has a high share of dedicated bus lanes, which helps with rush-hour predictability. Singapore’s electronic road-pricing system is powered by a digital device that automatically charges the driver the road toll when the car passes through a gantry, enabling frictionless road travel for both private and public vehicles, even during peak times.
Our convenience index assesses the ease of switching from one transport mode to another. High performers have invested in upgrading their ticketing systems, increasing internet access, and increasing the number of wheelchair-accessible buses and underground stations. Some offer convenient mobility-as-a-service applications (MaaS) to plan routes and to verify and pay fines and penalties.
Toronto delivers high levels of travel comfort, courtesy of a $934 million upgrade of its bus fleet, which is now 100 percent wheelchair friendly and located closer to subway stations. Hong Kong has also revamped its public-transport system. Ninety out of 93 metro stations have been outfitted with elevators and wheelchair ramps, making it easier and quicker for wheelchair-bound passengers to board and disembark. Meanwhile, Istanbul has risen in the convenience rankings with a significantly improved ticketing system using QR-code payments. The city has also introduced the Ulasim Asistani app, which helps travelers plan journeys across multiple forms of transport, leading to a considerable improvement in satisfaction ratings among its citizens.
4. Sustainability matters—in both investment and policy
Both commuter safety and the environment cannot be neglected in a city’s efforts to improve its transport system. In both our 2018 and 2021 surveys, respondents cited safety as their number-one priority, so it’s imperative that city planners and authorities constantly look to minimize accidents and fatalities while reducing the city’s carbon footprint. As mentioned earlier, leading cities tend to invest more in sustainable mobility options than contending and emerging cities do, which has resulted in greater use of their public-transport systems (Exhibit 5).
Initiatives to ensure compliance with safety requirements matter, as do the implementation of more stringent restrictions on the use of petrol and diesel engines, measures to reduce pollution, and incentives to switch to electric vehicles.
Tokyo boasts one of the world’s lowest road-fatality levels—9.6 deaths per 1 million people. Over the past several years, the government has deployed the data-driven smart-transport system to monitor and analyze information on people’s commuting patterns and traffic violations to inform decision making. As a result, road fatalities have decreased and more people are complying with traffic rules. The government is also using new toll-management technology to decrease vehicle traffic and improve road safety.
In China, Beijing and Shanghai are aggressively curbing the negative environmental impact of their transport systems. Both cities mandated in 2021 that only vehicles that adhere to the China 6 emissions standards (roughly equivalent to the Euro 6 standard in the European Union) can be sold.
5. In some cases, better communication is needed to bridge gaps between perception and reality
We tracked how satisfied residents were with how their transport system is doing according to specific metrics and based on changes implemented since 2018. Residents appear to appreciate the hard work urban authorities have put into transport projects, but in a few cases, their perceptions may not be aligned with reality. For instance, most citizens feel that public transport is too expensive in their cities (Exhibit 6). So even though Seoul, for example, stands out as a leader in public-transport affordability based on objective metrics, its citizens remain dissatisfied.
This suggests that authorities need to keep the residents informed of all positive changes and continue their efforts to improve public perceptions. It is highly likely that additional restrictions on personal motor vehicles will be introduced in the coming years and that environmental regulations will become more stringent. To improve the public perception of such efforts, city authorities must not only score tangible successes but also clearly articulate them.
Our full progress report benchmarking the transport systems in 25 cities around the world investigates the five themes outlined in this article in greater depth, and includes other findings that are relevant to key stakeholders. Overall, while there’s reason to celebrate the many improvements in the majority of our metrics in cities around the world, there’s still much work to be done. Making informed decisions about the further development of city transport systems will help.
Dmitry Chechulin is an associate partner in McKinsey’s Moscow office, where Vadim Pokotilo is a partner. Detlev Mohr is a senior partner in the Tokyo office, and Lola Woetzel is a senior partner in the Shanghai office.
Explore a career with us
Related articles.
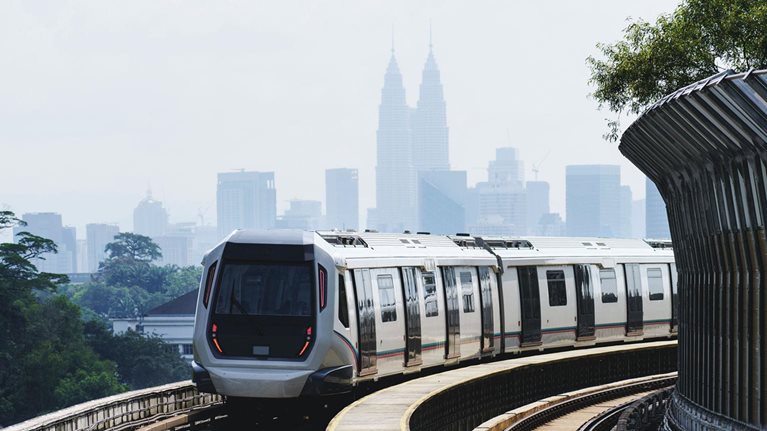
Transit investments in an age of uncertainty
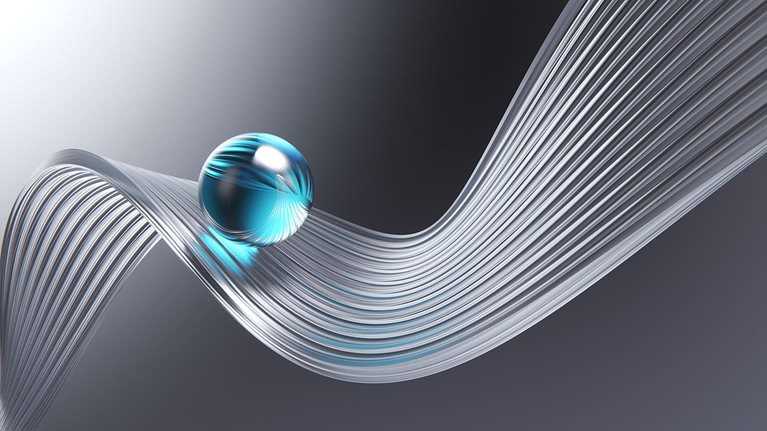
The road to seamless urban mobility
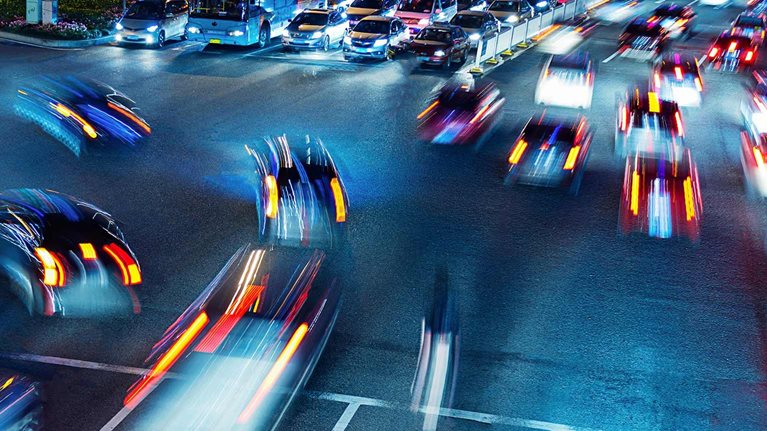
Elements of success: Urban transportation systems of 24 global cities
- Transportation & Logistics ›
Public Transportation & Mobility Services
Industry-specific and extensively researched technical data (partially from exclusive partnerships). A paid subscription is required for full access.
Street network area per car in biggest cities worldwide 2019
Street network area per car worldwide 2019, by city.
- Immediate access to 1m+ statistics
- Incl. source references
- Download as PNG, PDF, XLS, PPT
Additional Information
Show sources information Show publisher information Use Ask Statista Research Service
January 2020
France, Japan, Russia, Singapore, Spain, United Kingdom, United States
Other statistics on the topic
Rail Transport
Number of passenger journeys on the London Underground (UK) 2000-2023
Longest metro networks in European cities in 2023
Politics & Government
Government spending on public transport in the UK 2009-2023
Passenger journeys on the Manchester Metrolink in the United Kingdom 1992–2023
To download this statistic in XLS format you need a Statista Account
To download this statistic in PNG format you need a Statista Account
To download this statistic in PDF format you need a Statista Account
To download this statistic in PPT format you need a Statista Account
As a Premium user you get access to the detailed source references and background information about this statistic.
As a Premium user you get access to background information and details about the release of this statistic.
As soon as this statistic is updated, you will immediately be notified via e-mail.
… to incorporate the statistic into your presentation at any time.
You need at least a Starter Account to use this feature.
- Immediate access to statistics, forecasts & reports
- Usage and publication rights
- Download in various formats
You only have access to basic statistics. This statistic is not included in your account.
- Instant access to 1m statistics
- Download in XLS, PDF & PNG format
- Detailed references
Business Solutions including all features.
Other statistics that may interest you
- Budget allocation for roads and highways development India FY 2017-2024
- Average commute distance in China 2021, by city size
- Breakdown of public transport passengers in urban China 2021, by type
- Number of ferry passengers in China's cities 2021, by city
- Growth rate of ferry passenger numbers in China's cities 2019-2021, by city
- Transportation fatalities by mode in the United States 2021
- Complaints on cleanliness of public transport in Hong Kong Q2 2022
- Complaints per million passenger journey of bus services in Hong Kong Q2 2022
- Complaints on frequency or carrying capacity of public transport in Hong Kong Q2 2022
- Complaints on routing of public transport in Hong Kong Q2 2022, by type
- France: employees in the transportation & storage industry 2012-2021
- France: number of enterprises in the transportation and storage industry 2013-2022
- Fleet size of demand responsive transport in European countries 2019
- E-bikes sold in France 2005-2022
- Public investments in transportation infrastructures in France 2007-2020, by type
- France: road fatalities by mode of transportation 2021
- Revenue of the public transportation operator RATP in Paris, France 2011-2021
- Number of public transport trips in Paris and Île-de-France, by mode 2019-2020
- Closed-circuit television implementation rate in public transport in France 2011-2020
- Index of change in the number of weekly trips made by Vélib' service user France 2020
- Global GHG emissions from transportation 1990-2020
- Number of transportation and storage enterprises South Korea 2021, by sector
- Number of taxi rides in Russia 2015-2021
- Overseas business visitors in the U.S. 2019, by transportation used
- Mexico: passenger transport buses 2019, by model
- Chile: number of companies in the taxi sector 2005-2015
- Denmark: turnover of the transportation and storage industry 2013-2022
- Hungary: number of enterprises in the transportation and storage industry 2013-2022
- Austria: personnel costs of the transportation and storage industry 2012-2021
- BRI for Hong Kong's transportation industry 2013-2023
- Road freight volume in Russia 2022, by federal district
- Profit/loss before tax of transport organizations in Russia 2022, by sector
- Number of new and used EVs in Russia 2013-2023
- Top 10 regions by volume of passenger cars with mileage in Russia 2022
- Cargo transported through Northern Sea Route in Russia 2011-2022
- Number of EVs in the Russian car market 2022, by model
- Leading truck car brands in Russia 2022-2023, by sales volume
- Volume of passengers in Russia 2022-2023, by transportation mode
- Passenger turnover in public transport in Russia 2022-2023, by transportation mode
- Rail freight volume in Russia 2023, by commodity
Other statistics that may interest you Statistics on
About the industry
- Premium Statistic Budget allocation for roads and highways development India FY 2017-2024
- Premium Statistic Average commute distance in China 2021, by city size
- Premium Statistic Breakdown of public transport passengers in urban China 2021, by type
- Premium Statistic Number of ferry passengers in China's cities 2021, by city
- Premium Statistic Growth rate of ferry passenger numbers in China's cities 2019-2021, by city
- Premium Statistic Transportation fatalities by mode in the United States 2021
- Premium Statistic Complaints on cleanliness of public transport in Hong Kong Q2 2022
- Premium Statistic Complaints per million passenger journey of bus services in Hong Kong Q2 2022
- Premium Statistic Complaints on frequency or carrying capacity of public transport in Hong Kong Q2 2022
- Premium Statistic Complaints on routing of public transport in Hong Kong Q2 2022, by type
About the region
- Basic Statistic France: employees in the transportation & storage industry 2012-2021
- Basic Statistic France: number of enterprises in the transportation and storage industry 2013-2022
- Premium Statistic Fleet size of demand responsive transport in European countries 2019
- Basic Statistic E-bikes sold in France 2005-2022
- Premium Statistic Public investments in transportation infrastructures in France 2007-2020, by type
- Premium Statistic France: road fatalities by mode of transportation 2021
- Premium Statistic Revenue of the public transportation operator RATP in Paris, France 2011-2021
- Premium Statistic Number of public transport trips in Paris and Île-de-France, by mode 2019-2020
- Premium Statistic Closed-circuit television implementation rate in public transport in France 2011-2020
- Premium Statistic Index of change in the number of weekly trips made by Vélib' service user France 2020
Other regions
- Premium Statistic Global GHG emissions from transportation 1990-2020
- Premium Statistic Number of transportation and storage enterprises South Korea 2021, by sector
- Premium Statistic Number of taxi rides in Russia 2015-2021
- Premium Statistic Overseas business visitors in the U.S. 2019, by transportation used
- Premium Statistic Mexico: passenger transport buses 2019, by model
- Premium Statistic Chile: number of companies in the taxi sector 2005-2015
- Basic Statistic Denmark: turnover of the transportation and storage industry 2013-2022
- Basic Statistic Hungary: number of enterprises in the transportation and storage industry 2013-2022
- Basic Statistic Austria: personnel costs of the transportation and storage industry 2012-2021
- Premium Statistic BRI for Hong Kong's transportation industry 2013-2023
Related statistics
- Premium Statistic Road freight volume in Russia 2022, by federal district
- Premium Statistic Profit/loss before tax of transport organizations in Russia 2022, by sector
- Premium Statistic Number of new and used EVs in Russia 2013-2023
- Premium Statistic Top 10 regions by volume of passenger cars with mileage in Russia 2022
- Premium Statistic Cargo transported through Northern Sea Route in Russia 2011-2022
- Premium Statistic Number of EVs in the Russian car market 2022, by model
- Basic Statistic Leading truck car brands in Russia 2022-2023, by sales volume
- Premium Statistic Volume of passengers in Russia 2022-2023, by transportation mode
- Premium Statistic Passenger turnover in public transport in Russia 2022-2023, by transportation mode
- Premium Statistic Rail freight volume in Russia 2023, by commodity
Further Content: You might find this interesting as well
Thank you for visiting nature.com. You are using a browser version with limited support for CSS. To obtain the best experience, we recommend you use a more up to date browser (or turn off compatibility mode in Internet Explorer). In the meantime, to ensure continued support, we are displaying the site without styles and JavaScript.
- View all journals
- Explore content
- About the journal
- Publish with us
- Sign up for alerts
- Published: 11 November 2019
How transit scaling shapes cities
- Hao Wu ORCID: orcid.org/0000-0002-5526-8827 1 ,
- David Levinson ORCID: orcid.org/0000-0002-4563-2963 1 &
- Somwrita Sarkar 1
Nature Sustainability volume 2 , pages 1142–1148 ( 2019 ) Cite this article
1909 Accesses
26 Citations
23 Altmetric
Metrics details
- Engineering
- Sustainability
Transit accessibility to jobs (the ease of reaching a place of work by public transport) affects both residential location and commute mode choice, resulting in gradations of residential land-use intensity and transit (public transport) patronage. We propose a scaling model explaining much of the variation in transit use—the number of transit commuters per km 2 —and residential land-use intensity with transit accessibility. We find that locations with high transit accessibility consistently have more riders and higher residential density; transit systems that provide greater accessibility and with a larger base for patronage have proportionally greater ridership increase per unit of accessibility. All 48 metropolitan statistical areas in our sample have a scaling factor less than 1, so a 1% increase in access to jobs produces a less than 1% increase in transit riders; the largest cities therefore have higher scaling factors than smaller cities, indicating returns to scale. The models, derived from a new database of transit accessibility measured for every minute of the peak period over 11 million US census-blocks, and estimated for 48 major cities across the United States, find that the number of jobs reachable within 45 minutes of the rider’s base most affect transit rider density. The findings support the idea that transit investment should focus on mature, well-developed regions.
This is a preview of subscription content, access via your institution
Access options
Access Nature and 54 other Nature Portfolio journals
Get Nature+, our best-value online-access subscription
24,99 € / 30 days
cancel any time
Subscribe to this journal
Receive 12 digital issues and online access to articles
111,21 € per year
only 9,27 € per issue
Buy this article
- Purchase on Springer Link
- Instant access to full article PDF
Prices may be subject to local taxes which are calculated during checkout
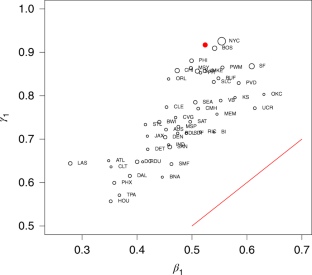
Similar content being viewed by others
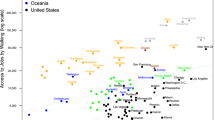
Urban access across the globe: an international comparison of different transport modes
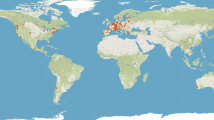

Access to mass rapid transit in OECD urban areas
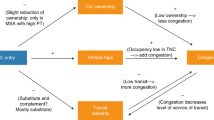
Impacts of transportation network companies on urban mobility
Data availability.
The transit accessibility data are obtained from the Accessibility Observatory at the University of Minnesota. Block-group accessibility measures are population weighted averages of constituent block values to match the spatial reporting of the mode share data from the US Census Bureau. Working population characteristics come from the 2016 American Community Survey and Longitudinal Employer-Household Dynamics programme’s 2015 Origin-Destination Employment Statistics 65 , 66 . Data on mode share are obtained from the 2016 American Community Survey 5 yr estimates which describes commute mode choice between 2012 and 2016. Transit is defined to include walking, ferry, rail (subway and commuter), and bus, trolley and streetcar. Data described in this section are available via public websites.
West, G. B., Brown, J. H. & Enquist, B. J. A general model for the origin of allometric scaling laws in biology. Science 276 , 122–126 (1997).
Article CAS Google Scholar
Louf, R. & Barthelemy, M. How congestion shapes cities: from mobility patterns to scaling. Sci. Rep. 4 , 5561 (2014).
Depersin, J. & Barthelemy, M. From global scaling to the dynamics of individual cities. Proc. Natl Acad. Sci. USA 115 , 2317–2322 (2018).
Samaniego, H. & Moses, M. E. Cities as organisms: allometric scaling of urban road networks. J. Transp. Land Use 1 , 21–39 (2008).
Article Google Scholar
Levinson, D. Network structure and city size. PLoS ONE 7 , e29721 (2012).
Bettencourt, L. M. The origins of scaling in cities. Science 340 , 1438–1441 (2013).
Merlin, L. A. A portrait of accessibility change for four US metropolitan areas. J. Transp. Land Use 10 , 309–336 (2017).
Google Scholar
Jones, C. & Kammen, D. M. Spatial distribution of us household carbon footprints reveals suburbanization undermines greenhouse gas benefits of urban population density. Environ. Sci. Technol. 48 , 895–902 (2014).
Sovacool, B. K. & Brown, M. A. Twelve metropolitan carbon footprints: a preliminary comparative global assessment. Energy Policy 38 , 4856–4869 (2010).
Marshall, J. D. Reducing urban sprawl could play an important role in addressing climate change. Environ. Sci. Technol. 42 , 3133–3137 (2008).
Bruun, E. & Givoni, M. Sustainable mobility: six research routes to steer transport policy. Nat. News 523 , 29–31 (2015).
Song, S. Modelling worker residence distribution in the Los Angeles region. Urban Stud. 31 , 1533–1544 (1994).
Weisbrod, G., Lerman, S. R. & Ben-Akiva, M. Tradeoffs in residential location decisions: Transportation versus other factors. Transp. Policy Decis. Mak. 1 , 13–26 (1980).
Zondag, B. & Pieters, M. Influence of accessibility on residential location choice. Transp. Res. Rec. 1902 , 63–70 (2005).
Schwanen, T. & Mokhtarian, P. L. What affects commute mode choice: neighborhood physical structure or preferences toward neighborhoods? J. Transp. Geogr. 13 , 83–99 (2005).
Ewing, R. & Cervero, R. Travel and the built environment: a synthesis. Transp. Res. Rec. 1780 , 87–114 (2001).
Cervero, R. Traditional neighborhoods and commuting in the San Francisco bay area. Transportation 23 , 373–394 (1996).
Renne, J. L., Hamidi, S. & Ewing, R. Transit commuting, the network accessibility effect, and the built environment in station areas across the United States. Res. Transp. Econ. 60 , 35–43 (2016).
Cervero, R., Murakami, J. & Miller, M. Direct ridership model of bus rapid transit in Los Angeles County, California. Transp. Res. Rec. 2145 , 1–7 (2010).
Sun, L., Jin, J. G., Axhausen, K. W., Lee, D.-H. & Cebrian, M. Quantifying long-term evolution of intra-urban spatial interactions. J. R. Soc. Inter. 12 , 20141089 (2015).
Levinson, D. M. Density and dispersion: the co-development of land use and rail in London. J. Econ. Geogr. 8 , 55–77 (2008).
Xie, F. & Levinson, D. M. How streetcars shaped suburbanization: a granger causality analysis of land use and transit in the twin cities. J. Econ. Geogr. 10 , 453–470 (2009).
King, D. A. Developing densely: Estimating the effect of subway growth on New York City land uses. J. Trans. Land Use 4 , 19–32 (2011).
Mayor, K., Lyons, S., Duffy, D. & Tol, R. S. A hedonic analysis of the value of rail transport in the Greater Dublin area. J. Transp. Econ. Policy 46 , 239–261 (2012).
Pan, Q. The impacts of an urban light rail system on residential property values: a case study of the Houston METRORail transit line. Transp. Plan. Technol. 36 , 145–169 (2013).
Grass, R. G. The estimation of residential property values around transit station sites in Washington, D.C. J. Econ. Finance 16 , 139–146 (1992).
Cervero, R. & Kang, C. D. Bus rapid transit impacts on land uses and land values in Seoul, Korea. Transp. Policy 18 , 102–116 (2011).
Giuliano, G., Gordon, P., Pan, Q. & Park, J. Accessibility and residential land values: some tests with new measures. Urban Stud. 47 , 3103–3130 (2010).
Diao, M. & Ferreira Jr, J. Residential property values and the built environment: empirical study in the Boston, Massachusetts metropolitan area. Transp. Res. Rec. 2174 , 138–147 (2010).
Bowes, D. R. & Ihlanfeldt, K. R. Identifying the impacts of rail transit stations on residential property values. J. Urban Econ. 50 , 1–25 (2001).
Glaeser, E. L., Kallal, H. D., Scheinkman, J. A. & Shleifer, A. Growth in cities. J. Polit. Econ. 100 , 1126–1152 (1992).
Ortman, S. G., Cabaniss, A. H., Sturm, J. O. & Bettencourt, L. M. Settlement scaling and increasing returns in an ancient society. Sci. Adv. 1 , e1400066 (2015).
Gallotti, R. & Barthelemy, M. Anatomy and efficiency of urban multimodal mobility. Sci. Rep. 4 , 6911 (2014).
Ermagun, A. & Levinson, D. Accessibility and Transit Performance Nexus Working Paper 000129 (University of Minnesota, Nexus Research Group, 2015).
Batty, M. Modelling cities as dynamic systems. Nature 231 , 425–428 (1971).
Makse, H. A., Havlin, S. & Stanley, H. E. Modelling urban growth patterns. Nature 377 , 608–612 (1995).
Chumak, A. & Braaksma, J. Implications of the travel time budget for urban transportation modelling in Canada. Transp. Res. 13 , 243–257 (1979).
Kawabata, M. & Shen, Q. Commuting inequality between cars and public transit: the case of the San Francisco bay area, 1990–2000. Urban Stud. 44 , 1759–1780 (2007).
American Community Survey Table B08301 (US Census Bureau, 2016); https://go.nature.com/2W6ywLU
American Community Survey Table B08136 (US Census Bureau, 2016); https://go.nature.com/33XkGhp
Huang, J., Levinson, D., Wang, J., Zhou, J. & Wang, Z. Tracking job and housing dynamics with smartcard data. Proc. Natl Acad. Sci. USA 115 , 12710–12715 (2018).
Clark, W. A., Huang, Y. & Withers, S. Does commuting distance matter?: commuting tolerance and residential change. Reg. Sci. Urban Econ. 33 , 199–221 (2003).
Mogridge, M. J. The self-defeating nature of urban road capacity policy: a review of theories, disputes and available evidence. Transp. Policy 4 , 5–23 (1997).
Smart, M. J. & Klein, N. J. Remembrance of cars and buses past: how prior life experiences influence travel. J. Plan. Educ. Res. 38 , 139–151 (2018).
Levinson, D. M. & Krizek, K. J. Metropolitan Transport and Land Use: Planning for Place and Plexus (Routledge, 2018).
Bar-Yosef, A., Martens, K. & Benenson, I. A model of the vicious cycle of a bus line. Transp. Res. 54 , 37–50 (2013).
Mohring, H. Optimization and scale economies in urban bus transportation. Am. Econ. Rev. 62 , 591–604 (1972).
Nelson, P. Information and consumer behavior. J. Polit. Econ. 78 , 311–329 (1970).
Owen, A. & Levinson, D. M. Modeling the commute mode share of transit using continuous accessibility to jobs. Transp. Res. Pt A 74 , 110–122 (2015).
Hansen, W. G. How accessibility shapes land use. J. Am. Instit. Plan. 25 , 73–76 (1959).
Ingram, D. R. The concept of accessibility: a search for an operational form. Reg. Stud. 5 , 101–107 (1971).
Wickstrom, G. V. Defining balanced transportation-a question of opportunity. Traffic Quart. 25 , 337–349 (1971).
Wachs, M. & Kumagai, T. G. Physical accessibility as a social indicator. Socio-econ. Plan. Sci. 7 , 437–456 (1973).
Merlin, L. A. & Hu, L. Does competition matter in measures of job accessibility? Explaining employment in Los Angeles. J. Transp. Geogr. 64 , 77–88 (2017).
Xu, W., Zhang, W. & Li, L. Measuring the expected locational accessibility of urban transit network for commuting trips. Transp. Res. Pt D 51 , 62–81 (2017).
Xu, W., Li, Y. & Wang, H. Transit accessibility for commuters considering the demand elasticities of distance and transfer. J. Trans. Geogr. 56 , 138–156 (2016).
Batty, M. Accessibility: in search of a unified theory. Environ. Plan. 36 , 191–194 (2009).
Xi, Y., Miller, E. J. & Saxe, S. Exploring the impact of different cut-off times on isochrone measurements of accessibility. Transp. Res. Rec. 2672 , 113–124 (2018).
Tobler, W. R. A computer movie simulating urban growth in the Detroit region. Econ. Geogr. 46 , 234–240 (1970).
Miller, H. J. Tobler’s first law and spatial analysis. Ann. Am. Assoc. Geogr. 94 , 284–289 (2004).
Geurs, K. T. & Ritsema van Eck, J. R. Accessibility measures: review and applications. evaluation of accessibility impacts of land-use transportation scenarios, and related social and economic impact. Environ. Plan . 30 , 69–87 (2001).
Reggiani, A., Bucci, P. & Russo, G. Accessibility and impedance forms: empirical applications to the german commuting network. Inter. Reg. Sci. Rev. 34 , 230–252 (2011).
Huang, A. & Levinson, D. Axis of travel: modeling non-work destination choice with gps data. Transp. Res. Pt C 58 , 208–223 (2015).
Levinson, D. & Kumar, A. Multi-modal trip distribution model. Transp. Res. Rec. 1466 , 124–131 (1994).
Horner, M. W. & Schleith, D. Analyzing temporal changes in land-use–transportation relationships: a LEHD-based approach. Appl. Geogr. 35 , 491–498 (2012).
Graham, M. R., Kutzbach, M. J. & McKenzie, B. Design Comparison of LODES and ACS Commuting Data Products Working Paper 14-38 (Center for Economic Studies, US Census Bureau, 2014).
Download references
Acknowledgements
We thank the Accessibility Observatory at the University of Minnesota for the provision of data.
Author information
Authors and affiliations.
University of Sydney, Sydney, New South Wales, Australia
Hao Wu, David Levinson & Somwrita Sarkar
You can also search for this author in PubMed Google Scholar
Contributions
D.L. created the study conception and design, H.W. and S.S. analysed and intepreted the results and H.W. prepared the manuscript.
Corresponding author
Correspondence to Hao Wu .
Ethics declarations
Competing interests.
The authors declare no competing interests.
Additional information
Publisher’s note Springer Nature remains neutral with regard to jurisdictional claims in published maps and institutional affiliations.
Supplementary information
Supplementary information.
Supplementary Figs. 1–2, Tables 1–2 and references.
Rights and permissions
Reprints and permissions
About this article
Cite this article.
Wu, H., Levinson, D. & Sarkar, S. How transit scaling shapes cities. Nat Sustain 2 , 1142–1148 (2019). https://doi.org/10.1038/s41893-019-0427-7
Download citation
Received : 18 December 2018
Accepted : 11 October 2019
Published : 11 November 2019
Issue Date : December 2019
DOI : https://doi.org/10.1038/s41893-019-0427-7
Share this article
Anyone you share the following link with will be able to read this content:
Sorry, a shareable link is not currently available for this article.
Provided by the Springer Nature SharedIt content-sharing initiative
This article is cited by
Energy and environmental impacts of shared autonomous vehicles under different pricing strategies.
- Shaopeng Zhong
npj Urban Sustainability (2023)
- Paolo Avner
- David Levinson
npj Urban Sustainability (2021)
City structure shapes directional resettlement flows in Australia
- Bohdan Slavko
- Kirill Glavatskiy
- Mikhail Prokopenko
Scientific Reports (2020)
Quick links
- Explore articles by subject
- Guide to authors
- Editorial policies
Sign up for the Nature Briefing newsletter — what matters in science, free to your inbox daily.

A systematic review on crowding valuation in public transport
- Original Research
- Published: 02 May 2024
Cite this article
- Rupam Fedujwar 1 &
- Amit Agarwal ORCID: orcid.org/0000-0002-3352-0227 1
In public transport, crowding is one of the variables that is likely to influence the decisions of the choice makers. Crowding has become a subject of concern in metropolitan areas, triggering passenger travel behavior, such as shifting from public to private modes of transport, changing routes or departure times, etc. Hence, there is a need to understand the effect of crowding in public transport and its influence on the behavior of travelers. Therefore, this review investigates essential factors (e.g., crowding representation, crowding measurement, modeling framework, etc.) after reviewing the 40 screened studies on the valuation of crowding in public transport. The paper’s findings show that the passenger perception towards crowding is different for varying levels of crowding, modes of transport, study areas, data types, different modeling frameworks, and the underlying distribution of the attribute parameters. A meta-analysis is performed to show the influence of explanatory variables affecting the value of the time multiplier. A net-salary-based city classification is used to make the results transferable. Lastly, this work provides a direction for the selection of the crowding representation, measure, and valuation for future studies. Further, several research gaps are identified for the model formulation, valuation, crowding at different locations, non-linearity, etc.
This is a preview of subscription content, log in via an institution to check access.
Access this article
Price includes VAT (Russian Federation)
Instant access to the full article PDF.
Rent this article via DeepDyve
Institutional subscriptions
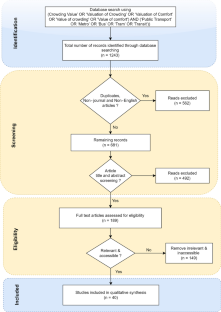
https://www.jabref.org/
The past studies have given the multiplier values for different crowding levels/densities. The range plots are drawn by retrieving the data from these studies.
The PPP rates are extracted from https://data.oecd.org/conversion/purchasing-power-parities-ppp.htm on Nov. 23, 2022.
For this, average annual US Consumer Price Index (CPI) data is used. This information is extracted from https://www.calculator.net/inflation-calculator.html on Nov. 23, 2022.
https://www.numbeo.com/cost-of-living/city_price_rankings?itemId=105
Aghabayk K, Esmailpour J, Shiwakoti N (2021) Effects of COVID-19 on rail passengers’ crowding perceptions. Transport Res Part A Policy Pract 154:186–202. https://doi.org/10.1016/j.tra.2021.10.011
Article Google Scholar
Bansal P, Hurtubia R, Tirachini A, Daziano RA (2019) Flexible estimates of heterogeneity in crowding valuation in the New York City subway. J Choice Model 31:124–140. https://doi.org/10.1016/j.jocm.2019.04.004
Basnak P, Giesen R, Muñoz JC (2022) Estimation of crowding factors for public transport during the COVID-19 pandemic in Santiago, Chile. Transport Res Part A Policy Pract 159:140–156. https://doi.org/10.1016/j.tra.2022.03.011
Basu D, Hunt JD (2012) Valuing of attributes influencing the attractiveness of suburban train service in Mumbai city: a stated preference approach. Transport Res Part A Policy Pract 46(9):1465–1476. https://doi.org/10.1016/j.tra.2012.05.010
Batarce M, Muñoz JC, de Dios Ortúzar J et al. (2015) Use of mixed stated and revealed preference data for crowding valuation on public transport in Santiago, Chile. Transport Res Rec J Transport Res Board 2535(1):73–78. https://doi.org/10.3141/2535-08
Batarce M, Muñoz JC, de Dios Ortúzar J (2016) Valuing crowding in public transport: implications for cost-benefit analysis. Transport Res Part A Policy Pract 91:358–378. https://doi.org/10.1016/j.tra.2016.06.025
Batley R, Bates J, Bliemer M et al. (2017) New appraisal values of travel time saving and reliability in great Britain. Transportation 46(3):583–621. https://doi.org/10.1007/s11116-017-9798-7
Björklund G, Swärdh JE (2017) Estimating policy values for in-vehicle comfort and crowding reduction in local public transport. Transport Res Part A Policy Pract 106:453–472. https://doi.org/10.1016/j.tra.2017.10.016
Cats O, West J, Eliasson J (2016) A dynamic stochastic model for evaluating congestion and crowding effects in transit systems. Transport Res Part B Methodol 89:43–57. https://doi.org/10.1016/j.trb.2016.04.001
Çelebi D, İmre Ş (2020) Measuring crowding-related comfort in public transport. Transport Plan Technol 43(7):735–750. https://doi.org/10.1080/03081060.2020.1805546
Cho SH, Park HC (2021) Exploring the behaviour change of crowding impedance on public transit due to COVID-19 pandemic: before and after comparison. Transport Lett 13(5–6):367–374. https://doi.org/10.1080/19427867.2021.1897937
Gao K, Sun L, Tu H, Li H (2018) Heterogeneity in valuation of travel time reliability and in-vehicle crowding for mode choices in multimodal networks. J Transport Eng Part A Syst 144(10):04018,061. https://doi.org/10.1061/jtepbs.0000187
Harzing AW (2020) Publish or perish. https://harzing.com/resources/publish-or-perish . Accessed 2020
Haywood L, Koning M (2015) The distribution of crowding costs in public transport: new evidence from Paris. Transport Res Part A Policy Pract 77:182–201. https://doi.org/10.1016/j.tra.2015.04.005
Hensher DA, Rose JM, Collins AT (2011) Identifying commuter preferences for existing modes and a proposed Metro in Sydney, Australia with special reference to crowding. Public Transport 3(2):109–147. https://doi.org/10.1007/s12469-010-0035-4
Hensher DA, Rose JM, Leong W et al. (2013) Choosing public transport—incorporating richer behavioural elements in modal choice models. Transport Rev 33(1):92–106. https://doi.org/10.1080/01441647.2012.760671
Hörcher D, Graham DJ, Anderson RJ (2017) Crowding cost estimation with large scale smart card and vehicle location data. Transport Res Part B Methodol 95:105–125. https://doi.org/10.1016/j.trb.2016.10.015
Kim KM, Hong SP, Ko SJ, Kim D (2015) Does crowding affect the path choice of metro passengers? Transport Res Part A Policy Pract 77:292–304. https://doi.org/10.1016/j.tra.2015.04.023
Kroes E, Kouwenhoven M, Debrincat L, Pauget N (2014) Value of crowding on public transport in île-de-France, France. Transport Res Rec J Transport Res Board 2417(1):37–45. https://doi.org/10.3141/2417-05
Li Z, Hensher DA (2011) Crowding and public transport: a review of willingness to pay evidence and its relevance in project appraisal. Transport Policy 18(6):880–887. https://doi.org/10.1016/j.tranpol.2011.06.003
Li H, Gao K, Tu H et al. (2016) Perception of mode-specific travel time reliability and crowding in multimodal trips. Transport Res Rec J Transport Res Board 2566(1):22–30. https://doi.org/10.3141/2566-03
Li H, Gao K, Tu H (2017) Variations in mode-specific valuations of travel time reliability and in-vehicle crowding: implications for demand estimation. Transport Res Part A Policy Pract 103:250–263. https://doi.org/10.1016/j.tra.2017.06.009
Liu J, Wen H (2016) Public transport crowding valuation: evidence from college students in Guangzhou. J Public Transport 19(3):78–97. https://doi.org/10.5038/2375-0901.19.3.5
Lu H, Fowkes T, Wardman M (2008) Amending the incentive for strategic bias in stated preference studies. Transport Res Rec J Transport Res Board 2049(1):128–135. https://doi.org/10.3141/2049-15
Márquez L, A JVA, Poveda JC, (2019) In-vehicle crowding: integrating tangible attributes, attitudes, and perceptions in a choice context between BRT and metro. Transport Res Part A Policy Pract 130:452–465. https://doi.org/10.1016/j.tra.2019.09.061
Moher D, Liberati A, Tetzlaff J et al. (2009) Preferred reporting items for systematic reviews and meta-analyses: the PRISMA statement. PLoS Med 6(7):e1000097
Prud’homme R, Koning M, Lenormand L et al. (2012) Public transport congestion costs: the case of the Paris subway. Transport Policy 21:101–109. https://doi.org/10.1016/j.tranpol.2011.11.002
Qin F (2014) Investigating the in-vehicle crowding cost functions for public transit modes. Math Probl Eng 2014:1–13. https://doi.org/10.1155/2014/502708
Sadeghi S, Daziano R, Yoon SY, Anderson AK (2022) Crowding and perceived travel time in public transit: virtual reality compared with stated choice surveys. Transport Res Rec J Transport Res Board 2677(5):296–307. https://doi.org/10.1177/03611981221130346
Sahu PK, Sharma G, Guharoy A (2018) Commuter travel cost estimation at different levels of crowding in a suburban rail system: a case study of Mumbai. Public Transport 10(3):379–398. https://doi.org/10.1007/s12469-018-0190-6
Shao M, Xie C, Li T, Sun L (2021) Influence of in-vehicle crowding on passenger travel time value: insights from bus transit in Shanghai. Int J Transport Sci Technol, China. https://doi.org/10.1016/j.ijtst.2021.09.001
Book Google Scholar
Shelat S, Cats O, van Cranenburgh S (2022) Traveller behaviour in public transport in the early stages of the COVID-19 pandemic in the Netherlands. Transport Res Part A Policy Pract 159:357–371. https://doi.org/10.1016/j.tra.2022.03.027
Shelat S, van de Wiel T, Molin E et al. (2022) Analysing the impact of COVID-19 risk perceptions on route choice behaviour in train networks. PLOS ONE 17(3):e0264805. https://doi.org/10.1371/journal.pone.0264805
Shin H, Kim DK, Kho SY, Cho SH (2020) Valuation of metro crowding considering heterogeneity of route choice behaviors. Transport Res Rec J Transport Res Board 2675(2):162–173. https://doi.org/10.1177/0361198120948862
Tang Y, Jiang Y, Yang H, Nielsen OA (2020) Modeling and optimizing a fare incentive strategy to manage queuing and crowding in mass transit systems. Transport Res Part B Methodol 138:247–267. https://doi.org/10.1016/j.trb.2020.05.006
Tirachini A, Hensher DA, Rose JM (2013) Crowding in public transport systems: effects on users, operation and implications for the estimation of demand. Transport Res Part A Policy Pract 53:36–52. https://doi.org/10.1016/j.tra.2013.06.005
Tirachini A, Hensher DA, Rose JM (2014) Multimodal pricing and optimal design of urban public transport: the interplay between traffic congestion and bus crowding. Transport Res Part B Methodol 61:33–54. https://doi.org/10.1016/j.trb.2014.01.003
Tirachini A, Sun L, Erath A, Chakirov A (2016) Valuation of sitting and standing in metro trains using revealed preferences. Transport Policy 47:94–104. https://doi.org/10.1016/j.tranpol.2015.12.004
Tirachini A, Hurtubia R, Dekker T, Daziano RA (2017) Estimation of crowding discomfort in public transport: results from Santiago de Chile. Transport Res Part A Policy Pract 103:311–326. https://doi.org/10.1016/j.tra.2017.06.008
Wardman M, Murphy P (2015) Passengers’ valuations of train seating layout, position and occupancy. Transport Res Part A Policy Pract 74:222–238. https://doi.org/10.1016/j.tra.2015.01.007
Wardman M, Whelan G (2011) Twenty years of rail crowding valuation studies: evidence and lessons from British experience. Transport Rev 31(3):379–398. https://doi.org/10.1080/01441647.2010.519127
Yap M, Cats O (2021) Taking the path less travelled: Valuation of denied boarding in crowded public transport systems. Transport Res Part A Policy Pract 147:1–13. https://doi.org/10.1016/j.tra.2021.02.007
Yap M, Cats O, van Arem B (2018) Crowding valuation in urban tram and bus transportation based on smart card data. Transportmetrica A Transport Sci 16(1):23–42. https://doi.org/10.1080/23249935.2018.1537319
Download references
Author information
Authors and affiliations.
Department of Civil Engineering, Indian Institute of Technology Roorkee, Roorkee, Haridwar, 247667, India
Rupam Fedujwar & Amit Agarwal
You can also search for this author in PubMed Google Scholar
Corresponding author
Correspondence to Amit Agarwal .
Additional information
Publisher's note.
Springer Nature remains neutral with regard to jurisdictional claims in published maps and institutional affiliations.
List of screened studies
Models MNL—multi-nomial logit, ML—mixed logit, LC—latent class, EC—error component
Data SP—stated preference, RP—revealed preference
Passive data AVL—automated vehicle location, APC—automatic passenger count
Crowding representation (CR) L—Linguistic, 2DD—2D diagram, P—pictorial
Crowding description LF—load factor, SD—standing density, SO—sitting occupancy
Crowding valuation TM—time multiplier, MVpH—monetary value per hour, MVpH—monetary value per trip, WTM—wait time multiplier
NA—not available
Rights and permissions
Springer Nature or its licensor (e.g. a society or other partner) holds exclusive rights to this article under a publishing agreement with the author(s) or other rightsholder(s); author self-archiving of the accepted manuscript version of this article is solely governed by the terms of such publishing agreement and applicable law.
Reprints and permissions
About this article
Fedujwar, R., Agarwal, A. A systematic review on crowding valuation in public transport. Public Transp (2024). https://doi.org/10.1007/s12469-024-00363-w
Download citation
Accepted : 06 March 2024
Published : 02 May 2024
DOI : https://doi.org/10.1007/s12469-024-00363-w
Share this article
Anyone you share the following link with will be able to read this content:
Sorry, a shareable link is not currently available for this article.
Provided by the Springer Nature SharedIt content-sharing initiative
- Crowding valuation
- Public transport
- Mode choice
- Crowding measurement
- Find a journal
- Publish with us
- Track your research
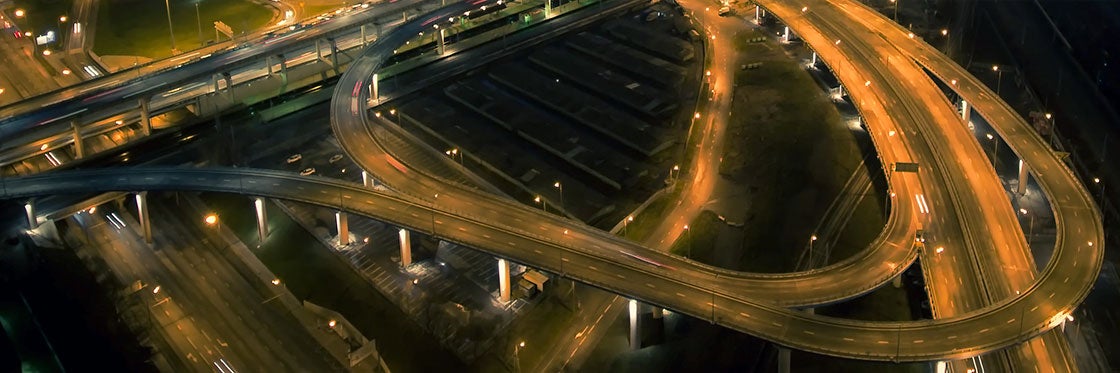
Getting around Moscow
Moscow's public transport network is efficient, comfortable and economical . Discover the city's main modes of transport, their timetables and prices.
Moscow Metro
Moscow tourist bus, tickets & travelcards, you may also be interested in.
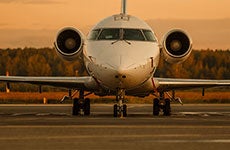
Vnukovo International Airport (VKO)
18 miles south of Moscow is the city's oldest operating airport, Vnukovo International Airport (VKO). Discover how to get to Moscow after landing!
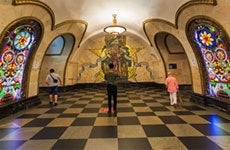
Opened in 1935 under Stalin, the Moscow Metro is one of the largest networks in the world and a tourist attraction in itself. Discover how to get around on it!
Access to Emergency Services: A New York City Case Study
- Chung, Sukhwan
- Smith, Madison
- Jin, Andrew
- Hogewood, Luke
- Kitsak, Maksim
- Cegan, Jeffrey
- Linkov, Igor
Emergency services play a crucial role in safeguarding human life and property within society. In this paper, we propose a network-based methodology for calculating transportation access between emergency services and the broader community. Using New York City as a case study, this study identifies 'emergency service deserts' based on the National Fire Protection Association (NFPA) guidelines, where accessibility to Fire, Emergency Medical Services, Police, and Hospitals are compromised. The results show that while 95% of NYC residents are well-served by emergency services, the residents of Staten Island are disproportionately underserved. By quantifying the relationship between first responder travel time, Emergency Services Sector (ESS) site density, and population density, we discovered a negative power law relationship between travel time and ESS site density. This relationship can be used directly by policymakers to determine which parts of a community would benefit the most from providing new ESS locations. Furthermore, this methodology can be used to quantify the resilience of emergency service infrastructure by observing changes in accessibility in communities facing threats.
- Physics - Physics and Society

IMAGES
VIDEO
COMMENTS
Our public transport model consolidates the timetables from thousands of different providers and agencies to produce an overall model of public transport for a country. At last count we had over 3m public transport stops and stations worldwide. We include all combinations of public transport including train, bus, coach, metro, tram, and ferry.
Travel time and travel time ratio vs. travel distance and population density. Travel time ... To calculate travel time by car, the road network ... P. Perceptions of public transport travel time ...
In a network of density R, we expect that a denser intersection spacing increases travel times due to more waiting compared to a network of the same density R with a larger intersection spacing 5 ...
Therefore, some studies have introduced travel characteristics such as passenger flow, travel time, and travel distance into single-layer networks to quantify the connection strength as the weights of edges, which improves the quality of the network model [14], [17], [18]. However, multilayer public transport network models involve multiple ...
This paper uses a travel time threshold of 30 min that is consistent with many estimates of one-way travel time budgets to work, to measure access to jobs in all cities 35,36,37,38,39.
One argument for containing urban densities is that cities need a critical population density to sustain sufficiently available public transportation. However, the question of whether denser cities foster shorter public transport networks empirically is problematic because real-world transport nets are a product of many additional factors presumably not related to urban form.
We propose probabilistic models for travel time density prediction. ... Modelling probability distributions of public transport travel time components; Bunte S. et al. An overview on vehicle scheduling models. Public Transp. ... Stochastic Trip Planning in High Dimensional Public Transit Network. 2023, arXiv. View all citing articles on Scopus ...
1. Introduction. In order to increase ridership and attract new users, public transport agencies put increasing emphasis on improving the quality of the service they provide and particularly its regularity, also referred to as reliability (Ma et al., 2014).Studies show that a majority of passengers put more value on a reduction of the travel time (TT) variability than on a reduction of TT ...
Travel time variability (TTV) must be considered in evaluations of the effect of traffic control on transport network performance. The objective of this paper was to investigate the relationship between a travel time variability diagram (TTVD), which relates average travel time to its standard deviation, and a macroscopic fundamental diagram (MFD), which relates average network flow to its ...
It takes into account metrics that usually involve transport cost, in-vehicle travel time ... time variability on accessibility to jobs in a large public transport network using smart card big data. J. Trans. Geogr. ... Barthelemy, S.I., Fernández, M.G.: Crowd density estimation for public transport vehicles. In: Proceedings of the EDBT/ICDT ...
Robust relationships between travel time variability and network density and flow rate were analytically derived, investigated, and validated with both simulated and real-world trajectory data. ... Taylor M. Travel Time Variability: The Case of Two Public Modes. Transportation Science, Vol. 16, No. 4, 1982, pp. 507-521. Crossref. Google ...
The paper then analyzes the day-to-day distribution of public transport travel time by using transit signal priority data. ... The probability density function of lognormal distribution is finally used for calculating probability indicators of PTTV. ... "User equilibrium traffic network assignment with stochastic travel times and late arrival ...
1. Introduction. To plan an efficient and attractive public transportation system, it is necessary to know the determinants affecting it (Wei Et Al., Citation 2021).An equitable transit system can cater to the needs of captive riders and maximize transit service coverage (Welch & Mishra, Citation 2013).Access coverage is essential in public transit planning, as this is how service is provided ...
The temporal concentration of travel demand in an urban transport network Carmen Cabrera-Arnau1 , 2∗, Ng Liang Wei , Howard Wong 3, Chen Zhong October 5, 2023 1 Department of Geography and Planning, University of Liverpool, London, UK 2 Centre for Advanced Spatial Analysis, University College London, London, UK 3 Transport for London, London, UK ∗Corresponding author: c.cabrera-arnau ...
We propose a strategy for estimating the public transport system occupancy using open data. Specifically, we use the origin-destination matrix, the population density, and routes' data to determine the traveler's density in the public transportation...
A city's transportation network is its cardiovascular system—a multifaceted structure that enables the continuous flow of people and goods through its arteries. Municipal authorities, city councils, urban planners, and transport-infrastructure owners and operators around the world are well aware that its quality and efficiency are crucial for the economy and for the well-being of citizens.
Various public transport (PT) agencies publish their route and timetable information with the General Transit Feed Specification (GTFS) as the standard open format. Timetable data are commonly ...
With the population density increasing over time [1], the gap be-tween the demand and the supply in public transport system is becoming larger [37]. Enhancing the transit network with new transit routes can reduce this gap [52, 68], hence having the po-tential to bring people from private transport to public transport [20, 41, 61, 62].
Crowding in public transport is a major externality, becoming an increasingly noteworthy influence on users' modal and route choice along with the more traditionally recognized factors of travel time and cost (1, 2).Passenger crowding can lead to transit-users' negative affect and a feeling of stress and discomfort (4 -8).Negativity of crowding is even more pronounced in the age of the ...
Street network area per car worldwide 2019, by city [Graph], Moscow Transport (Department for Transport and Road Infrastructure Development of the Moscow City Government), January 1, 2020. [Online].
Increases in total capacity of public transport, million people per day Share of people using public trans - port1,1 % Average travel time on public trans - port,2 minutes 1 In average total trips on working days. 2 From residential districts near the Moscow Ring Road to the city centre during morning rush hour. 2017 37.6 27.9 2010 2020 +35% 32 ...
Scaling intercity variation in transit rider density to intercity differences in transit accessibility (population weighted city average) produced a scaling coefficient of 1.343 ( R2 = 0.91 ...
In public transport, crowding is one of the variables that is likely to influence the decisions of the choice makers. Crowding has become a subject of concern in metropolitan areas, triggering passenger travel behavior, such as shifting from public to private modes of transport, changing routes or departure times, etc. Hence, there is a need to understand the effect of crowding in public ...
Moscow's public transport network is efficient, comfortable and economical. Discover the city's main modes of transport, their timetables and prices. Civitatis Moscow. ... While Moscow has an efficient public transport system, you may sometimes find it more convenient to travel by taxi. Find fares and top tips here! Read more.
Emergency services play a crucial role in safeguarding human life and property within society. In this paper, we propose a network-based methodology for calculating transportation access between emergency services and the broader community. Using New York City as a case study, this study identifies 'emergency service deserts' based on the National Fire Protection Association (NFPA) guidelines ...